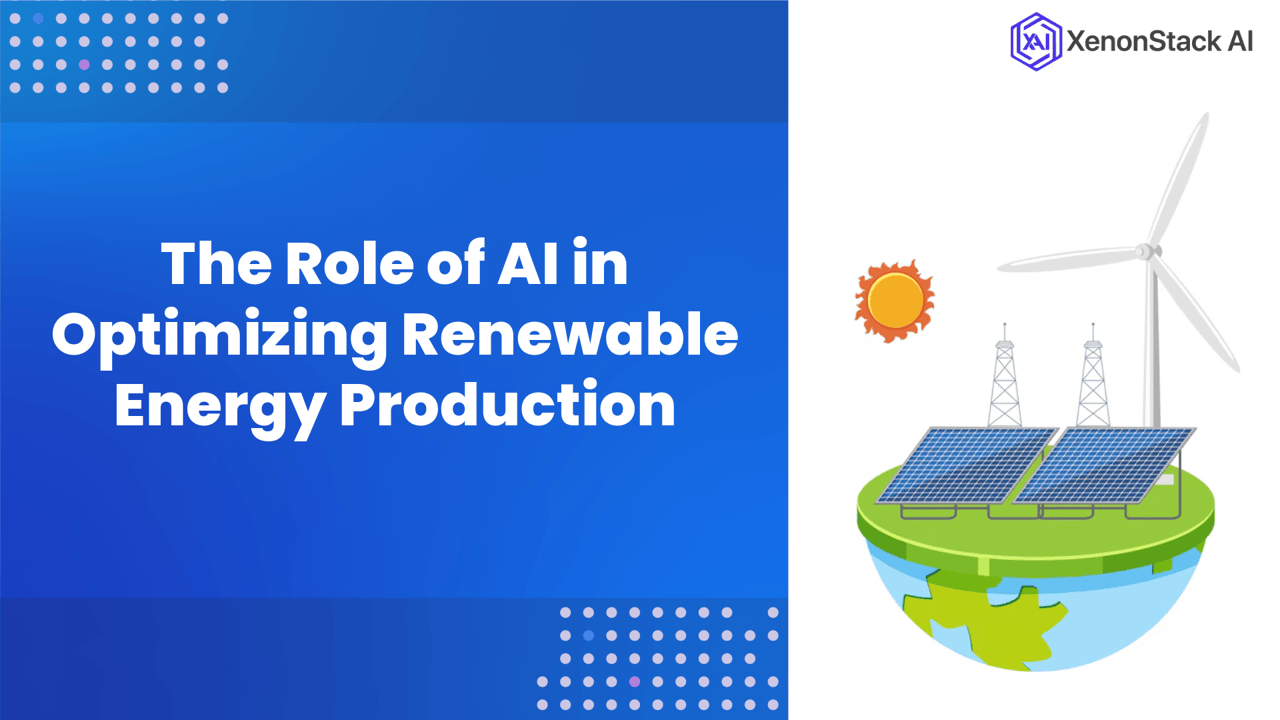
Renewable energy has become the cornerstone of global efforts to combat climate change and achieve sustainable development. Wind, solar, and other renewable energy sources are transforming energy production. However, the intermittent nature of these energy sources presents significant challenges in maintaining a stable and efficient energy supply. Artificial intelligence (AI) emerges as a game-changing solution, offering powerful tools to optimize renewable energy production, address challenges, and drive innovation in the energy sector.
This blog delves into how AI is revolutionizing renewable energy production, exploring its methodologies, real-world applications, benefits, challenges, and future trends.
The Role of AI in Renewable Energy Optimization
AI employs advanced algorithms, machine learning models, and predictive analytics to analyze vast datasets and improve decision-making processes. In renewable energy production, AI offers solutions for:
-
Predicting Energy Generation: AI models can forecast energy output from wind turbines, solar panels, and other renewable sources based on historical and real-time weather data.
-
Enhancing Grid Integration: AI facilitates the seamless integration of renewable energy into power grids by predicting demand, balancing supply, and preventing blackouts.
