Thanks to advanced technologies and social media where content creators such as YouTube, TikTok, Facebook, and Instagram, the world’s internet is flooded with new videos. As this increase fosters massive opportunities to be inventive and to participate, it also presents immense difficulties in regulating the seriousness or profaneness of content on such a large scale.
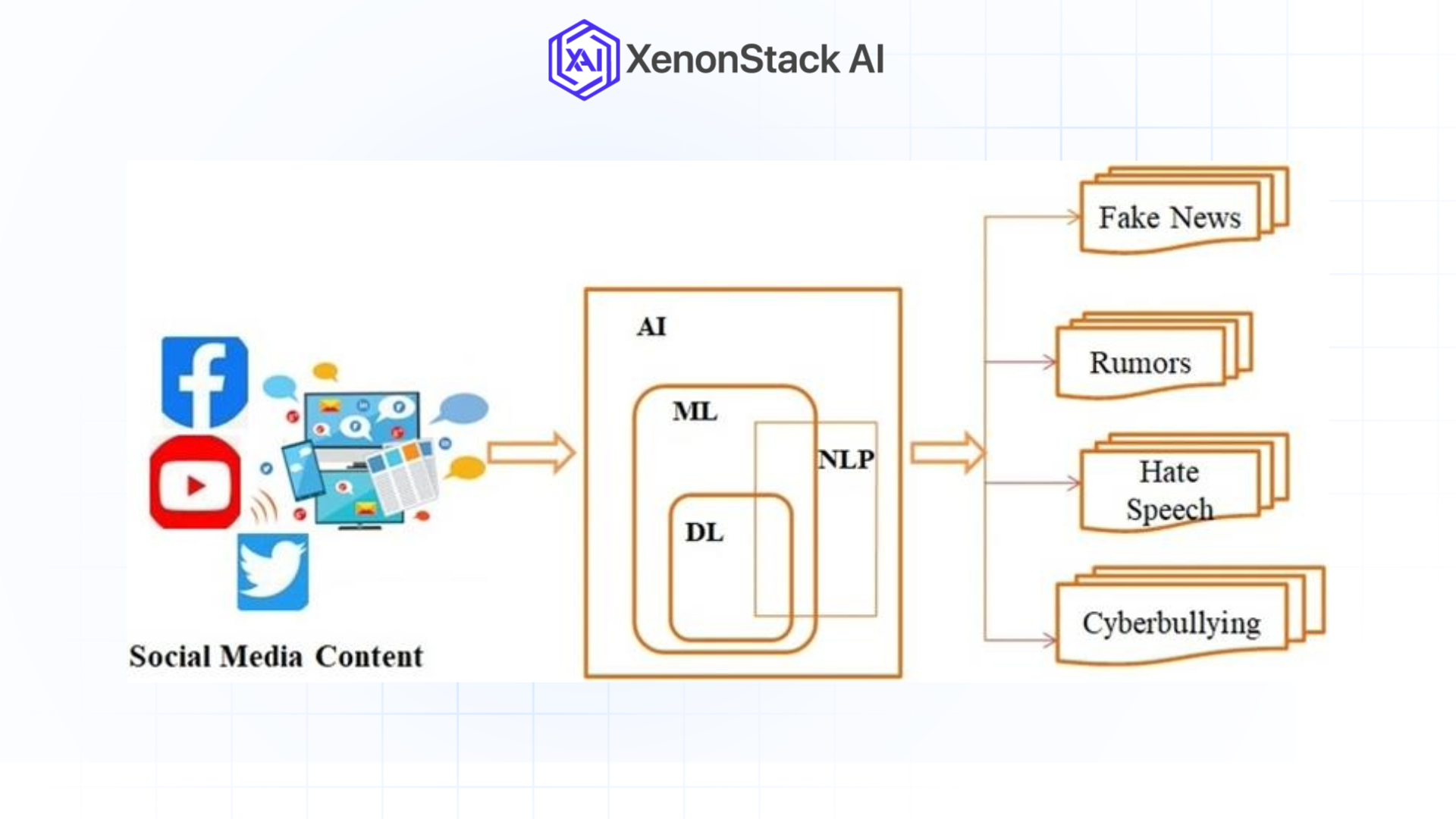
Figure 1: AI-based techniques for detection of detrimental content on SM platforms
Traditional manual interventions, where most of the content is reviewed by humans, are not efficient enough to handle the avalanche of uploads. Moreover, human factors such as mistakes, bias, inefficiency, and procrastination cannot solve the fluidity and internationality of online information. This is where AI video analytics makes a worthy and effective solution to content moderation in real-time.
