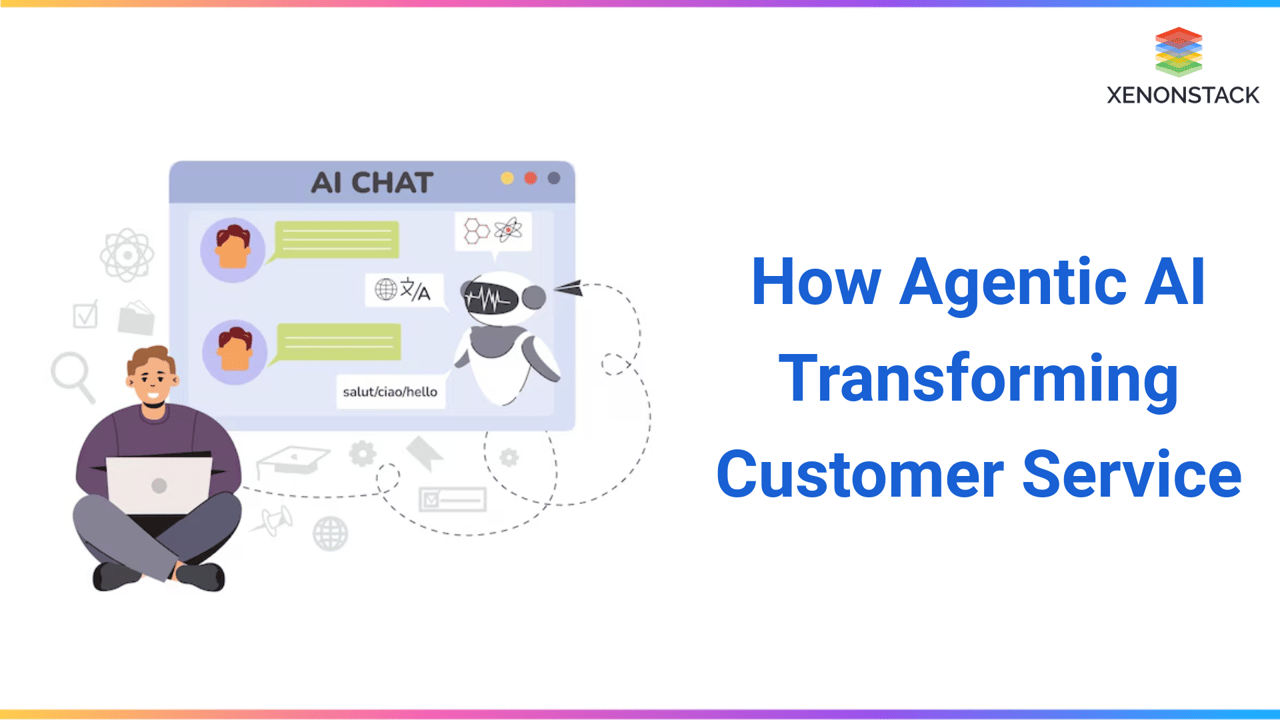
In today's competitive business landscape, delivering exceptional customer service is no longer optional—it's a strategic imperative. With customers expecting immediate, personalized responses 24/7 and businesses seeking to optimize costs, AI agents have emerged as a transformative solution. These sophisticated digital assistants redefine the customer service paradigm, enabling enterprises to deliver superior experiences while reducing operational costs.
What Are AI Agents for Customer Service?
AI agents are intelligent, autonomous digital workers who operate within business processes to complete tasks, make decisions, and adapt to changing conditions without human intervention. Unlike traditional chatbots that follow rigid scripts, AI agents leverage advanced natural language processing, machine learning, and cognitive automation to understand context, detect intent, and interact dynamically with customers.
Industry research explains, AI agents in customer service transform the traditional support model by offering personalized, round-the-clock assistance to customers. By analyzing vast amounts of data in real-time, these agents can swiftly resolve inquiries, provide accurate information, and even anticipate customer needs before they arise.
