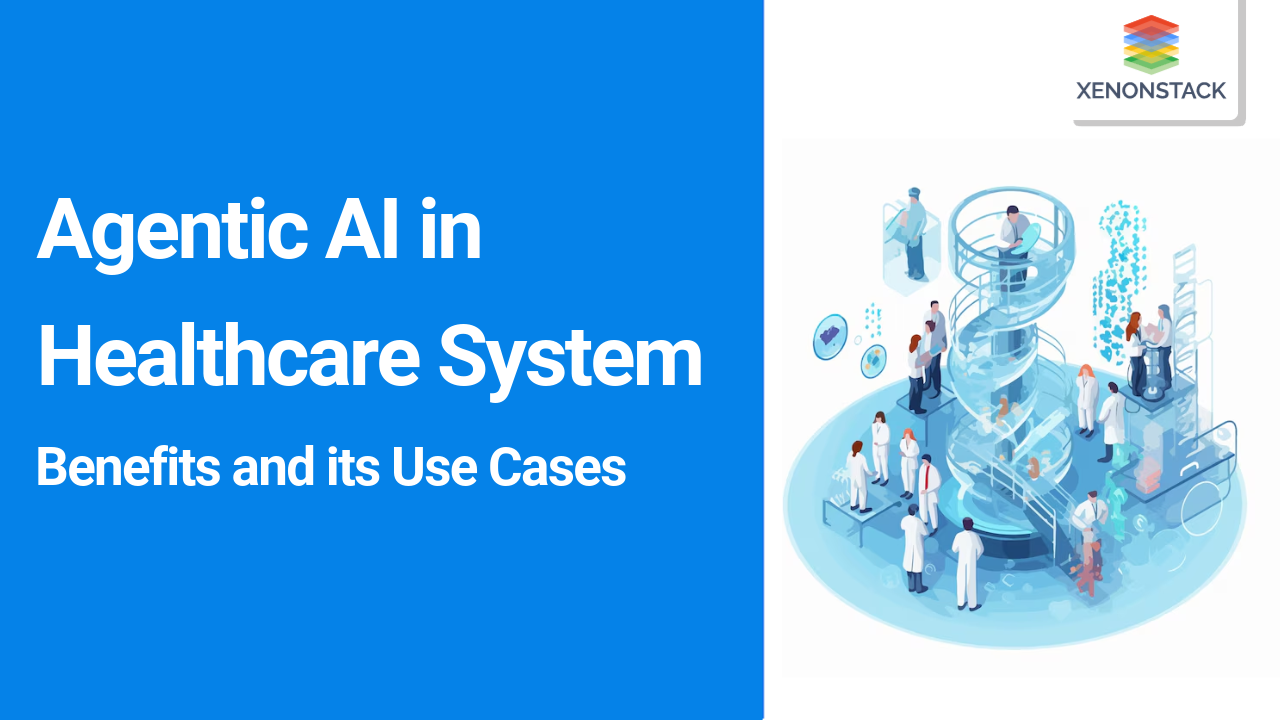
2. Medical Imaging Enhancement
Description
Medical imaging provides critical insights into the human body, supporting early disease detection and treatment. However, processing and analyzing large volumes of imaging data remains challenging.
Challenges & Opportunities
-
Large, complex imaging datasets
-
Data integration issues across Electronic Health Records (EHRs)
-
Security and privacy concerns regarding sensitive patient data
How Can Agentic AI Help?
-
Image Enhancement: Enhances image quality to help doctors detect diseases more accurately.
-
Data Augmentation: Generates additional medical images to improve diagnostic model training and research.
-
Noise Reduction: Eliminates distortions and artefacts from scans, making medical images more explicit.
-
Super-Resolution Imaging: Converts low-quality scans into high-resolution images for better analysis.
-
Automated Segmentation: Highlights and isolates specific areas, such as organs or tumours, for precise examination.
-
Pathology Prediction: Identifies patterns in images to detect diseases at an early stage.