-
Improving Equipment Efficiency: AI-driven predictive maintenance reduces downtime and prolongs the lifespan of renewable energy equipment.
-
Optimizing Storage Systems: AI algorithms manage energy storage systems, determining optimal charging and discharging cycles to ensure a steady energy supply.
AI Techniques for Renewable Energy Optimization
The global transition to renewable energy is a cornerstone of sustainable development, and artificial intelligence (AI) is pivotal in accelerating this shift. By leveraging advanced AI techniques, energy providers, grid operators, and policymakers can optimize renewable energy systems, enhance efficiency, and address the challenges of integrating variable energy sources. Reducing Carbon Emission in the Energy Sector. Below are the key AI techniques transforming the renewable energy sector:
Predictive Analytics for Energy Forecasting
AI-powered predictive analytics models use historical and real-time data to accurately forecast energy generation and demand. This is particularly crucial for renewable energy sources like solar and wind, which are inherently variable.
Techniques like:
-
Time-Series Analysis: Models historical trends to predict future energy outputs.
-
Machine Learning (ML) Models: Employ neural networks and gradient boosting for improved accuracy in predicting weather patterns and energy generation.
Real-time monitoring and Control
IoT sensors and AI algorithms enable real-time monitoring and control of renewable energy assets. AI systems:
-
Detect inefficiencies or faults in solar panels, wind turbines, or grid infrastructure.
-
Optimize energy production by adjusting operations based on real-time conditions.
-
Utilize Reinforcement Learning to improve decision-making in grid management dynamically.
Grid Management and Load Balancing
The integration of renewable energy into traditional grids requires sophisticated grid management solutions. AI aids in:
-
Dynamic Load Balancing: Ensuring stable energy distribution by predicting and managing fluctuations in supply and demand.
-
Energy Storage Optimization: AI models help determine optimal battery charging and discharging cycles to enhance grid reliability.
Smart Energy Trading
AI techniques facilitate the emergence of decentralized energy markets where prosumers (producers and consumers) trade energy. Key applications include:
-
Blockchain Integration: Ensures secure and transparent transactions.
-
Market Prediction Models: Optimize trading strategies by analyzing market trends and participant behaviour.
Fault Detection and Predictive Maintenance
AI-powered diagnostic tools identify faults in renewable energy systems before they escalate. Techniques like:
-
Anomaly Detection Algorithms: Recognize deviations from normal operating conditions.
-
Predictive Maintenance Models: Forecast equipment failures and recommend proactive maintenance, reducing downtime and costs.
Energy Efficiency Optimization
AI-driven energy management systems analyze consumption patterns to suggest efficiency improvements. Techniques include:
-
Optimization Algorithms: Minimize energy waste by recommending equipment upgrades or operational changes.
-
Demand Response Systems: AI predicts peak demand periods and suggests shifts in energy usage to reduce strain on the grid.
Renewable Energy Siting and Resource Assessment
AI algorithms analyze geographic and meteorological data to identify optimal locations for renewable energy installations. These techniques:
- Incorporate Geographic Information Systems (GIS) data to assess feasibility.
- Use simulation models to predict energy yield and environmental impact.
AI-driven Hybrid Energy Systems
Hybrid systems combine multiple energy sources like solar, wind, and batteries to ensure reliability. AI enables:
-
Energy Source Optimization: Balances energy generation from different sources to meet demand efficiently.
-
Hybrid System Simulation: Models performance under various scenarios to design robust systems.
Consumer-centric Energy Solutions
AI enhances customer engagement by providing personalized energy-saving recommendations and demand-side management tools responsible ai in retail.