Thanks to technological advances in artificial intelligence, machine learning, computer vision, natural language processing, or NLP, technology has been developed to monitor video material in real time to detect and filter out hate speech and other undesirable elements such as pornographic material, violence, and fake news. These technologies make online experiences safer and help navigate local and international restrictions.
Why AI-Powered Video Analytics?
-
Scalability at Speed
Hear this: millions of videos are uploaded to various social media platforms every day. Manual review teams cannot handle this volume, whereas AI systems are designed to handle large volumes of content within seconds.
-
Consistency and Objectivity
This is a better system than human reviewers because humans often bring in their biases or may get tired, negatively influencing the results to be issued.
-
Cost Efficiency
Automation of moderation minimizes the need for organizations to employ a large number of human moderators, reducing the expenses of operating an online platform.
-
Real-Time Responsiveness
AI solutions allow several instances of analyzing and reporting improper content during or after it was posted so the platforms can step in and prevent the wide distribution of such content.
Basic Technologies That Power AI for Videos
AI-powered video analytics integrates several advanced technologies to deliver accurate and real-time moderation:
Computer Vision
The video frames are decrypted using artificial intelligence computer vision models, which allow us to identify vulgar scenes, violent scenes, or prohibited symbols. Such systems are designed to recognize objects, scenes, and actions and provide in-depth content analysis.
