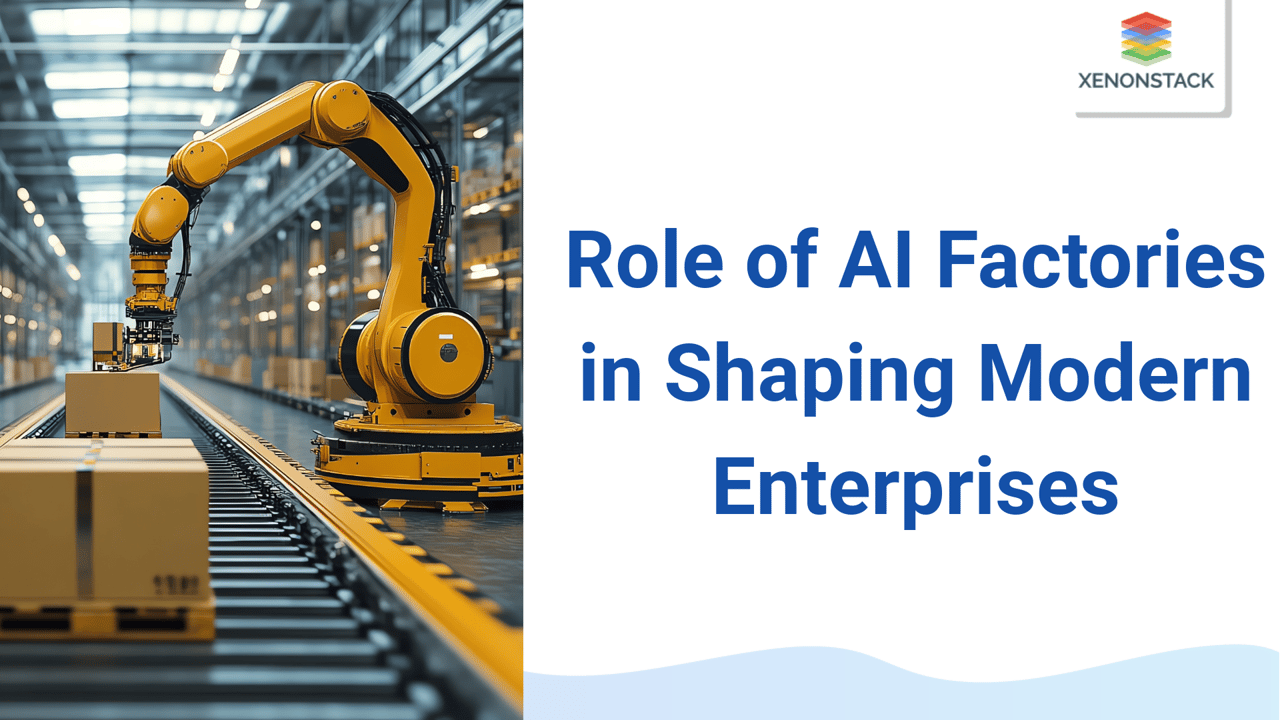
AI has become the new frontier of innovation for industries worldwide. Since industries need factories, AI industries also need an “AI factory” to help implement their AI strategies. An AI factory is not just a convenient term but a functional model that encapsulates computing, storage, and networking to provide real-time insight from seemingly infinite data pools for businesses.
What is an AI Factory?
An AI factory actualizes artificial intelligence by maximizing computer systems, storage, and networking to provide an environment that optimally supports AI tasks. It makes it possible to achieve optimal performance throughout the entire system so that raw data generate insights, predictions, and automation. It forms the basis for businesses to derive better decisions, efficiencies, and new value propositions based on artificial intelligence.
In its simplest terms, an AI factory seeks to silence and reproduce the AI process and bake it into the culture so everyone can use it across the organization. GPUs and high-performance computing structures are some of the factors of AI operations that make running core algorithms more efficient for businesses, eradicating issues like high latency and poor resource utilization.
The Growing Need for AI Factories
Due to the spectacular progress of AI in academic research and its application, AI requirements have grown, and there is a necessity for specific infrastructure. It is very expensive and often technologically unworkable to attempt to build traditional data centres to manage the tremendous amount of computer processing that AI applied demands; often, it is thousands of times more than what is demanded by conventional computing tasks. This has led to the emergence of recently christened AI Factories, which are increasingly embraced in the retail, manufacturing, and health sectors.
Why Do We Need an AI Factory?
AI is no longer a thing of the future because it has been integrated into business functionality. Today, industries apply AI in various ways, such as effective forecasting, streamlining business processes, individual customer treatment, and immediate decision-making. However, for any AI program to work, AI-driven quality control must integrate immense quantities of data and analyze them in real time.
