What is Agentic AI?
Consequently, it is a form of Artificial Intelligence that can be described as a highly developed approach that attempts to replicate human decision-making as closely as possible regarding goal setting, plan generation, and modifications depending on new data or feedback availability.
Agentic AI, or Autonomous AI, refers to artificial intelligence systems that exhibit autonomy and proactivity. Unlike traditional AI, which primarily follows pre-defined rules or scripts, agentic AI can:
- Make decisions independently: I can do this based on my understanding of the environment and its goals.
- Take initiative: Proactively seek out opportunities or address challenges.
- Learn and adapt: Improve its performance over time through experience.
- Exhibit goal-oriented behavior: Pursue specific objectives or outcomes.
Autonomous AI is cognitive and can reason, learn from its environment, and adapt. It can also deconstruct problems into subproblems and self-organize precisely what should be done to achieve certain goals. Some of the principles on which these techniques are based include autonomy, adaptability, and feedback.
History of Agentic AI
Agentic structures mark a major development in generation, expanding on earlier ideas of generative systems. Unlike conventional systems, which reply to activations, agentic structures autonomously set desires, reason about them, and plan moves to achieve them.
-
Early Foundations (1950s-60s): In the 1950s and 1960s, self-maintaining structures developed with rule-based frameworks. These systems were designed to tackle problem-fixing obligations using predefined algorithms specializing in primary choice-making and logical operations.
-
Development and Formalization (1980s-90s): In the 1980s and 1990s, the Agent-Based Model (ABM) emerged as an effective approach for simulating and reading complicated structures, especially in economics and social sciences. ABM allowed researchers to version interactions and emergent behaviors within diverse structures.
-
Expansion and Integration (2000s-10s): During the 2000s and 2010s, mixing gadget-gaining knowledge with agent-primarily based systems enabled those sellers to learn from and adapt to large volumes of records. This integration enhances sellers' efficiency and overall performance via advanced algorithms and huge data analytics.
-
Modern Era (2020s-Present): In the 2020s, agent-based total structures have improved significantly, with studies that specialize in improving agent autonomy and interplay skills and additionally address moral issues associated with transparency and societal effect.
Key Takeaways
-
Agentic AI replicates human decision-making through goal setting, planning, and adaptability based on feedback.
-
It improves customer service, with 54% of companies using agentic AI for faster, personalized responses, boosting satisfaction.
- The global AI market is projected to reach $594 billion by 2032, driven by the widespread adoption of Agentic AI across various industries.
-
Key features include autonomy, adaptability, and real-time learning for decision-making.
-
Agentic AI systems can dynamically set goals and adjust strategies using real-time data, enhancing effectiveness in complex scenarios.
-
Its architecture focuses on modularity, scalability, and interoperability for seamless integration with existing technologies.
-
Applications include healthcare, finance, and autonomous vehicles, demonstrating its versatility.
-
Challenges include ensuring transparency, building trust in autonomy, and addressing ethical decision-making implications.
72% of companies are now deploying Composite AI solutions as per According to McKinsey. By 2026, Gartner Predicts over 80% of enterprises will use AI Agents and Agentic Workflow for Data Management and Fabrics
Agentic AI Architecture
Although it is expected that Agentic AI marketers will perform as many activities as possible, such as planning and scenario expectation, situation retention for historical leverage, and using hardware or apps such as APIs, their systems' runtime ability creates risks of running code, as there are risks associated with system runtime that need to be managed in the overall architecture.
Additives of Agentic AI Architecture
Perception
Perception accommodates a synthesis of sensory information toward expert surroundings. Perception additionally contains the outlook of accumulating statistics from numerous assets, processing fusion of noise, or even suppressed data.
Key Technology Components:
-
Multi-modal Fusion: Universal Modal Fusion is the simultaneous use of many specific devices, including cameras, microphones, and sensors, in a scene to increase situational focus, enhance precision, and integrate various bodily complex intelligence inside one composite system.