Latest Advancements: Current versions like Vision Transformers (ViT) and SAM (Segment Anything Model) enable us to detect objects' exact pixels and shapes with superb detailing. Such developments allow AI to identify minor iconizations of hatred or other nonverbal signs of impropriety. 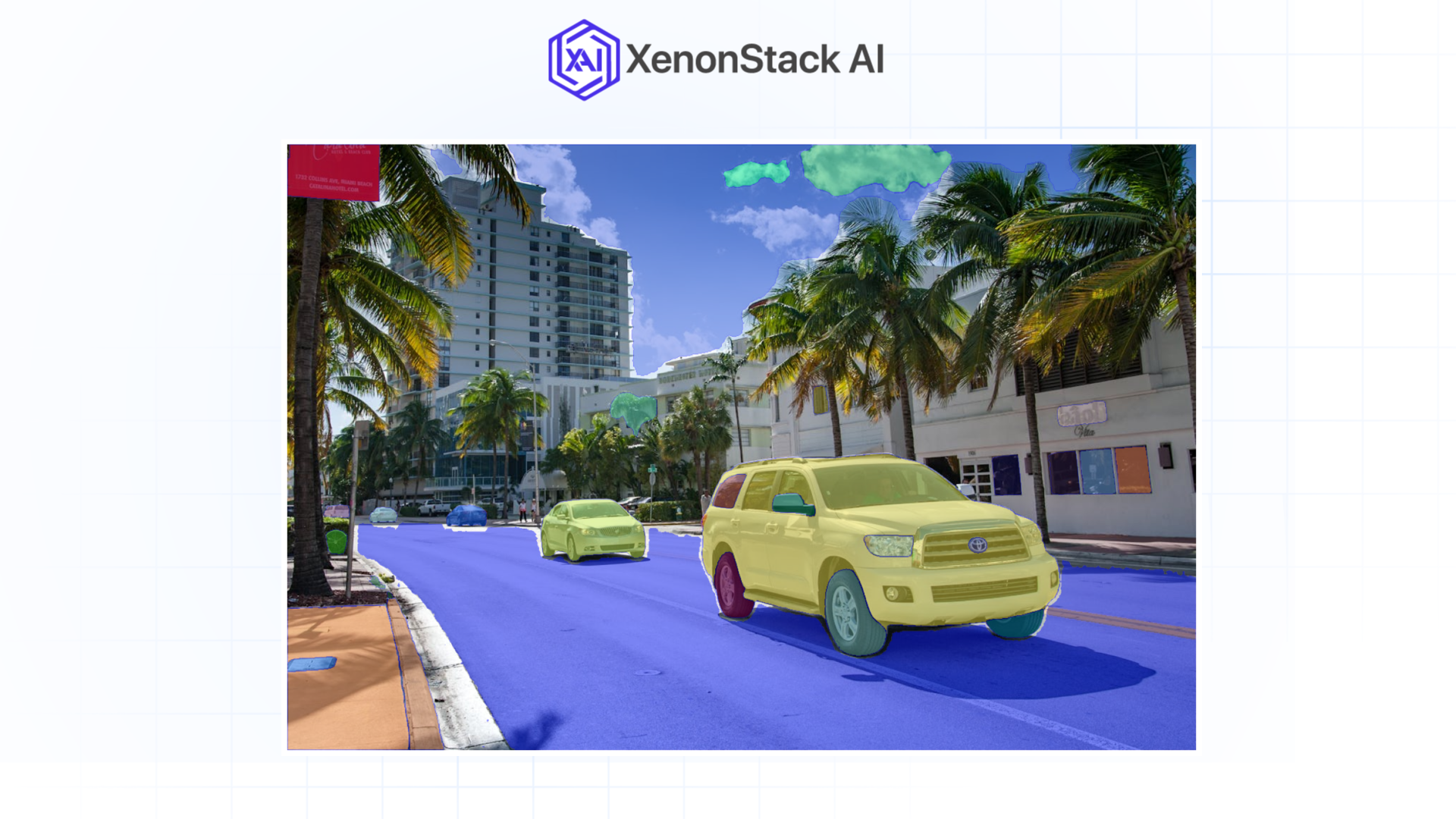
Figure 2: Image inference result using Segment Anything Model
Natural Language Processing
Video audio could be narration or dialogue; video subtitles might be text streams or bubbles. Such algorithms transcribe and analyse this text according to toxic speech, hate speech, or disinformation guidelines.
Latest Advancements: In social media, newer multimodal models like OpenAI’s CLIP and the vision-enabled GPT can further deepen understanding of the context of videos.
Figure 3: Process flow for text-based hate speech detection using machine learning.
Audio Analysis
AI can also transcribe text from spoken words and analyze a track's tone, pitch, and tone for elements of aggression, harassment, or distress.
Example: AI can identify threatening tones when speaking abusive language, even when such malicious words are missing.
Behavioural Analysis
These new-age AI solutions monitor videos for any traces of violent or other perilous action, trolling in large groups or fraudulent activity.
Latest Advancements: Static and dynamic features in video content are better analyzed if graph neural networks (GNNs) and behavioural AI models are used to investigate patterns and interactions.
Multimodal Fusion
The synergy from analyzing visuals, text, and audio information enables AI systems to analyze video content comprehensively, escalating the detection of suspicious content and eradicating percent falsification.