-
Scalability: AI adoption will continue to rise, but businesses require a solution capable of handling this growing, complex nature and volume of data.
-
Performance: AI applications are process-intensive and demanding in processing requirements across various AI applications, including deep learning and natural language processing. AI factories make the best use of GPUs and other resources to offer the best results.
-
Efficiency: Machine shops allow data to flow smoothly, increasing the pace and volume of analytics and action.
-
Automation: Using artificial intelligence to help with data preparation, training, and model deployment, AI factories minimize resource consumption and eliminate human factors.
Key Components of an AI Factory
Every AI factory depends on four main engines, without which the data would be useless and could not be turned into intelligence.
-
Data Pipeline
A data pipeline could, therefore, be defined as the logistics of data management involving Data Acquisition, Data Cleaning, and Data Integration. Data management primarily checks that the data being fed into AI models is of good quality, cleansed, and fit for AI consumption. Data pipelines help to process raw, unstructured data, consolidating it into a form usable by AI algorithms. Data quality takes centre stage in the AI factory's effectiveness since bad data implies poor performance and wrong forecasts. -
Algorithm Development
Algorithms are the pipelines that generate any AI solution. In data analysis, algorithms filter data, identify features and patterns, forecast, and provide decision-making. Depending on the data type and business outcomes, some of these algorithms must be selected appropriately. The concept of an algorithm is critical when deciding between successful and unsuccessful AI implementation. -
Software Infrastructure
Software infrastructure is essential to an AI factory as it covers the entire production process flow. Indeed, it integrates several parts of the system, such as data storage, data processing, and even the AI models themselves. The best algorithms and data pipelines will never be delivered with the wrong creative infrastructure. The infrastructure should be able to grow, be dependable, and be tailor-made for AI processing. -
Experimentation Platform
An enhanced hypothesis is an experimentation platform that enables the business to run several simulations and hypotheses. It allows the team to try numerous algorithm choices, calibrate results, and adjust based on real-life circumstances. This area is crucial to the noted evolution and adjustments in business dynamics.
How AI Factories Operate: Architecture and Flow
The components described above combine to make the Architecture of an AI factory able to incorporate all of them inclusively. Below is a high-level diagram that illustrates how an AI factory functions:
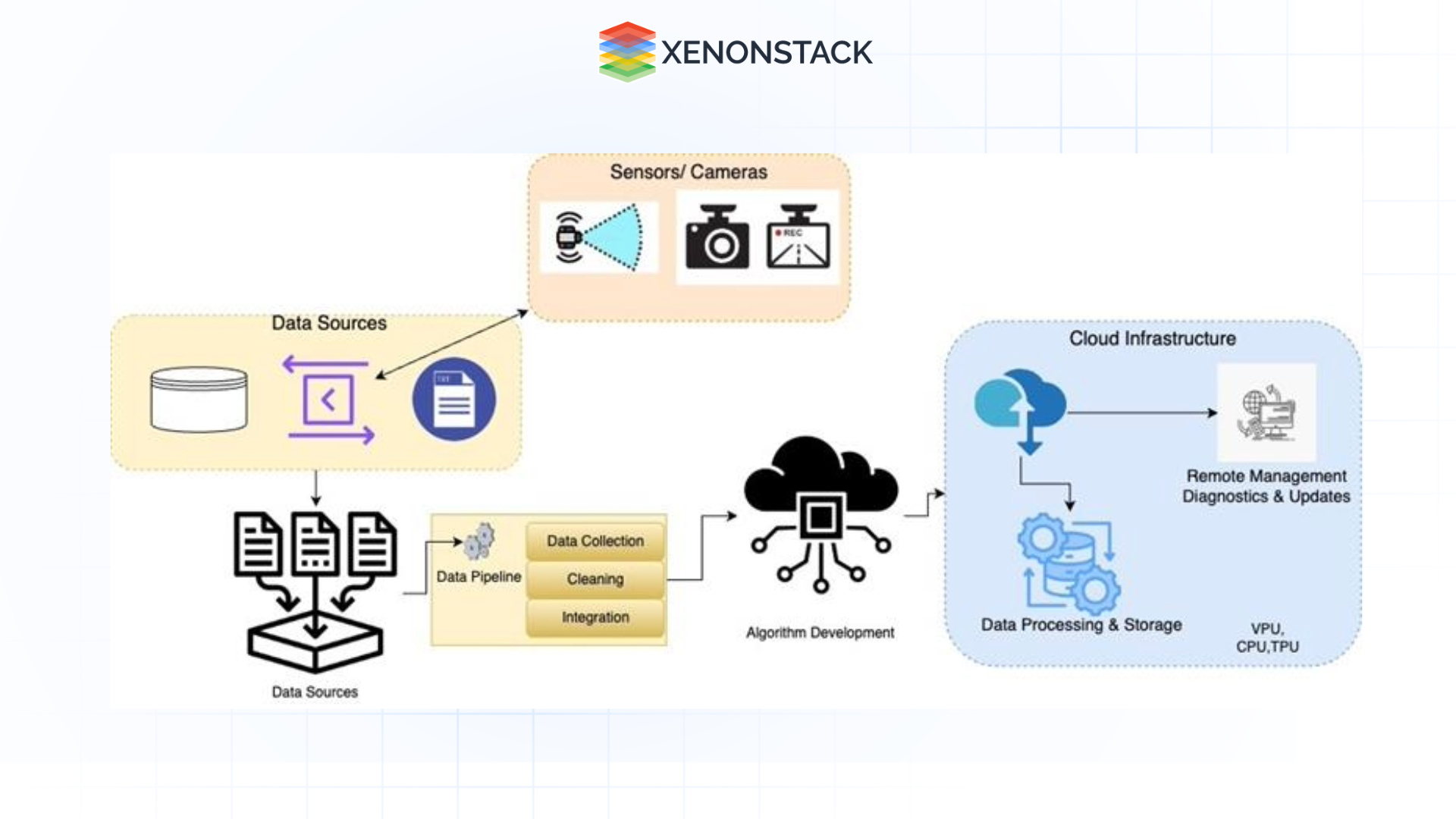
How Data Flows through the AI Factory
-