-
Personalized Medicine: Adapts imaging analysis to a patient's unique medical history for tailored treatments.
-
Clinical Decision Support: Assists radiologists by providing insights and recommendations based on imaging data.
3. Drug discovery and development
The drug discovery process is lengthy, expensive, and prone to high failure rates. Traditional methods require years of research and clinical trials to bring new treatments to market.
Challenges & Opportunities
-
High costs and extended timelines
-
Complexity in identifying viable drug candidates
-
Regulatory hurdles and compliance requirements
How Can Agentic AI Help?
-
Target Identification: Helps identify biological targets that could lead to effective new drugs.
-
Compound Generation: Designs and suggests new drug compounds with the right chemical properties.
-
Predicting Drug Interactions: Assesses potential risks when combining different drugs to prevent adverse effects.
-
Lead Optimization: Refines molecular structures to improve drug effectiveness and reduce side effects.
-
Personalized Medicine: Uses patient genetic data to customize drug treatments for better outcomes.
-
Biomarker Discovery: Identifies biological markers that help in diagnosing diseases and selecting targeted therapies.
-
Clinical Trial Optimization: Improves patient selection and trial efficiency to increase success rates.
-
Drug Repurposing: Finds new uses for existing drugs, speeding up treatment availability.
4. Medical Research and Data analysis
Medical research relies on vast amounts of data to improve disease prevention, diagnosis, and treatment.
Challenges & Opportunities
-
Data privacy and security regulations (e.g., HIPAA)
-
Standardization and interoperability issues
-
Complex genomic and proteomic data analysis
How Can Agentic AI Help?
-
Efficient Data Processing: Quickly analyzes large medical datasets to uncover meaningful insights.
-
Natural Language Processing (NLP): Understands and interprets complex medical literature and patient records.
-
Document Summarization: Condenses lengthy medical studies into easy-to-understand summaries.
-
Trend Analysis: Identifies patterns in research data to highlight emerging healthcare trends.
-
Search and Retrieval: Improves search accuracy, making it easier to find relevant medical information.
-
Data Integration: Merges data from various sources to create a unified and comprehensive dataset.
-
Predictive Analytics: Forecasts disease trends and predicts treatment outcomes based on historical data.
5. Risk Prediction of Pandemic Preparedness
Predicting and managing pandemics requires real-time data analysis and early intervention strategies.
Challenges & Opportunities
-
Identifying emerging diseases early
-
Equitable vaccine distribution
-
Global coordination of response efforts
How Can Agentic AI Help?
-
Early Detection: Monitors real-time health data to identify potential outbreaks before they spread.
-
Predictive Analytics: Analyzes trends to anticipate disease hotspots and guide preventive actions.
-
Vaccine Development: Accelerates research by identifying promising vaccine candidates more efficiently.
-
Supply Chain Management: Helps predict and prevent shortages of medical supplies during health crises.
-
Public Communication: Identifies misinformation and ensures accurate health updates reach the public.
-
Resource Allocation: Assists in distributing hospital beds, equipment, and staff based on demand.
-
Scenario Planning: Simulates different outbreak scenarios to improve preparedness and response.
6. Generating Synthetic Medical Data
Synthetic medical data is artificially generated but statistically resembles accurate patient data. It helps train AI models while preserving patient privacy.
Challenges & Opportunities
-
Ensuring realism and diversity in generated data
-
Complying with privacy regulations
-
Balancing synthetic data quality with real-world applications
How Can Agentic AI Help?
-
Privacy Preservation: Produces patient-like data that mimics real cases while protecting actual identities.
-
Customization: Generates specific datasets tailored to different medical research and training needs.
-
Testing and Validation: Provides a safe environment for testing new healthcare technologies and models.
-
Training AI Models: Supplies diverse and extensive datasets to improve the accuracy of healthcare tools.