While chatbots are rule-based and limited to predefined scripts, AI agents can:
-
Autonomously execute tasks such as processing refunds, updating records, or scheduling appointments
-
Learn and adapt from past interactions to continuously improve accuracy and effectiveness
-
Integrate seamlessly with multiple business systems, including CRMs, help desks, and analytics platforms
-
Understand and respond contextually using NLP and sentiment analysis, ensuring more human-like interactions
The Business Case for AI Agents
The compelling business case for AI agents becomes evident when examining the current customer service landscape:
- According to LeewayHertz, 65% of customers switch companies due to poor experiences, resulting in $3.7 trillion in global sales losses for 2024
- Automation Anywhere reports that autonomous customer support has proven to slash resolution times by close to 90%
- NVIDIA highlights that 41% of organizations use AI-powered copilots for customer service, with 60% implementing them for IT help desks
- Beam AI states that organizations can reduce operational costs by up to 70% by automating routine processes with AI agents.
How AI Agents Work in Customer Service
AI agents transform customer service by automating interactions, personalizing support, and providing instant resolutions. Here’s a step-by-step look at how these intelligent systems operate within modern customer service environments:
-
Customer Interaction Initiation: AI agents begin their work when a customer reaches out via chat, email, voice, or social messaging platforms. These interactions are captured immediately, allowing the agent to start processing the query without human involvement.
-
Natural Language Understanding (NLU): Using advanced natural language processing (NLP), the AI interprets the customer's language, identifies the intent behind the message, and extracts key data points like names, dates, and order numbers. It may also analyze sentiment to adjust the tone of its replies.
-
Intent Mapping & Decision Making: Once the customer’s intent is clear, the AI agent maps it to the appropriate action. This could range from pulling up account details and issuing a refund to redirecting the query to a human agent if needed.
-
Real-Time Data Retrieval: AI agents connect with backend systems like CRMs, order management software, and support databases to fetch real-time information. This enables immediate answers to inquiries such as “Where’s my order?” or “What’s my last invoice?”
-
Response Generation: Based on the data retrieved and the conversation context, the AI composes a reply using language models or templated responses. These messages are personalized, accurate, and human-like in tone, ensuring smooth interactions.
-
Knowledge Base & FAQ Use: AI agents leverage existing knowledge bases and FAQs to resolve common questions. They can also automatically generate or suggest new FAQ content based on recurring customer queries, reducing ticket volume over time.
-
Escalation to Human Agents: If the AI cannot resolve the issue or senses frustration, it hands off the interaction to a human agent. All relevant context — chat history, sentiment, and user profile — is shared with the human for a seamless transition.
-
Learning & Feedback Loop: Every interaction provides data for improvement. AI agents use customer feedback, agent corrections, and performance analytics to continuously learn and refine their responses, becoming more effective over time.
Types of AI Agents in Customer Service
Customer service operations can benefit from various specialized AI agents:
Fig 1: Types of AI Agents in Customer Service
-
Virtual Customer Assistants (VCAs): These advanced AI agents handle complex customer service tasks by integrating multiple capabilities, including chat, voice, sentiment analysis, and predictive analytics, to provide comprehensive support.
-
Automated Follow-Up Agents: These agents ensure that customer concerns are adequately addressed by following up on unresolved issues, sending reminders, collecting feedback, and providing additional assistance.
-
Fraud Detection Agents: They monitor customer interactions and transactions for signs of fraudulent activity, analyzing patterns and behaviors to identify potential fraud and taking preventive actions.
-
Intelligent Routing Systems: These systems analyze and direct customer issues to the appropriate department or support channel, ensuring accurate and timely resolutions.
-
Sentiment Analysis Agents: They analyze the emotional tone of customer interactions, detect frustration levels, and help prioritize certain concerns for quicker resolution.
-
Predictive Analysis Agents: These agents use historical customer data, browsing history, and purchase patterns to forecast future needs and tailor customer service accordingly.
-
Knowledge Management Agents gather and store information such as product details, troubleshooting guides, and FAQs, leveraging machine learning and NLP to keep the knowledge base up-to-date and accessible.
![]()
Key Capabilities of AI Agents for Customer Service
Modern AI agents offer a comprehensive set of capabilities that transform customer service operations:
Perceive and Respond to Dynamic Changes: AI agents can detect and interpret shifts in customer behaviour and preferences, track changes in buying patterns, identify trends in inquiries, and recognise sentiment shifts in feedback.Reasoning and Interpretation: Using advanced algorithms, AI agents analyze extensive datasets, extract meaningful patterns, and derive actionable insights, enabling them to understand complex customer queries and predict potential issues.