-
Noise Robustness: Noise Robustness ensures that the agent can still work satisfactorily under noise or under conditions of wrong and missing statistics. These issues use measures that reduce these issues use measures which reduce noise, preserve a given stage of performance, and withstand specific noise tiers.
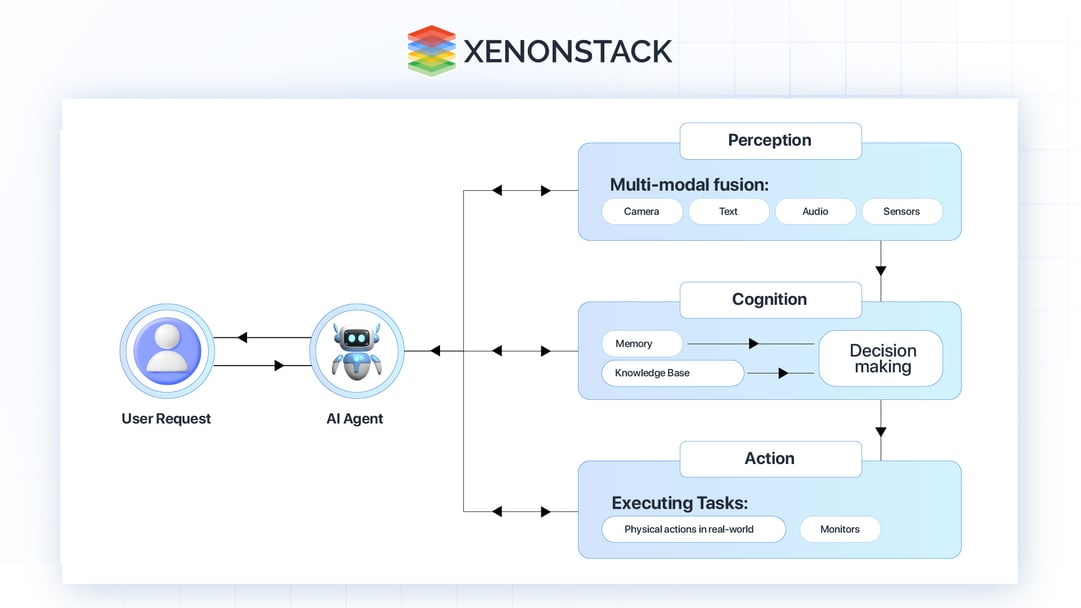
Cognition
Cognition describes acquiring and processing information, making conclusions, and learning from what is studied. It includes reading files, assessing selections, changing strategies primarily based on results, and enhancing one's capability to make powerful decisions based on enjoyment and comments.
The key technology in this location includes:
-
Deep Learning Models: These models rely upon and train neural networks in complicated duties, including judgment, choice-making, and pattern recognition of massive volumes of records with a hierarchy of capabilities and complex dating between inputs and outputs. Deep Learning Applications and Challenges
-
Reinforcement Learning focuses on gaining knowledge of the high-quality movements to take. It uses rewards and punishments to refine techniques through experimentation to optimize benefits over dissimilar periods in diverse situations.
-
Probabilistic Reasoning: This approach manages uncertainty by applying probabilities to measure feasible consequences and making the best choices under limited or ambiguous information, thereby improving predictions and selection-making.
-
Meta-mastering ensures that the character can quickly adapt to exceptional duties by enabling them to apply techniques and studies acquired in past situations. Thus, examining and carrying out duties with little extra statistics or education is feasible.
Action
Concept of carrying out responsibilities and making decisions; rendering the action into control algorithms for a specific task, employing robots and actuators for interaction with the environment, and using feedback to adjust and improve performance.
Essential parts include:
-
Control Algorithms: Performing various tasks using mathematical models and computer-based techniques with optimal effectiveness and efficiency instigated by mental actions and environmental parameters.