Data Ingestion: This process starts with receiving raw data from different origins, internal or external. This includes Internet of Things devices, sensors, or users. All this information is then fed to the data pipeline.
-
Data Cleaning and Integration: The data pipeline addresses two major data processing steps: cleaning and integration. It is the process of organizing data so that it can be processed further to complete an analysis of data.
-
Algorithm Selection and Training: The cleaned data is then passed through models developed using statistical models, which enable it to be processed, patterns to be recognized, and even predictions made on the expected outcome.
-
Deployment and Testing: Once the algorithms are trained, they are launched on two types of more scalable infrastructures. An experimentation platform for businesses means they can fine-tune the models using actual conditions.
Real-World Applications of AI Factories
AI factories have found applications in multiple industries, enabling businesses to unlock new capabilities and drive innovation.
Industry
|
Problem
|
AI Factory Solution
|
Manufacturing
|
Frequent downtimes
|
AI models forecast equipment breakdowns, thus cutting their duration.
|
Inefficient inventory management
|
AI means that inventory is intelligently carried out by computing the regular demand and using forecasts to inform the same.
|
|
Inconsistent product quality
|
Machine vision in vision models identifies defects independently, improving the quality.
|
|
Finance
|
Rising financial fraud
|
Artificial intelligence consumes transactions, and the AI models identify fraud as it takes place.
|
Difficulty in assessing risk
|
AI analyzes financial information for market trends, changes, and risk identification.
|
|
Slow decision-making in trading
|
By staying atop market dynamics, AI algorithms facilitate high turnover and automatic trading.
|
|
Healthcare
|
Long diagnostic times and errors
|
AI models help diagnose diseases faster and more accurately using medical data.
|
Generic treatment plans
|
AI uses data to diagnose and advise on which treatment should be given to every patient.
|
|
Inaccurate health predictions
|
AI estimates the condition of the patient and the number of resources that will be required by the patient for treatment.
|
|
Retail/
E-commerce
|
Lack of tailored recommendations
|
AI customizes recommendations for a product by analyzing customers’ behaviours.
|
Seasonal demand fluctuations
|
AI is good at predicting the demand the product is likely to receive; hence, the right stock is ordered.
|
|
Static pricing strategies
|
The current position of the company and competitor actions are used to develop dynamic pricing by AI.
|
|
Gaming
|
Lack of intelligent NPCs and dynamic environments
|
AI introduces proper characters and environments, easily improving the gameplay.
|
Low player retention
|
Intelligence uses information about clients to guide the type of content and difficulty that would be of keen interest to them and thus retain their interest.
