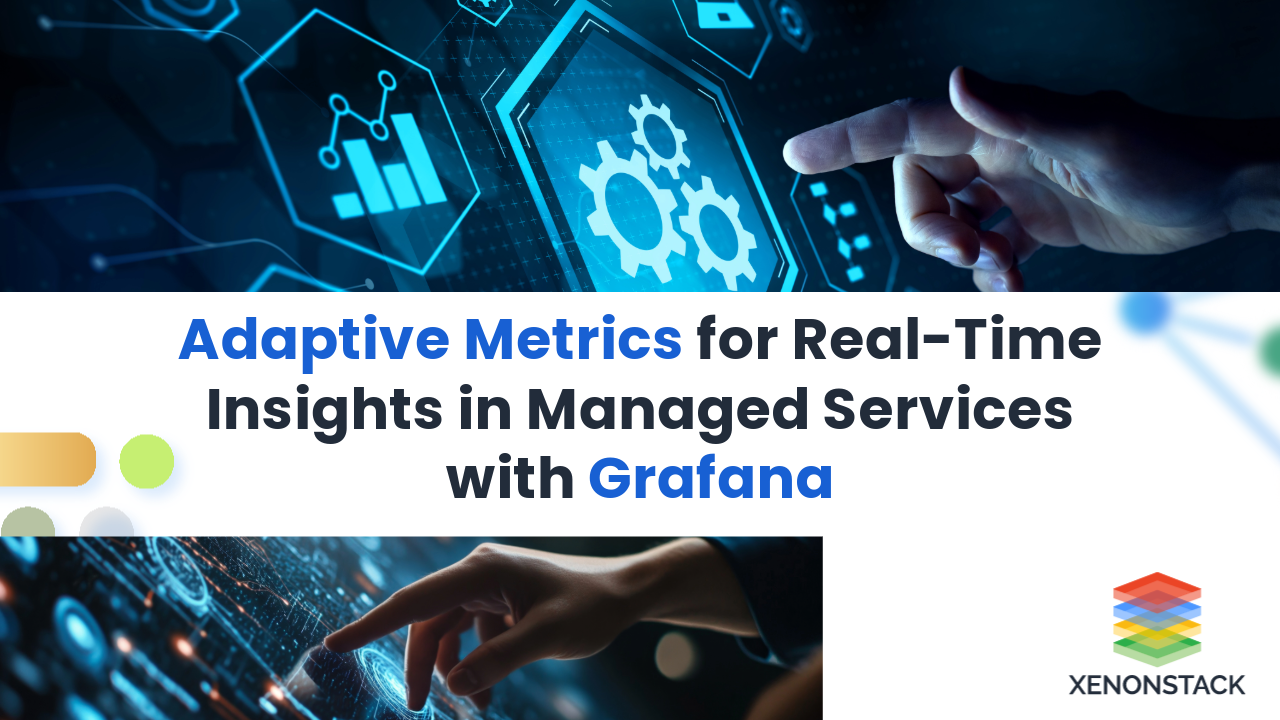
Grafana as a Trusted Solution for Adaptive Metrics
Grafana is an open-source tool for monitoring, analyzing, and alerting real-time data. Prometheus is widely used and integrated into various Parameters like InfluxDB, Elasticsearch, AWS cloud watch, etc., so it is an ideal choice for adaptive Metrics.
Using Grafana, users can create their own dashboards to monitor KPIs in real-time. Its adaptive alerting system may be perfect for dealing with the rather intricate and dynamic character of modern systems’ performance.
Why Grafana is an ideal solution for the adaptive metrics
-
Custom Dashboards: This application, Grafana, enables the creation of visualizations that can be easily modified in response to system operation variations.
-
Adaptive Alerts: The platform can dynamically set alerting limits and avoid reaching limits that might cause false positives.
-
Data Integration: Grafana is capable of receiving data from many data sources simultaneously and may compile a complete picture of the system.
-
Custom Queries: By that, users may use certain languages such as PromQL to query data in real-time and set various tailored automatic change operations.
Adaptive Metrics in Grafana is made up of the following parts
-
Data Sources: Grafana works together with Prometheus and InfluxDB, for example as both pull and push sources, for collecting bucket metrics from your infrastructure.
-
Dashboards: These assist users in making visual images of the performance of the system and adaptive metrics.
-
Panels: Every panel on the Grafana dashboard displays one or several metrics that can be changed live depending on the data.
-
Alerting System: Another feature of Grafana is adaptive alerts, whereby users can define rules for alerts based on changes in the system’s alerting behavior.
Grafana and Prometheus as a Strong Partnership for Adaptive Metrics
Grafana complements Prometheus, an open-source systems monitoring and alerting toolkit, very well. Prometheus is a system for collecting and visualizing time-series data with dynamic rules, whereas Grafana is a data visualization tool and flexible alerting tool.
Both combined provide a robust solution for managing and monitoring such metrics in real-time, reducing the hassles involved in handling changes to system performance.