Problem-Solving Skills: AI agents excel at resolving various customer service challenges, from troubleshooting technical issues to resolving billing disputes and providing product information. Continuous Learning and Adaptation: Through ongoing interactions with customer data, AI agents engage in inference and learning processes, analyzing past interactions, identifying patterns, and anticipating customer needs.Natural Conversations: Advanced NLP capabilities allow AI agents to engage customers in natural, free-flowing conversations, fostering meaningful dialogue and building customer rapport across various communication channels.
Use Cases of AI Agents in Customer Service
Category |
Use Case |
Description |
AI Agent Capabilities |
Inquiry & Request Handling |
Customer Inquiry Triage |
Automatically understands, classifies, and routes queries from chat/email. |
NLU, intent recognition, real-time routing. |
|
Order Status Updates |
Shares personalized, real-time order info with customers. |
System integration, FAQ-based responses, proactive updates. |
|
Intent Recognition |
Detects query intent to deliver accurate resolutions. |
Pattern analysis, contextual awareness, multi-turn dialogue. |
|
FAQ Automation |
Builds dynamic FAQ responses from helpdesk data. |
Automated KB/FAQ generation, self-learning from conversations. |
Ticket Management |
Auto Ticket Creation |
Creates support tickets with key extracted details. |
GenAI-based templates, entity extraction. |
|
Categorization & Prioritization |
Sorts and prioritizes tickets based on urgency and content. |
NLP, sentiment analysis, urgency scoring. |
|
Smart Routing |
Directs tickets to the right team instantly. |
Classification, skill-based routing logic. |
|
Customer Acknowledgment |
Sends auto-confirmation messages post-ticket creation. |
Personalized auto-responses with customer data merge. |
Resolution & Closure |
Suggested Solutions |
Recommends fixes, articles, or workflow steps. |
Real-time KB search, contextual solution suggestions. |
|
AI-Generated Responses |
Drafts human-like, accurate replies. |
LLM-powered response generation and language tuning. |
|
Resolution Progress Updates |
Share status updates with customers and agents. |
Real-time workflow integration and ticket state monitoring. |
|
Follow-ups & Closure Notices |
Sends post-resolution follow-ups and closure confirmations. |
Automated message sequencing and satisfaction check-ins. |
Post-Issue Engagement |
Customer Satisfaction Survey |
Measures post-resolution satisfaction. |
Smart survey personalization, feedback categorization. |
|
Feedback Request |
Solicits open-ended feedback from customers. |
Automated messaging and sentiment tagging. |
|
Testimonial Collection |
Gathers positive reviews from satisfied users. |
Trigger-based outreach and testimonial templates. |
|
Knowledge Gap Analysis |
Identifies missing or unclear support info. |
Analytics on repeat queries, auto-suggestion for KB updates. |
Customer Interaction |
Conversational Support |
Delivers human-like interactions across channels. |
LLMs, multi-modal interfaces, contextual memory. |
|
Contextual Engagement |
Adapts replies based on previous conversations. |
Persistent session data, real-time memory recall. |
|
Chat Transcript Delivery |
Automatically provides detailed transcripts. |
Auto-logging, PDF/email generation. |
Sentiment & Feedback |
Feedback Analysis |
Summarizes insights from open-ended responses. |
NLP clustering, key phrase extraction. |
|
Sentiment Detection |
Adjusts tone or priority based on user sentiment. |
Emotion detection, tone shifting. |
|
Proactive Service Adjustments |
Detects trends and updates services or policies. |
Social listening, feedback loop integration. |
Operational Analytics |
Customer Churn Prediction |
Identifies potential at-risk customers. |
Behavior pattern mining, churn scoring. |
|
Service Demand Forecasting |
Optimizes resource allocation based on trends. |
Predictive modelling, ticket volume heat mapping. |
Real-Time Features |
Knowledge Base Access |
Instantly retrieves relevant support docs. |
AI search, intent-matching retrieval. |
|
Live FAQ Handling |
Real-time auto-reply to common questions. |
FAQ mapping to intents, dynamic updates via user feedback. |
Benefits of AI Agents in Customer Service
Implementing AI agents in customer service operations delivers numerous benefits:
Operational Efficiency
-
24/7 Availability: AI agents provide round-the-clock support, ensuring customers can access assistance anytime and anywhere.