|
Future of AI Factories
As the demand for AI solutions grows, the role of AI factories will become even more prominent. Future AI factories will be even more advanced, with improved algorithms, faster processing speeds, and greater scalability. The integration of next-gen technologies like 5G, edge computing, and quantum computing will further enhance the capabilities of AI factories, enabling them to process data even faster and closer to the source. Examples like,
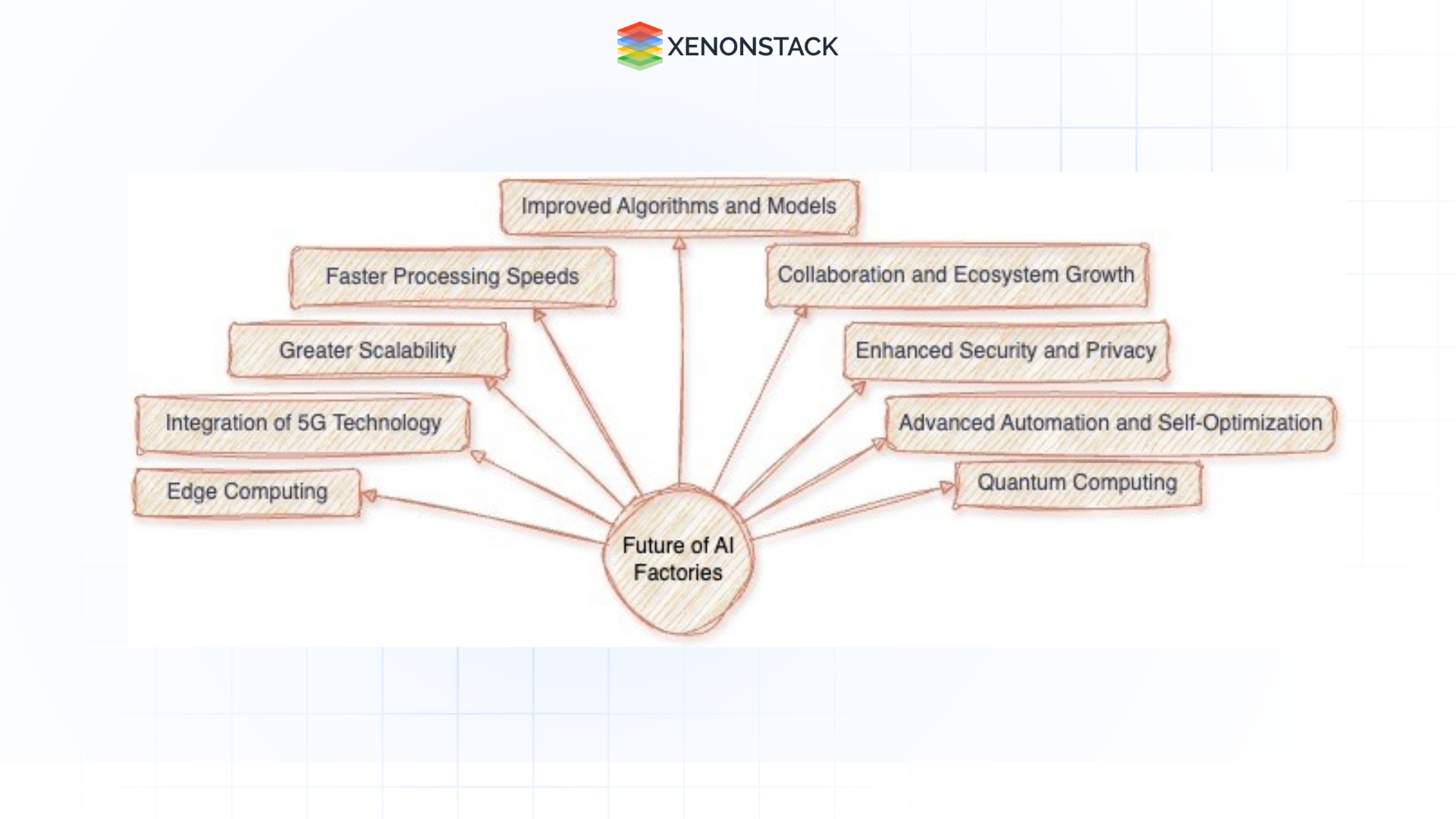
Conclusion
AI factories are widespread to alter the fashion of sourcing AI and already provide a centralized approach to the extensive corporate implementation of AI workloads. By combining data streams, complex analytical computations, strong platform support, and experimentation frameworks, AI factories allow for the extraction of insights and decision-making much faster. As industries move to production-scale AI, AI factories will become fundamental to any enterprise's ability to keep up with the future technological landscape.
Next Steps in AI Factories
Consult our experts about implementing compound AI systems and how industries and departments leverage AI Factories to drive decision-centric operations. Utilize AI to automate and optimize processes, enhancing efficiency and responsiveness across the organization.