Latest Advancements: Meta’s ImageBind framework combines various data sources to analyse all at once and enables complex moderation tasks. 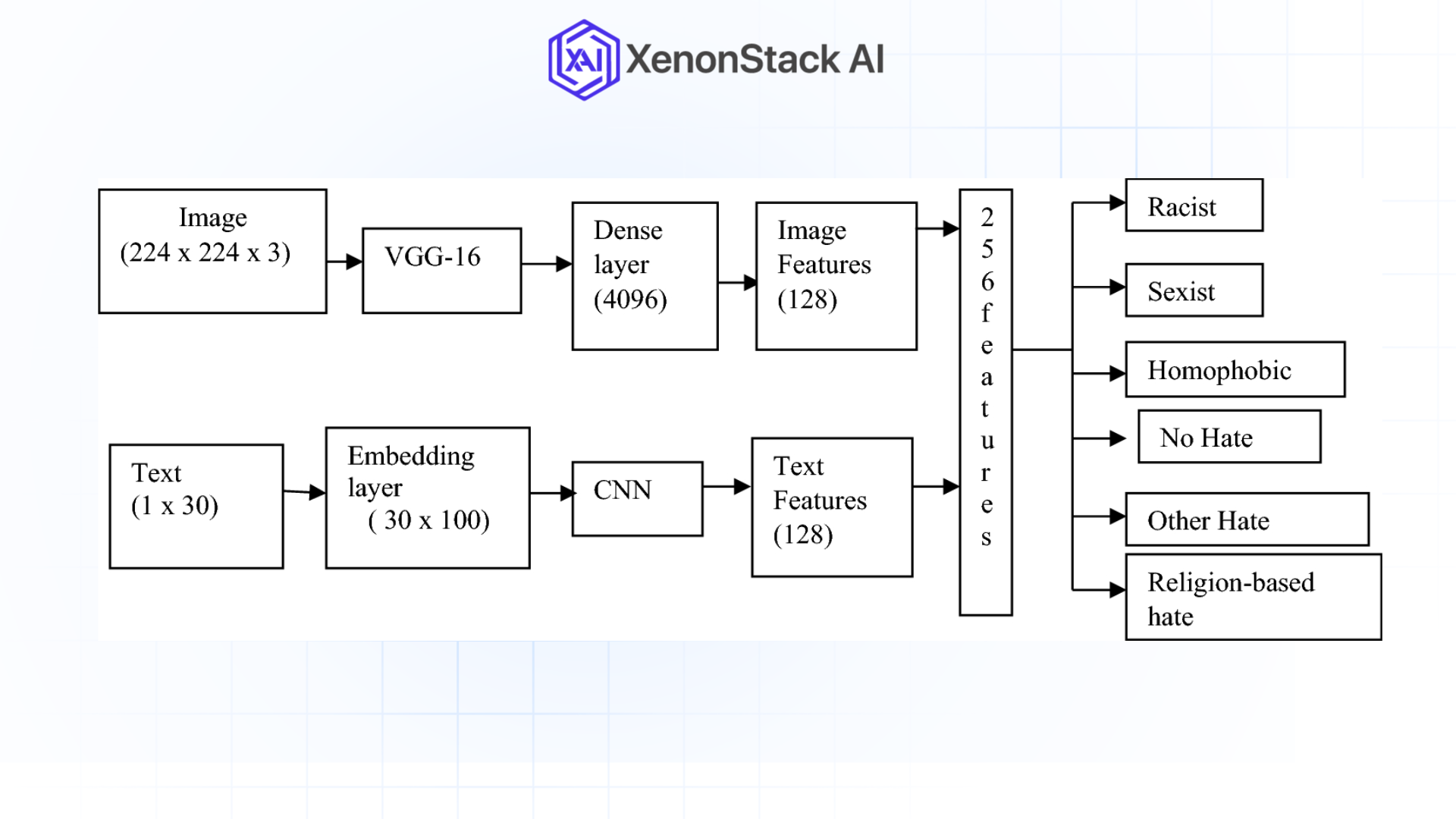
Figure 4: Neural network model architecture for multimodal hate speech classification
Best AI-Powered Content Moderation Systems to Look For
AI-powered video moderation systems offer several advanced features:
-
Contextual Understanding
It can also understand context through the engagement of video and audio and the relationship between text and media. For instance, it can discern between a shooting of violence as used in the making of a documentary and a shooting in a propagating violence video analytics surveillance.