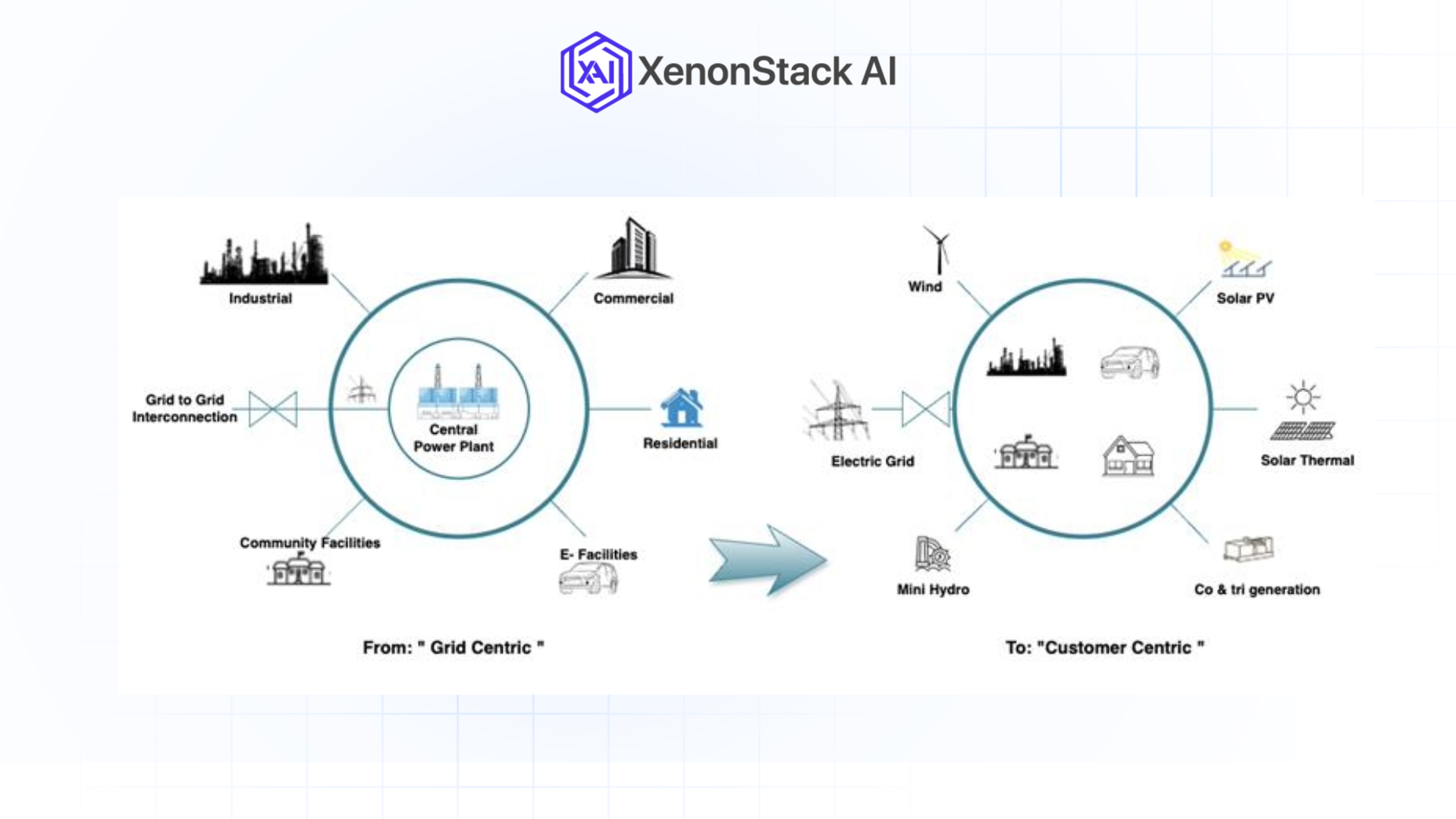
Examples include:
-
AI Chatbots: Assist consumers in understanding and managing their energy usage.
-
Behavioral Analytics: Encourage energy-saving habits through gamification and personalized incentives.
Real-World Applications of AI in Renewable Energy
Solar Energy Optimization
AI improves the efficiency of solar energy systems by predicting solar radiation levels and optimizing the orientation of solar panels. For example:
-
Google’s Project Sunroof: Uses AI to estimate solar panel output based on rooftop size, shape, and weather conditions.
-
Tesla’s Solar Roof: Combines AI and IoT to monitor and manage solar energy production and storage in real-time.
Wind Energy Optimization
AI enhances wind energy production by predicting wind speeds and directions. For instance:
-
DeepMind and Google: AI reduced energy costs for wind farms by 20% by predicting wind power output 36 hours in advance.