-
Automating Routine Tasks: They handle mundane tasks like answering emails, managing tickets, and processing simple requests, improving efficiency and consistency.
-
Handling Large Volumes of Data: AI agents quickly analyze vast amounts of data to identify patterns and root causes of issues, enabling timely and relevant resolutions.
-
Reducing Average Handling Times (AHT): Advanced AI agents can "complete tasks in under a minute, streamlining workflows and enhancing speed across operations."
Enhanced Customer Experience
-
Consistent Support: AI agents ensure uniform response across different platforms and departments.
-
Multilingual Support: They provide seamless support in multiple languages without additional costs.
-
Personalized Interactions: By processing real-time data and referencing past assistance, AI agents deliver customised experiences that make customers feel valued.
-
Eliminating Repetitive Explanations: With instant access to past interactions, AI agents provide immediate and informed responses without requiring customers to repeat themselves.
Cost Efficiency
-
Reducing Operational Costs: AI agents can save up to 70% on operational costs by eliminating the need for ongoing training and handling mundane tasks.
-
Easy Scalability: AI agents can be scaled instantly with minimal adjustments, handling sudden increases in call volumes without extensive hiring and training.
-
Easy Data Availability: They provide instant access to crucial customer data, helping human agents understand the context of issues and respond more effectively.
Building AI Agents for Customer Service
Developing AI agents that effectively handle customer service tasks involves more than just plugging in a chatbot. It requires a thoughtful combination of business strategy, technical architecture, and continuous learning. Here’s a comprehensive breakdown of the key stages involved:
Fig 2: Building AI Agents for Customer Service
1. Define the Use Cases
Before writing a single line of code, identify the problems the AI agent intends to solve.
-
Target High-Impact Areas: Start with use cases that are repetitive, rule-based, and time-consuming for human agents. Examples include order tracking, password resets, refund requests, appointment bookings, and basic troubleshooting.
-
Align With KPIs: Ensure the agent is being built to support measurable goals such as reducing average response time, increasing first-contact resolution (FCR), or lowering customer service costs.
-
Avoid Overreach Early On: It's best to begin with a narrow scope, prove the AI agent’s value, and expand to more complex tasks.
2. Choose the Right AI Platform or Framework
Your development platform choice significantly affects how scalable, intelligent, and integratable the AI agent will be.
-
Cloud-Based Platforms:
-
Google Dialogflow: Strong in natural language understanding (NLU), easy integration with Google products.
-
Microsoft Bot Framework: Robust SDK with Azure support, great for enterprise integrations.
-
IBM Watson Assistant: Known for reliability and enterprise-grade deployments.
-
-
Open Source & Customizable:
-
Rasa: Ideal for full control over data, workflows, and on-premise deployments.
-
OpenAI GPT APIs: Perfect for building conversational, generative experiences using large language models.
-
-
No-Code Platforms:
-
Tars, Landbot, or Chatfuel: Great for quick prototyping and non-technical teams.
-
3. Design Conversational Workflows
Conversation design is at the heart of a successful AI agent.
-
Map Intents and Entities: An intent is what the user wants to do (e.g., “Track my order”), while entities are the specific details (e.g., order number, product name).
-
Create Dialog Flows: Use flowcharts or conversational design tools to build logical paths, including greetings, main tasks, fallback responses, and handover triggers to human agents.
-
Design for Error Recovery: Users will make mistakes, so ensure the bot can gracefully handle typos, vague questions, and misunderstood inputs.
-
Add Personality: A consistent tone aligned with your brand enhances user trust and engagement.
4. Train with Domain-Specific Data
AI agents must understand your customers, products, and business context — this only comes with good data.
-
Use Real Conversations: Feed the agent with historical support tickets, chat transcripts, email queries, and FAQ documents.
-
Label Training Data: For supervised learning, tag examples with the correct intent and expected outputs to improve accuracy.
-
Iterate Frequently: Your AI model should continuously retrain and adapt as customer behaviour and language evolve.
5. Integrate with Backend Systems
An AI agent is only as good as the systems it can interact with.
-
CRM Integration: Access customer profiles, past interactions, and preferences in real-time (e.g., Salesforce, HubSpot, Zoho).