-
Scalable Moderation
The current situation means that one does not have to worry about anything appearing online as a video since AI systems can track tens of millions of videos at a time.
-
Adaptive Learning
Machine learning models are constantly updated by integrating human feedback and flagged content into the training data, ensuring they remain relevant to current trends.
-
Cultural Sensitivity
AI applications are programmed to understand the relevance of regions and cultures and avoid conflicting with the policies and laws of the respective areas.
-
Compliance Support
AI tools enable platforms to comply with and abide by laws like the DSA and other global content regulation laws.
AI video analytics in the real world
-
Social Media Platforms
YouTube, TikTok, and Facebook utilize AI to scrutinize thousands of clips daily for violations of policies of hate speech, violence, or pornography.
Figure 5: Illustration of the real-time pipeline for sensitive content detection
-
Live Streaming Platforms
X (formerly Twitter) and Facebook Live use AI-implemented filtering to flag material immediately during live broadcasts.
-
Electronic Commerce and Online Marketplace
Real product videos must also adhere to policies the AI moderation platform places so that unauthorized items, such as fake or banned products, are not advertised.
-
Higher Education & Corporate Training
In this area, educational platforms apply AI to filter what is discussed in class to eliminate the effect of conveying the wrong message. Further, it helps present the right content for the learning activities per the targets.
-
News and Media Platforms
Uploaded videos are scanned for fake or malicious information, and therefore, news platforms are credible and ethical video analytics tools for the journalistic profession.
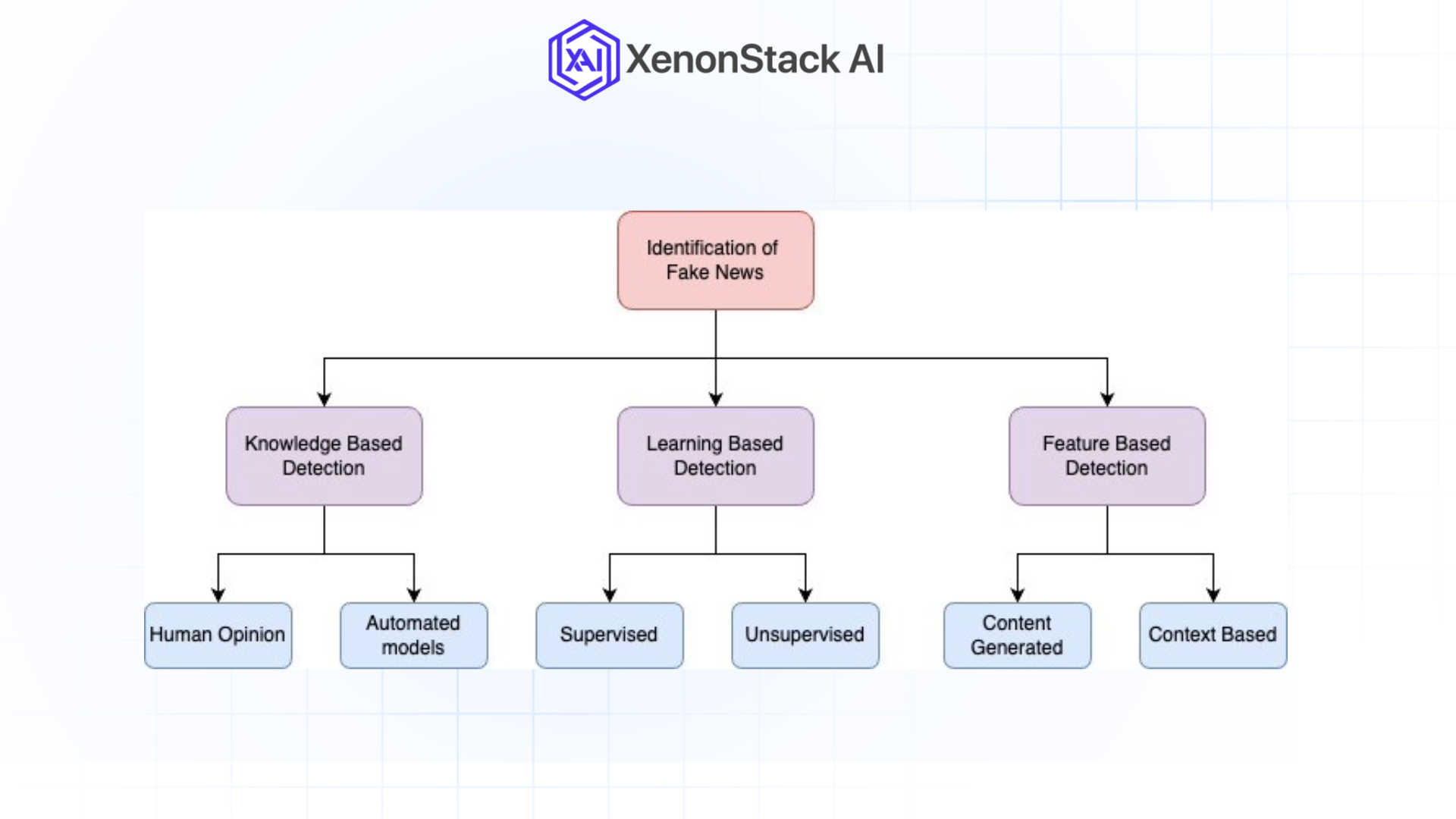
Figure 5: Different approaches towards fake news identification
Recent Advancements Shaping AI Video Analytics
-
The application of Edge AI for Real-Time Moderation
Some newer approaches to moderation are performed locally on the user’s device or a local server, bordered by edge computing.
Example: The Jetson platform from NVIDIA deals with the moment real-time processing of video analytics that can run on the edge.
-
Deepfake and Generative AI Detection
Most platforms are implementing technologies to call out manipulated content that may result from deploying these technologies, such as deepfakes and artificial intelligence videos.
