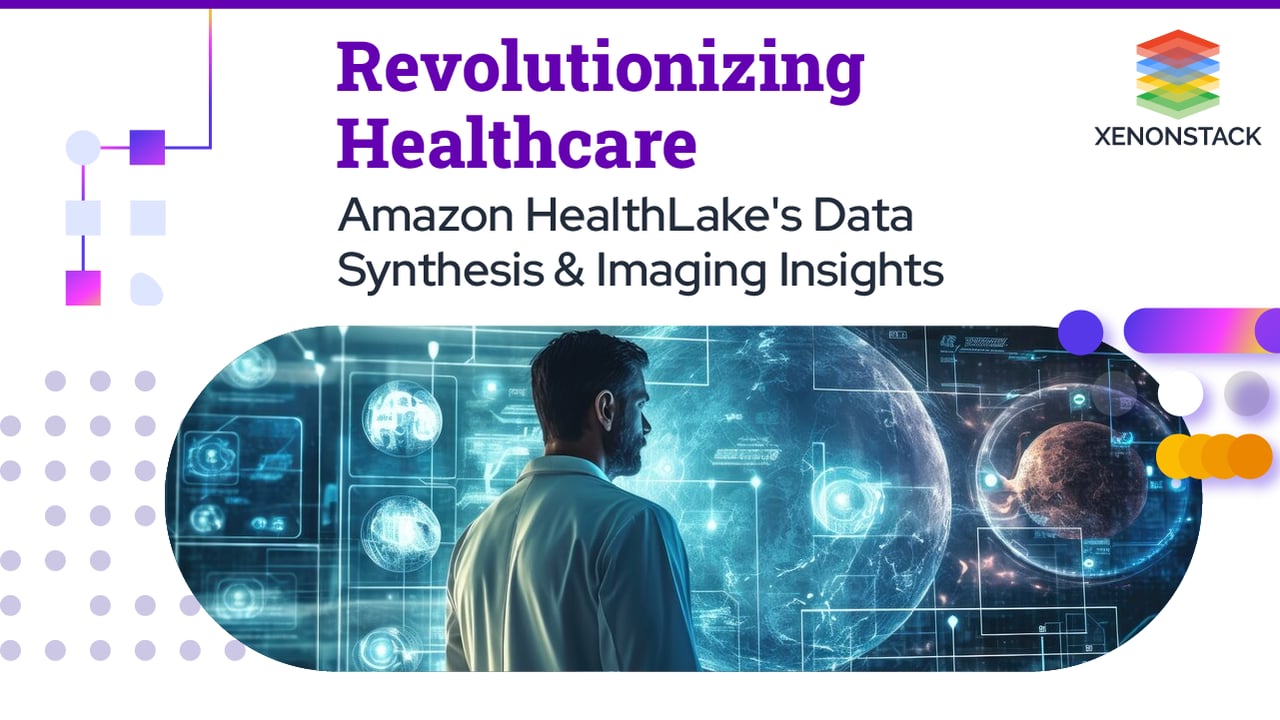
Overview of Amazon HealthLake
Healthcare data is a treasure trove of insights, but managing it can be complex and time-consuming. Enter Amazon HealthLake, a cloud-based Amazon Web Services (AWS) service designed to streamline healthcare data management.
Imagine a secure and compliant data lake specifically built for healthcare organizations. That's the essence of Amazon HealthLake. It empowers healthcare providers, insurers, and pharmaceutical companies to:
-
Store Data Securely: HealthLake provides a centralized repository for all your healthcare data, ensuring its safety and accessibility. This eliminates the need for disparate systems and simplifies data governance.
-
Transform Data for Insights: Raw healthcare data is often messy and unstructured. HealthLake offers tools to transform this data into a usable format, making it easier to analyze and extract valuable insights.
-
Analyze Data with Ease: Leverage HealthLake's built-in analytics capabilities to uncover hidden patterns and trends in your healthcare data. This can inform strategic decision-making, improve patient care, and accelerate research efforts.