-
Ethical and Legal Compliance: Ensures that generated data meets privacy regulations and ethical guidelines
7. Personalized medicine
Personalized medicine tailors treatments to individual patients based on their genetic and molecular profiles.
Challenges & Opportunities
-
Complexity in interpreting genetic data
-
Lack of standardized genetic protocols
-
Patient communication and consent issues
How Can Agentic AI Help?
-
Data Analysis: Examines complex genomic data to identify patterns and personalize treatments.
-
Clinical Decision Support: Assists doctors in choosing the best treatment based on patient-specific data.
-
Education and Training: Keeps healthcare professionals informed about the latest developments in genomics.
-
Patient Communication: Simplifies genetic information, making it easier for patients to understand their health.
-
Pharmacogenomic Insights: Predicts how a patient will respond to medications based on their genetic profile.
Generative AI adoption in the Healthcare industry faces several challenges that need careful consideration for successful implementation:
Challenges in Adopting Agentic AI in Healthcare
1. Ensure Data Privacy and Security: Prioritize robust data privacy and security measures. Implementing encryption, access controls, and ensuring compliance with healthcare privacy regulations like HIPAA are crucial steps in safeguarding patient information.
2. Collaboration with Healthcare Professionals: Promote collaboration between AI specialists, data analysts, and medical professionals. Involving clinicians in the development process ensures that AI solutions align with clinical workflows and address real-world healthcare challenges.
3. Ethical Guidelines and Bias Mitigation: Establish ethical guidelines for AI use in healthcare. Mitigate biases in training data and algorithms to ensure fair and unbiased outcomes, especially in sensitive areas like diagnostics and treatment recommendations.
4. Intuitive User-Friendly Interfaces: Design user-friendly interfaces for healthcare professionals to interact with AI systems seamlessly. Integrating AI into existing workflows should enhance efficiency and decision-making without causing disruptions.
5. Continuous Training and Education: Ensure that healthcare professionals receive continuous training and education to effectively comprehend and utilize AI tools. Keep them informed about the most recent advancements, ethical considerations, and optimal approaches in AI applications.
6. Clinical Validation and Regulatory Compliance: AI models should undergo thorough clinical testing and validation to ensure their accuracy and reliability. It is crucial to adhere to regulatory standards and obtain the required approvals before implementing AI solutions in clinical environments.
7. Transparent Decision-Making Processes: Ensure transparency in AI decision-making processes. Healthcare professionals should understand how AI algorithms arrive at conclusions to build trust and facilitate collaboration.
8. Scalability and Integration: Scalability and smooth interaction with current healthcare systems are critical factors to take into account while developing AI solutions. For smooth integration, compatibility with various hospital IT systems and Electronic Health Records (EHR) is essential.
10. Patient Engagement and Informed Consent: Involve patients in discussing AI applications in their healthcare. Ensure transparency and informed consent for AI technologies, respecting patient autonomy and privacy.
Future Trends in Agentic AI for Healthcare
Rapid technological advancements make healthcare more predictive, personalized, and efficient. These innovations will transform patient care, medical research, and operational workflows.
-
Smarter Drug Discovery: New technologies will accelerate the identification of potential drug compounds and predict interactions. This will reduce development time and costs, bringing life-saving treatments to patients faster.
-
Precision in Surgery: Robotic-assisted procedures will enhance surgical accuracy and reduce recovery times. Minimally invasive techniques will lead to better outcomes and lower complications.
-
Tailored Treatments: Advancements in genomics will enable highly personalized treatment plans based on individual biology. This approach will improve effectiveness and minimize side effects for patients.
-
Real-Time Disease Monitoring: Advanced data analysis will track disease outbreaks and predict health trends. Proactive responses will improve public health strategies and pandemic preparedness.
-
Virtual Health Assistants: Digital tools will provide instant medical guidance, symptom tracking, and mental health support. This will improve healthcare accessibility, especially in remote and underserved areas.
These innovations will drive efficiency, improve patient experiences, and create a more proactive healthcare system.