Example: Microsoft’s Video Authenticator can detect deepfakes by looking at tiny components of frames.
-
Explainable AI (XAI)
A list of explainability tools' uses shows that such tools make AI decision-making transparent, helping platforms clarify their moderation choices and avoid scandals.
Example: Google’s Explainable AI tools show how moderation models thinking processes that lead them to their decisions and are therefore transparent.
-
Emotion Recognition
Technical processing of video streams includes the recognition of signals in which the subject is depicted to be experiencing discomfort, aggression, or any other form of diktat over feelings.
Example: Affectiva’s emotion AI video analytics add facial and vocal emotion recognition to their set.
-
Super diversification and cross-cultural analysis
New AI models enable blocking and filtering services in real-time and for different languages and accents people use in other countries.
Example: In turn, Meta’s SeamlessM4T provides a solution for real-time content moderation by simultaneously processing speech and/or text in multiple languages.
Challenges in AI-Powered Video Moderation
Despite its advancements, AI-powered video analytics faces challenges:
-
Contextual Errors
The vulnerabilities are that AI systems might fail to decipher satire art or educational content as safe.
Solution: Implementation of further development of multimodal analysis and better comprehension of contextual models.
-
Bias and Fairness
An AI system's moderation results may be skewed, mainly because the systems are trained using unfair datasets.
Solution: Adequate audits, training on the protocol’s various datasets, and the inclusion of human supervision in the process protect against bias.
-
Privacy Concerns
Featuring video content provokes privacy concerns. This approach is problematic since users’ data are integrated into centralized systems.
Solution: Edge AI and federated learning address these issues because no data are transferred to other locations.
-
Emerging Threats
The change in trends in the content that is considered harmful develops quickly, making the AI systems post-operational with the new forms and symbols that the trends may be taking.
Solution: More often, retraining and real-time substituting of datasets help to make it responsive to the new threats.