-
Share Data Securely: Collaboration is key in healthcare. HealthLake facilitates secure data sharing with authorized personnel and institutions, fostering innovation and collaboration across the healthcare ecosystem.
But Amazon HealthLake's impact goes beyond basic data management. It plays a critical role in enabling the transformative power of generative AI in healthcare. Here's how:
-
Centralized Data Access: Generative AI models require vast amounts of high-quality healthcare data for training. HealthLake provides a centralized repository, simplifying access for developers crafting these AI models.
-
Improved Data Quality: HealthLake's data transformation tools ensure the data used for training generative AI models is clean, consistent, and accurate. This leads to the creation of more reliable and robust AI models.
-
Enhanced Security and Compliance: Generative AI applications often involve sensitive patient data. HealthLake's robust security features and compliance with healthcare regulations like HIPAA ensure responsible and ethical use of data throughout the AI development process.
Amazon HealthLake acts as a catalyst for generative AI in healthcare by providing a secure, compliant, centralised platform for healthcare data management. This unlocks a world of possibilities, paving the way for advancements in drug discovery, personalized medicine, and more accurate medical diagnoses.
Synthetic Data Generation Techniques in Healthcare
Data scarcity is a persistent challenge in healthcare. Sensitive patient information often requires strict privacy controls, limiting data availability for research and development. Here's where synthetic data generation emerges as a game-changer.
Synthetic data generation techniques create new, realistic data that statistically mirrors real-world data. This empowers healthcare professionals to overcome data limitations and unlock new possibilities:
-
Generative Adversarial Networks (GANs): Imagine two AI models locked in a competition. One (generator) creates synthetic data, while the other (discriminator) tries to distinguish it from real data. As they continuously learn from each other, the generator produces increasingly realistic synthetic data. This technique holds immense potential for generating synthetic medical images for AI model training.
-
Variational Autoencoders (VAEs): These AI models compress real data into a lower-dimensional latent space, capturing its core characteristics. VAEs can then generate new data points that resemble the original data while maintaining statistical properties. This approach can create synthetic patient records for clinical trial simulations.
-
Augmentation Techniques: Real-world data can be enriched by adding noise, variations, or occlusions (hiding parts of the data) through synthetic data generation. This helps AI models become more robust in handling diverse data and improves their generalizability, leading to more accurate diagnoses and predictions.
Benefits Beyond Quantity
Synthetic data offers several advantages over traditional data collection methods:
-
Enhanced Privacy: By not using real patient data, synthetic data safeguards patient privacy, addressing ethical concerns and regulatory hurdles.
-
Reduced Costs: Generating synthetic data is often cheaper than collecting and anonymizing real-world data, making healthcare AI development more cost-effective.
-
Improved Data Quality: Synthetic data generation techniques can address inconsistencies and missing information, leading to higher-quality data for training AI models.
Looking Ahead: A Brighter Future
Synthetic data generation is not a replacement for real-world data but rather a powerful tool to supplement it. By leveraging these techniques, researchers and developers can overcome data scarcity and accelerate innovation in healthcare AI. This paves the way for advancements in drug discovery, personalized medicine, and improved patient care.
Applications of Generative AI in Medical Imaging Analysis
Medical imaging plays a pivotal role in diagnosing and treating diseases. However, traditional analysis methods can be time-consuming and prone to human error. Enter generative AI, a transformative technology poised to revolutionize medical imaging analysis and unlock a new era of healthcare. Here's how generative AI is making a significant impact:
1. Synthetic Image Creation: One of the most exciting applications is the ability to create synthetic medical images. This eliminates the need for vast amounts of real-world patient data, which can be scarce due to privacy concerns or limited availability. These synthetic images can be used for training and validating AI-powered diagnostic tools, ultimately leading to more accurate diagnoses.
2. Augmenting Real-World Images: Generative AI can enhance real-world medical images by introducing variations or noise. This "data augmentation" helps AI models become more robust. Imagine training an AI to detect lung cancer in X-rays. By augmenting real X-rays with lighting or image quality variations, the AI learns to recognize the disease even in less-than-ideal scenarios, improving its overall diagnostic accuracy.