-
Robotics and Actuation: Incorporate onboard electromechanical systems, such as motors and sensors, to achieve active physical motion in the real world. This allows devices and robots to interact effectively with environments.
-
Feedback Loops: Feedback mechanisms assist in altering movements or actions by identifying changes made from the intended movements. Sensors make it possible to change and enhance the effectiveness of a given task over time.
Key Principles Guiding the Architecture of Agentic AI Systems
-
Modularity: Subdividing competencies into different modules of concepts, each responding to exact duties such as notion or movement. This approach makes it easier to develop, protect, and enhance, as well as flexibility, robustness, and integration.
-
Scalability: AI agents' capacity to extend computational belongings and abilities to address developing statistics and complexity, the usage of dispensed computing, cloud infrastructures, and parallel processing to keep common overall performance.
-
Interoperability: Provides standard formats of protocol interfaces to ensure smooth integration of high-quality modules and structures. This principle enables the summation of several technologies, 1/3-birthday party services, and legacy systems into one AI agent.
-
Adaptability: Allows AI Agents to examine new testimonies and alter them to convert environments. It uses online knowledge acquisition, switch analyzing, and dynamic updates to stay powerful and relevant.
Framework of Agentic AI System
-
Agent Architecture: Defines the internal structure, including decision-making, memory, and interaction capabilities.
-
Environment Interfaces: Connect agents to their operating environments, whether simulated or real.
-
Task Management: Manages task definition, assignment, and completion tracking.
-
Communication Protocols: Facilitates interaction among agents and between agents and humans.
-
Learning Mechanisms: Machine learning algorithms are employed for performance improvement over time.
-
Integration Tools: Connect agents with external data sources, APIs, and other software.
-
Monitoring and Debugging: Allows developers to observe behavior, track performance, and identify issues.
How Agentic AI Systems Make Decisions
Unlike fixed goals and plans, the objectives of Agentic AI are established dynamically, and its routines involve modeling the consequences of actions based on data from various information sources and taking appropriate action.
It starts when a need arises and subsequently passes through several stages. The AI agent detects the problem by gathering necessary data and then develops a plan using programmed or personal learning techniques. The created strategies are adjusted to the new environment in unexpected environmental changes.
Example in E-commerce: An AI system intended to increase traffic on certain websites might initially be effective in drawing traffic. Yet, if business stakeholders note that high traffic does not lead to sales, for example, AI would understand that a change of tactic is desirable. It might then focus on optimizing the check-out process to capture more values. This approach transcends mere shallow actions; it empowers AI systems to haggle or mediate and interact independently without human intercession.
Adapting to New Information: Adaptability is the core of Agentic AI. These systems continuously refine their decisions based on real-time information, ensuring alignment with evolving objectives and environmental changes.
Concepts Associated with Tailoring Agentic AI
Understanding and implementing tailored Agentic AI involves several key concepts:
-
Personalization vs. Customization:
-
Personalization involves adjusting the AI agent's interactions based on an individual's preferences and behaviors.
-
Customization optimizes the AI agent's structure and performance to address specific business or industry challenges.
-
Autonomy and Decision-Making:
-
Autonomy pertains to the degree of independence an AI agent possesses and the frequency with which it must make decisions on behalf of users.
-
Decision-making refers to the processes by which AI agents evaluate options and make choices based on their programming and learning capabilities.
-
Data Privacy and Security:
-
Data Privacy ensures that user data used for personalization and modifications is well-protected and complies with established privacy standards.
-
Security Measures involve implementing safeguards within the AI system to protect its data and prevent unauthorized access.
Key Methods of Adaptation in Agentic AI System
Best Practices for Deploying Agentic AI in an Enterprise
Developing Agentic AI agents means following a set procedure to tailor them to particular functions.
Here’s a step-by-step overview:
Define Objectives and Requirements
-
Identify Needs: Determine the particular business or person’s requirement that the AI agents will fulfill. This includes consulting, involving other stakeholders, and setting goals and objectives.
