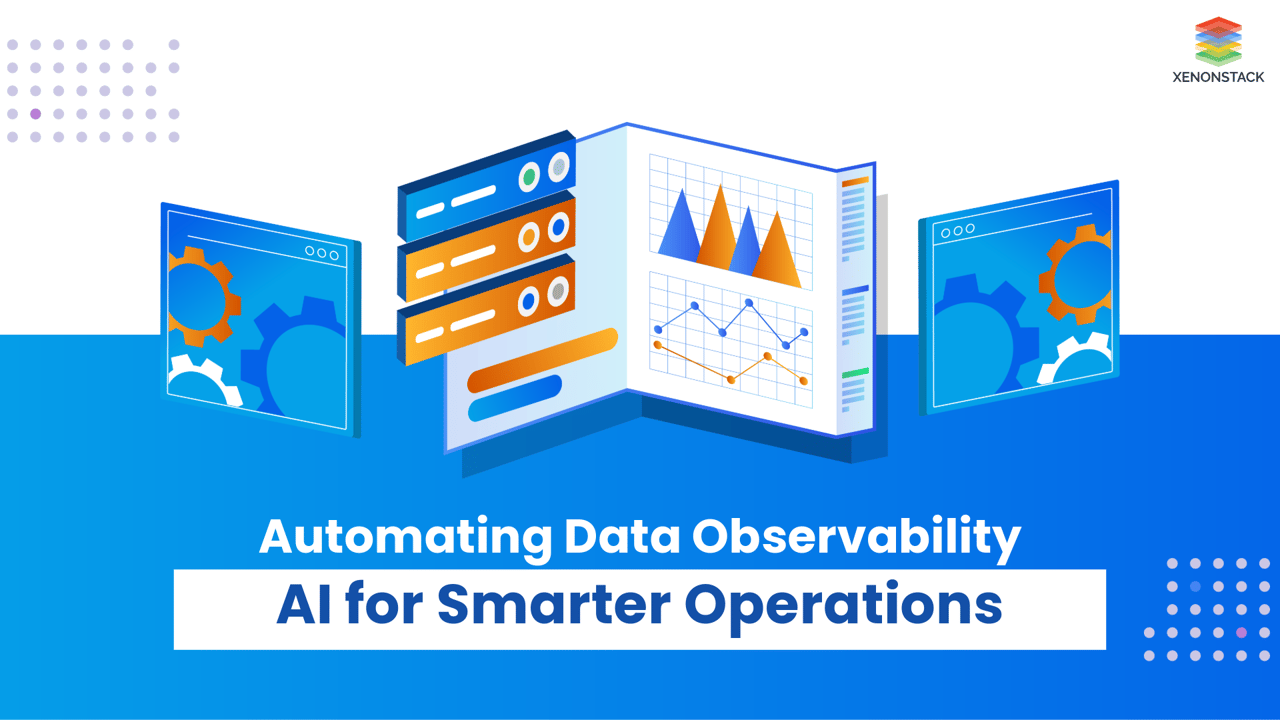
Proactive Downtime Prevention: How AI Transforms System Reliability
AI-driven data observation basically changes how downturn is prevented. Through predictive analysis, automated workflows and continuous monitoring, AI can identify potential problems and solve them before disturbance.
Early Warning Systems
The AI system can act as initial warning systems by detecting the pattern that indicates potential problems, such as data operation, Schema change or network failure. For example, if any data pipeline experiences significant changes in the data coming, AI can flag it as a potential computer driving and notify the teams before the downstream system is the effect.
Fig - AI-Driven Workflow
-
Pattern Recognition in Data Drift and Schema Changes - AI-driven systems can trace and identify patterns in data operations and skima changes. For example, if the format or distribution of data that comes over time changes, the AI system may detect these changes and increase the notice so that the teams can check and solve problems before affecting the data.
Automated Remediation Workflows
When a problem is detected, the AI system can trigger automatic workflows. For example, if a nonconformity is found in a dataset, the system can automatically roll back in the previous version of the data, fix the problem and restore the general operation without manual intervention.
Reducing Mean Time to Detection (MTTD) and Resolution (MTTR)
By detecting deviations and automatic causes the fundamental cause analysis, AI reduces the time it takes to identify and solve. This leads to Mean time to detect (MTTD) and Mean Time to Resolution (MTTR), which ensures rapid recovery from possible operating arrangements.
Case Studies: AI Observability in Action
-
E-commerce: Stop box failure
E-commerce platforms are very dependent on the box system for sale. AI-driven data observation can reveal that when problems occur, such as payment processing or nonconformity in stock data. By quickly identifying problems, AI can ensure that the box goes smoothly to prevent potential loss of income.