-
Ticketing Systems: Automate ticket creation, categorization, and escalation (e.g., Zendesk, Freshdesk).
-
Order Management: Let the AI retrieve order statuses, initiate returns, or modify delivery preferences.
-
APIs & Webhooks: Ensure smooth, secure data exchange between the AI agent and business systems.
This enables AI agents to talk to users and take meaningful action on their behalf.
6. Enable Multichannel Deployment
Modern customer service spans multiple channels — and so should your AI agent.
-
Channels to Cover:
-
Website chat widgets
-
Mobile apps
-
WhatsApp, Facebook Messenger
-
Email and SMS
-
Voice assistants or IVR systems
-
-
Unified Experience: Use an orchestration layer to maintain context across channels so users don’t have to repeat themselves.
-
Omnichannel Analytics: Track user behaviour and satisfaction across all touchpoints for a holistic view of customer experience.
7. Monitor, Analyze & Optimize
Once your AI agent is live, it is crucial to treat it as a learning system rather than a finished product.
-
Performance Metrics:
-
Ticket deflection rate
-
Customer satisfaction score (CSAT)
-
Net promoter score (NPS)
-
First response time and resolution time
-
Escalation rates
-
-
User Feedback Loops: Let users rate bot responses and flag errors — use this data to fine-tune accuracy.
-
Regular Audits: Schedule monthly or quarterly conversation logs reviews to spot improvement areas.
8. Ensure Governance, Ethics, and Compliance
As AI agents gain autonomy, trust and transparency become essential.
-
Data Privacy: Ensure compliance with regulations like GDPR, CCPA, and HIPAA — anonymize data where needed.
-
Security Measures: Implement authentication, encryption, and secure data handling practices.
-
Bot Transparency: Always disclose when users interact with an AI agent and offer easy opt-outs to speak with humans.
-
Bias & Fairness Checks: Regularly test for biases in training data or decision-making patterns to ensure fair treatment of all users.
Challenges and Considerations in Customer Service
While AI agents offer significant benefits, organizations should be aware of potential challenges:
-
Data Privacy and Security: Ensuring the secure handling of customer data is crucial, requiring strict compliance with data protection regulations such as GDPR and CCPA.
-
Implementation Complexity: Deploying AI agents requires careful planning, investment, and system integration, with a phased approach recommended for successful adoption.
-
Complex Query Handling: AI agents may struggle with highly nuanced customer issues that require human empathy, contextual understanding, or advanced decision-making.
-
Continuous Training Requirements: These systems need regular updates with fresh data and insights to maintain accuracy, relevance, and efficiency in dynamic customer service environments.
Real-World Applications of AI Agents in Customer Service
Organizations across industries are already leveraging AI agents to transform their customer service operations:
ServiceNow: ServiceNow has introduced IT and customer service management AI agents that "can understand context, create step-by-step resolutions and get live agent approvals when needed."
The Ottawa Hospital: NVIDIA reports that the hospital uses AI agents to "improve patient care and reduce pre-procedure anxiety" by providing consistent, accurate, and continuous access to information.
City of Amarillo, Texas: The city uses a multilingual digital assistant named Emma to provide its residents with 24/7 support, improving "the disbursement of important information to all residents, including the one-quarter who don't speak English."
Why AI agents are the Future of Customer Service
AI agents rapidly emerge as a cornerstone of modern customer service strategies because they deliver consistent, high-quality support at scale. Unlike human agents, they offer uninterrupted, 24/7 availability, ensuring customers receive timely assistance across all time zones. Their capacity to handle thousands of interactions simultaneously makes them exceptionally scalable, allowing businesses to meet growing support demands without increasing headcount.
AI agents significantly reduce response and resolution times by accessing real-time data and automating routine workflows, enhancing customer satisfaction and operational efficiency. Additionally, they contribute to substantial cost savings by automating Tier-1 queries and repetitive tasks. Most importantly, AI agents provide a seamless omnichannel experience, maintaining consistent interactions across live chat, email, voice, and social media—ensuring a unified and professional brand presence across all customer touchpoints.
Next-Level Agentic AI
Talk to our experts about implementing Agentic AI for customer service. Learn how businesses use Agentic AI to automate support, enhance engagement, and deliver personalized, efficient customer experiences.