-
Set Goals: Some of the best practices that should follow customization include Setting quantifiable targets and standards to measure the effectiveness of the customization.
Data Collection and Analysis
-
Gather Data: Gather data that will be used to train the AI agent and create a personalized model for the user. This data can be user, business process, or specific information typical for an industry.
-
Analyze Data: Research to gather information about client behaviors and needs that must be followed when developing customized products.
Create and Educate the ML Model
-
Design Model: Select the most suitable AI models and algorithms for the set goals and Cookies source.
-
Training: Use the data collected to train the AI agent and change parameters and/or algorithms to achieve the desired results.
Implement Customization
-
Integrate with Systems: The AI agent must be used in a workflow or system integration that it has been designed to fit. This may mean API connections, software changes, or system settings.
-
Test and Validate: Undertake comprehensive exercises to test this AI agent and establish its performance against set objectives.
Monitor and Optimize
-
Performance monitoring entails continuously evaluating the level to which an AI agent meets its needs to measure its efficiency.
-
Optimization: Optimize it iteratively, using feedback and performance to improve the agent's accuracy.
Agentic AI Applications
The choice is also worthy of recognition for self-sustaining dealers who select and communicate with their surroundings. Its relevance is as broad as historical perspective, social context, and a domain of multiple disciplines and further advancement in those fields.
Problem Advancement and Process Optimization
-
Examples of complicated structures: Asset-based AI particularly allows for evaluating rising dispositions and resulting garb with the beneficial resource of mapping gender illustration, which incorporates financial markets or excursion spot traveler styles and permits mastering the definition of a complex device with many interacting additives.
-
Optimization: Agents correct optimization issues based on suggestions by reviewing several feasible choices and optimizing for remarks. This technique is especially useful in logistics, networking, and payload distribution, improving productivity and overall performance.
Controls and Robots
-
Autonomous automobiles: Agentic AI is essential for developing sufficiently unbiased motors, in aggregate with motors, drones, and robots, permitting them to navigate, make actual-time alternatives, and interact with objects. A dynamic environment interacts with distinct entrepreneurs.
-
Robotics: AI, based entirely on object-oriented robotics, lets robots autonomously carry out complex obligations, adapt to changing environments, and interact efficiently with human beings and specialized robots.
Communication structures and Digital Dealers
-
Virtual Assistants: Powered through Agentic AI, virtual assistants like Siri, Alexa, and Google Assistants interpret human requests, select gadgets, and ship customized responses to foster interplay and customize patron interactions.
-
Games and Entertainment: AI, based completely on life-like marketers, are customizable NPCs in video games and simulations, allowing them to change their simple conduct based totally on the movements of the individuals and accompanied by using other video games of participation.
Social and Economic Simulations
-
Economic Modeling: Agent-based total fashions simulate monetary structures to forecast market behaviors, reading man or woman stores with desires and constraints to assess how coverage modifications or out-of-door conditions affect the monetary tool.
-
Social Systems: Agentic AI is used to look at social dynamics, which incorporates behavior unfolding and desire-making strategies and the outcomes of social networks, with programs in public health, sociology, and policymaking.
Research and Development
-
Advancing AI Theory: Research in agentic AI enhances the theoretical records of sensible structures, specializing in designing entrepreneurs who exhibit complicated behaviors and interact efficiently with their environments.
-
Innovative Applications: Ongoing advancements in Agentic AI have addressed issues of proactive development in various fields, leading to advanced functionality of self-directed systems, which are otherwise becoming a norm in people’s lives.
Agentic AI System Use Cases
Agentic AI has diverse and impactful use instances throughout numerous fields.
-
Robots: Robots carry out complicated responsibilities and engage with people.
-
Virtual Assistants: Personal assistants like Siri and Alexa offer personalized answers.