-
Fintech: Avoid transaction data leaks
In the fintech industry, the privacy and security of data is important. AI systems can monitor financial transactions in real time and detect unusual patterns that may indicate fraud or data violations. By identifying and addressing these problems, AI transactions help ensure safety and integrity of data data.
Integrating AI-Driven Observability into Existing Systems
AI-operated observation is not a standalone solution, rather the overall system is integrated with existing systems to increase flexibility, such as devops and dataops.
-
Compatibility with DevOps and DataOps practice
The AI-driven observational qualifications can basically be integrated with devops and dataops practices, increase the workflow for software growth, purpose and data operations. With AI monitoring in place, Team can identify bottlenecks, improve the system's reliability and automate many aspects of the development and distribution process.
-
Cloud-outland observation equipment
Many cloud suppliers offer natural observation equipment that can easily be integrated with AI-powered solutions. These devices are often designed to function originally within their respective ecosystem (eg AWS, GCP or Azure), making it easier for companies to use AI observation on a scale.
-
AWS, GCP and Azure ecosystem integration
Cloud platforms such as AWS, Google Cloud and Microsoft Azure offer broad observation service including AI-operated monitoring and deviations. By taking advantage of these Skyland units, organizations can integrate AI-controlled observations into their existing infrastructure without the need to invest in complex, standalone solutions.
-
Build active data culture of governance
To be effective by AI interaction overview, organizations must use an active data management culture. This involves installing clear data quality guidelines, privacy and security and ensuring that AI equipment to identify potential risks and problems is often trained.
Navigating the Limitations: Realistic Expectations for AI Observability
While AI operated data observation provides huge benefits, there are challenges and boundaries to consider.
-
Data Privacy and Ethical AI Concerns
The use of AI in data monitoring increases the concerns of privacy and moral ideas. It is important to ensure that the AI models do not unintentionally violate privacy laws or prejudice that can lead to discriminatory practice.
-
Over-Reliance on AI: Balancing Automation with Human Oversight
While AI can automate many tasks, it is necessary to maintain human inspection to ensure that the automatic decisions match business goals and moral standards. There should be a balance between taking advantage of AI for efficiency and ensuring that the human decision is still an important part of the decision -making process.
-
Model accuracy and false positive/negative
AI systems are not infallible. False positive (incorrect identification of a problem) and false negatively (failed to identify a problem) can still occur, affecting the reliability of the observation system. Continuous monitoring and model training are necessary to reduce these errors.
-
Cost and complexity in the implementation
AI implementation of the driven observation system can be composed and expensive. Organizations must weigh potential benefits against AI infrastructure, training and investments required for integration.
The Future of AI-Driven Observability
The AI future for data observation appears to be promising. As AI technologies develop, observation skills will expand the scope and abilities, which can lead to even greater efficiency in the prevention of downtime.
-
Self -healing data pipeline
One of the most exciting developments is the capacity of self -healing data pipes. When AI constantly monitors and analyses data, the system can automatically correct when problems arise and end the requirement of manual intervention.
-
Edge Computing and IoT overview
As an edge data processing and IoT units, the AI-driven observation will extend beyond centralized cloud infrastructure. Organizations must monitor and manage the huge amounts of data generated on the edge, making AI-producing observation another important component.
-
Advances in Explainable AI (XAI) for Transparency
Explaining AI (XAI) is a growing area that wants to make AI -declining processes more transparent. When XAI technologies improve, organizations will be able to understand that the AI-driven observation system detects and makes decisions, increases confidence and adoption.
-
Industry-Specific Observability Solutions
AI-driven observation solution will be more sewn for specific industries. In the health care system, for example, AI can be used to monitor real -time medical data, while in finance it can help to detect and ensure compliance with rules.
Concluding Insights: Transforming Enterprise Resilience with AI
Recap of AI’s Transformative Impact
AI-controlled data observation is a game switch for companies to prevent shutdown. By taking advantage of machine learning, predictable analysis and automatic workflows, business data quality and system can continuously identify and solve problems, ensuring reliability.
Call to Action: Adopting AI Observability
Organizations should begin using AI-operated observation to remain competitive in today's data-driven world. By integrating AI into its data tube lines and operating work flows, companies can achieve real -time insights, increase operating efficiency and reduce downtime.
Final Thoughts on Sustainable Downtime Prevention
The future of downturn prevention lies in the AI action overview. As technology develops, the possibilities of observation skills are also able to lie in front of potential problems and ensure continuous accessibility of the data. Clamps AI, companies can ensure more flexible, skilled and durable future.