Benefits of Using Grafana and Prometheus for Adaptive Metrics
-
Real-Time Monitoring: Both tools deal with dynamic metrics and their alarms depending on the conditions in the system.
-
Custom Alerts: Thanks to Prometheus’s query language, PromQL, and Grafana’s alerting engine, an alert is generated only when something is really changing in a meaningful range.
-
Multi-Source Integration: They both are cloud, multi-clouds, and on-premise compatible tools.
Proactively measuring and monitoring goals in managed services as adaptation metrics
Adaptive metrics are essential in multifaceted environments such as managed services. They assist in identifying poor performance, delays, and outages, which is paramount when it comes to meeting SLAs and maintaining service availability.
Appropriate Roles of Adaptive Metrics in Managed Service Projects
-
Auto-Scaling Microservices: It is worth mentioning that microservices have adaptive metrics that can auto-scale based on the actual load. This way, the utilization of CPU and memory in real-time allows for the allocation of the resources appropriately.
-
Real-Time Anomaly Detection: Adaptive metrics capture thresholds for each tenant in a multi-tenant environment, so performance issues can be easily identified and resolved without necessarily impacting the entire landscape.
-
Resource Optimisation and Cost Control: It assists MSPs to more effectively monitor resources usage and optimize them to cut on running expenses.
-
Compliance Monitoring: For clients with strict SLA regulations, adaptive metrics allow MSPs to define service performance indicators and notice when service delivery exceeds the SLA.
Setting Up Adaptive Metrics in Grafana for Managed Services
Step 1: Data Source Configuration
When working with managed service projects of the adaptive metrics kind in Grafana, the first thing to do is enter data sources such as Prometheus or InfluxDB. These data sources furnish actual-time information, and they can constantly show data for monitoring system performance.
Step 2: The interconnected systems are to make dynamic dashboards
Once you’ve configured the data sources, it is possible to make Grafana dashboards that would be adequate for a certain managed service environment. For instance, you can create dashboards to oversee diverse clients or aspects of your infrastructure. These dashboards shall be dynamic, and this means that in instances where there is a change made in the performance data, the changes will reflect automatically hence providing you with relevant performance data.
Step 3: Self-organising - Configure Alert Mechanisms
Now, create dynamic alert rules in Grafana based on the Prometheus query language—or PromQL. For instance, you can set conditions that form alarms, such as high CPU usage over the normal range for a given time. The whole purpose of using adaptive thresholds is to prevent you and your team from being disrupted by a lot of false positives and let you deal with what actually matters.
Step 4: Real-time monitoring and optimization of the algorithms
Once the Grafana dashboards are completed, one can monitor the metrics in real-time. Leverage the data to optimize the system's settings, increase the number of resources if required, and address any problem before it impacts your clients’ SLAs (Service Level Agreements). Such an approach proves effective as it allows you to prevent problems before they occur.
Benefits of Adaptive Metrics in Managed Service Projects
-
Proactive Issue Resolution: Adaptive metrics enable service providers to identify issues that are likely to arise in the future and that will affect the client. This improves uptime and helps to meet the set SLAs more easily.
-
Customized Monitoring: This means that some of your clients or a certain part of your infrastructure may require different parameters for monitoring and alerting. Adaptive metrics enable you to define different parameters for each client, which also makes it possible to define the rules more freely regarding systems.
-
Cost-Effective: Adaptive metrics, on the other hand, allow the dynamic tracking and allocation of resources within the system, meaning that unnecessary resource usage is eliminated. This amounts to a reduction in wastage and efficient use of structures, hence reducing costs.
Challenges and Solutions
-
Handling Complexity: Prometheus’ data is vital and raw, which, of course, may present some intricacies when creating accurate PromQL queries in Grafana. An example of how this may be made easier is to incorporate standards templates or community-proposed dashboard templates first.
-
Avoiding Alert Fatigue: Although adaptive metrics are designed to filter out false positive alarms, if the thresholds are set incorrectly, the opposite result can occur. This way, only strong alerts get delivered, and reviewing and changing the alert settings after a while can provide valuable insight and better fine-tune the alert settings.
Conclusion
Adaptive metrics are the future of monitoring, this kind of statistics is very important in keeping modern systems running the way they should. Using these metrics with Grafana only enhances them as they turn into more efficient real-time data visualization, flexible alerting, and the opportunity to work with several data sources at a time.
In a managed service environment, adaptive metrics can help in monitoring ahead of time, conforming to the SLAs, and utilizing the resources optimally. With infrastructures changing and evolving into complex systems, there are enabling products like Grafana that can assist organizations in preventing concerns about performance that may affect their clients’ engagement.
As the need for performance data in real-time grows, so does the interest in adaptive metrics, adjusting the metrics for the current state and working with Grafana in particular, suitable for both MSPs and large enterprise clients.
Explore more about Top Real Time Analytics Use Cases Read about Real Time Analytics Architecture