-
Games: Responsible NPCs who control player moves to gain participation.
-
Healthcare: Licensing AI for prognosis, treatment-making plans, and private care.
-
Financial: Identify the place of job practices and fraud by reading financial statements.
-
Smart Cities: Optimization of communications shipping, power, and public safety in city garages.
-
Economic concerns: Modeling marketplace quarter behavior and coverage consequences for the venture.
-
Customer carrier: AI chatbots meet questions and useful duties through adaptive learning.
-
Supply Chain Management: To decorate inventory management and logistics through predictive analytics.
-
Autonomous vehicles: Autonomous cars and drones press and pick in actual time.
Building Agentic AI Systems for Redefining Enterprise Applications
-
Autonomy: Operates independently, reducing the desire for human intervention.
-
Adaptability: Adjusts to new statistics and converts situations efficiently.
-
Scalability: Handles growing data and complexity by increasing assets.
-
Complex Problem Solving: Tackles hard problems with superior algorithms and simulations.
-
Real-Time Decision Making: Makes immediate choices based mostly on non-stop feedback.
-
Enhanced Interaction: Provides customized and interactive reports via adaptive getting-to-know.
-
Robust Performance: Maintains reliability in noisy or incomplete statistical situations.
-
Integration and Flexibility: Seamlessly integrates diverse technologies and tools.
-
Improved Efficiency: Automates obligations, streamlines approaches, and decreases manual striving.
-
Predictive Capabilities: Forecasts future outcomes through analyzing information and styles.
Difference Between Agentic AI vs. Traditional AI
Aspect
|
Agentic AI
|
Traditional AI
|
Decision-Making
|
Makes independent decisions based on actual-time records and goal behavior.
|
It typically follows constant policies and default algorithms.
|
Adaptability
|
Adapts to new information and changing environment.
|
Works in rigid frameworks with limited adaptability.
|
Discuss
|
It interacts dynamically with the environment and different elements.
|
Communication is normally extra habitual and much less energetic.
|
Learning
|
Continue to examine and enhance with actual-time comments.
|
Often, gaining knowledge is based totally on historical statistics without real-time optimization.
|
Scalability of Performance
|
Scales are used to increase computing sources and improve algorithms.
|
Scalability is restrained via predefined rules and stuck algorithms.
|
Easy adjustments
|
It presents a high stage of pliability to carry out exclusive duties and meet different necessities.
|
Change is averted by using predefined regulations and described constraints.
|
Dealing with Complexity
|
Handles complex, dynamic conditions with superior algorithms.
|
It handles easy and well-defined conditions with little complexity.
|
Real-Time Feedback
|
Provides immediate responses to environmental modifications and interactions.
|
Feedback is usually delayed or rigid to real-time adjustments.
|
Difference Between Agentic AI vs Generative AI
Aspect
|
Generative AI
|
Agentic AI
|
Purpose
|
Designed to create new content material or records based totally on discovered patterns.
|
Focuses on self-sustaining decision-making and goal fulfillment.
|
Learning
|
Learns from large datasets to produce novel outputs.
|
Uses actual-time feedback for non-stop development and version.
|
Applications
|
Applied in content material introduction, language models, and innovative responsibilities.
|
Used in robotics, independent automobiles, and complex simulations.
|
Complexity Handling
|
Manages complexity in data era and sample recognition.
|
Handles complicated, dynamic eventualities through adaptive algorithms.
|
Flexibility
|
Adapts content generation based on educational information and algorithms.
|
Adapts behavior and strategies primarily based on actual-time inputs and dreams.
|
Integration
|
Integrates into content-centered applications and innovative gear.
|
Integrates into structures requiring decision-making, interplay, and flexibility.
|
Interactivity
|
Limited interactivity is targeted at generating outputs in preference to interactions.
|
Engages dynamically with environments and different dealers.
|
Scalability
|
Scales by growing information size and model complexity.
|
Scales through increasing computational sources and adapting algorithms.
|
Challenges in Agentic AI Systems
However, several factors hinder Agentic AI, though it has a lot of potential as follows:
-
Transparency and Explainability: As these systems become relatively more autonomous, being clear on decision-making processes has become relevant. How an agent arrives at a certain decision should only be known to the users and the developers. To some extent, a new area called Explainable AI has emerged to make such AI decisions understandable to humans.