-
Siemens Gamesa: Utilizes AI for turbine performance analysis and predictive maintenance.
Energy Storage Management
Efficient energy storage is critical for renewable energy systems. AI optimizes battery performance by determining ideal charging and discharging times. Companies like Fluence and Stem Inc. use AI to enhance energy storage systems’ efficiency and reliability.
Smart Grids
AI enables innovative grid systems to balance energy supply and demand. For instance:
-
IBM’s Smarter Energy: Uses AI to predict energy demand and manage renewable energy distribution.
-
National Grid ESO: Employs AI for real-time grid balancing to accommodate renewable energy variability.
Hybrid Renewable Systems
AI facilitates the integration of multiple renewable energy sources. For example:
-
AI can optimize a hybrid system combining solar, wind, and hydropower to ensure a consistent energy supply.
-
GE Renewable Energy: Uses AI to optimize multi-source energy systems.
Benefits of AI in Renewable Energy Production
-
Increased Energy Efficiency: AI minimizes energy waste by optimizing production and consumption patterns. For example, AI-powered models can adjust turbine blade angles to maximize wind energy capture.
-
Cost Reduction: AI reduces operational costs by improving system efficiency, optimizing resource allocation, and predicting maintenance needs.
-
Enhanced Reliability: AI’s predictive capabilities ensure a steady energy supply by addressing the intermittency of renewable energy sources.
Environmental Benefits
-
Optimized energy systems reduce greenhouse gas emissions and minimize the environmental impact of energy production.
-
Scalability: AI-driven solutions can be scaled to accommodate growing energy demands and expanding renewable energy systems.
Challenges in Implementing AI for Renewable Energy
-
Data Quality and Availability: AI models require large volumes of accurate and diverse data. Inconsistent or incomplete data can hinder AI’s effectiveness.
-
Integration with Legacy Systems: Incorporating AI into existing energy infrastructure can be complex and costly.
-
Cybersecurity Risks: AI systems are vulnerable to cyberattacks, which can compromise energy production and grid stability.
-
High Initial Costs: Developing and deploying AI systems for renewable energy requires a significant upfront investment.
-
Regulatory and Policy Barriers: Lack of standardized regulations can delay the adoption of AI in renewable energy systems.
Future Trends in AI-Driven Renewable Energy
Advanced Predictive Models: Future AI models will incorporate more sophisticated algorithms to improve accuracy in forecasting energy production and demand.
Decentralized Energy Systems: AI will enable the management of decentralized energy systems, where consumers also act as producers (prosumers).
Blockchain Integration: Blockchain technology can enhance the transparency and security of AI-driven energy systems. Autonomous Energy Systems: AI will enable fully autonomous energy systems capable of self-optimization and decision-making. AI-Powered Renewable Energy Policy: AI can provide data-driven insights to inform and improve renewable energy policies and regulations.
Conclusion for Renewable Energy Production
AI is transforming renewable energy production, addressing key challenges, and unlocking new opportunities for efficiency, reliability, and sustainability. As businesses and governments prioritize renewable energy, AI will play an increasingly vital role in achieving a cleaner and more sustainable energy future. By leveraging advanced algorithms, predictive analytics, and IoT integration, AI empowers energy producers to optimize renewable energy systems, reduce costs, and minimize environmental impact.
Despite challenges, the benefits of AI-driven renewable energy far outweigh the obstacles, paving the way for a greener and more resilient energy sector. The future of energy lies in the seamless integration of AI and renewable energy, promising a world where sustainable energy is accessible, efficient, and impactful. Companies and policymakers must embrace these advancements to drive the global transition towards a more sustainable and equitable energy landscape.
Next Steps with Renewable Energy Production
Talk to our experts about implementing compound AI systems and how industries and different departments use Agentic workflow and Decision Intelligence to become decision-centric. Utilize AI to automate and optimize renewable energy production, improving efficiency and sustainability in energy systems. AI can enhance energy forecasting, optimize grid operations, and improve energy storage management, ensuring reliable and efficient renewable energy generation and distribution.