-
Trust and Reliability: Self-contained means that the characteristics of how a founded system will perform are difficult to predict in specific circumstances. And that creates trust issues, especially where you have risk contexts, such as health or finance.
It is difficult to achieve this, as indicated by the following. From the higher-order models to the actual APIs and complicated polymorphic memory arrangements, many technologies contribute to the formation of AI in a very agentic realm. This makes development and deployment challenging, particularly for resource-scarce organizations or agencies.
-
Ethical Implications: The ethical concern is much more massive when dealing with AI’s self-made decisions. This raises a concern about what will happen if the AI makes a different decision that is contrary to the interests of humans, even with a bias or by having consequences that are not well predicted. The commitment to ethical policies and governance concerning these systems will thus become necessary for properly utilizing this technology.
Agentic AI Platform For ITOps and ServiceOps
Applying Agentic AI to IT Service and Operations management data, tooling, and processes enables ServiceOps ( AIOps, observability, and automation). Unify Workflows and Data use cases involving descriptive, predictive, prescriptive, and cognitive phases, leveraging real-time telemetry data with a unified platform, accelerating productivity, insights, and automation.
Workflow Data Fabrics bring convergence across data stores, integrations, APIs, dashboards, services, and more with Agentic AI and AI Agents. Real-time visibility, incident prevention, change risk management, and cross-team collaboration require the Democratization of Data for Contextual intelligence and Decision Intelligence. High-cardinality data management and high-dimensional data allow detailed examination without performance bottlenecks. Telemetry data and generating the desired context become very challenging.
Leveraging Agentic AI Workflows and autonomous agents to Drive Progressive Delivery with continuous deployments with visual Prompting and conversational prompts would automate DevSecOps and ITOps processes by creating workflows.
Data Fabric And Telemetry Workflows For Agentic AI
Progressive Delivery, DevSecOps, ITOps, IT planning, and SRE teams use autonomous agents to query the Agentic AI system to execute telemetry or observability pipelines for analytics. For example:
- Descriptive: Understanding the target environment, assets, and their interdependencies.
- Predictive: Simulate what-if scenarios using digital twins for complex IT environments.
- Prescriptive: Run complex tasks and closed-loop automation to resolve commonly encountered incidents, add and remove devices into maintenance modes, or run Camunda or Ansible scripts.
- Cognitive: Composable tasks to run autonomous services on agentic AI systems.
These telemetry pipelines are responsible for data integration, ingestion, aggregation and transformation, and data enrichment and routing, which create the knowledge graph used by GraphRAG.
Conversational queries can now be used with Agentic Workflows for
- AI Insights for Productivity and Efficiency: Create dashboards and KPIs, update CMDBs, and create data center or application modernization plans.
- Data Analysis and Incident Management: Context data, correlations, resolve tickets, and Summarization of tickets and Analysis.
- Automation as Code: Execute configuration, provisioning, and Integration services or intelligent diagnostic actions.
Agentic AI Systems Make Autonomous Enterprises
Agentic AI, also called autonomous AI, drives enterprises towards autonomous operations with advancements in Machine learning, NLP, large language models, LVM, and multimodal AI for unbiased formation and modification of complex, dynamic environments using optimized algorithms, modular designs, and scalable architectures. Major Applications in autonomous cars, conversational platforms, Voice AI Agents, complex simulations, and DNA Analysis structures power innovation and new breakthroughs. Composite AI and Casual AI drive adoption as Hybrid AI with Different functions, enhancing performance and capabilities.