3. Image Segmentation: Accurate segmentation of medical images, where different anatomical structures are identified, is crucial for tasks like tumour detection and treatment planning. Generative AI can automate and improve this process by learning the subtle differences between various tissues and organs within an image. This saves radiologists valuable time and leads to more precise diagnoses and treatment plans.
4. Personalized Medicine: Generative AI can analyze a patient's specific medical history and imaging data to create personalized simulations. This can predict how a disease might progress or how a patient might respond to a particular treatment. This personalized approach to medicine holds immense potential for improving patient outcomes.
5. Early Disease Detection: Generative AI models can be trained to identify subtle anomalies in medical images that the human eye may miss. This allows for earlier detection of diseases, leading to improved patient outcomes and potentially saving lives.
Beyond Efficiency
Generative AI offers benefits that reach beyond just improved efficiency:
-
Reduced Costs: By creating synthetic data and automating tasks, generative AI can potentially reduce healthcare costs associated with traditional imaging analysis methods.
-
Improved Accessibility: Generative AI applications can be deployed in remote locations where access to experienced radiologists might be limited, potentially improving healthcare access for underserved populations.
The Future of Medical Imaging
As generative AI evolves, we can expect even more groundbreaking applications to emerge. Imagine a world where AI-powered diagnostic tools, trained on synthetic and augmented images, become ubiquitous, leading to faster, more accurate diagnoses and personalized treatment plans for all. This is the future that generative AI promises in medical imaging analysis.
Benefits of Using Synthetic Data for Healthcare Applications
Traditionally, healthcare applications have relied heavily on real-world patient data. However, collecting and using such data often presents challenges like privacy concerns, limited availability, and potential biases. Here's where synthetic data generation emerges as a game-changer, offering a wealth of benefits for healthcare applications:
-
Enhanced Patient Privacy: Synthetic data, by definition, doesn't contain any real patient information. This eliminates privacy risks and ethical concerns associated with using real data, allowing researchers and developers to freely explore healthcare applications without compromising patient confidentiality.
-
Reduced Costs and Time: Obtaining and anonymizing real-world patient data can be costly and time-consuming. Synthetic data generation offers a cost-effective and faster alternative, accelerating research and development cycles for healthcare applications.
-
Improved Data Quality and Consistency: Real-world data can be riddled with inconsistencies and missing information. Synthetic data generation techniques can address these issues by creating high-quality, consistent data that accurately reflect real-world scenarios. This leads to more reliable and robust healthcare applications.
-
Overcoming Data Scarcity: Certain diseases or medical conditions may have limited real-world data. Synthetic data generation can bridge this gap by creating realistic data sets for specific scenarios, allowing researchers to develop and test healthcare applications for less common conditions.
-
Data Augmentation and Generalizability: Generative AI techniques can "augment" real-world data by adding noise, variations, or occlusions. This helps train healthcare AI models to handle diverse scenarios and generalize their learnings to real-world situations, leading to more accurate diagnoses and predictions.
-
Facilitating Personalized Medicine: Researchers can develop personalised simulations by creating synthetic data specific to a patient's medical history. This can predict disease progression or a patient's response to specific treatments, paving the way for a more personalized approach to medicine.
Ethical and Regulatory Considerations in Healthcare Data Generation
While synthetic data offers exciting possibilities, ethical and regulatory considerations remain:
-
Data Bias: Synthetic data can inherit biases from the real-world data used to train the generative models. This could lead to biased AI applications in healthcare, potentially harming underrepresented groups.
-
Data Security and Privacy: Highly realistic synthetic data, including medical images or patient records, raises security concerns. Malicious actors could misuse it for deepfakes or medical fraud.
-
Explainability and Transparency: Understanding how generative models arrive at particular synthetic data points can be challenging. This lack of transparency raises ethical concerns, especially when dealing with sensitive healthcare data.
-
Regulatory Uncertainty: The regulatory landscape surrounding synthetic data in healthcare is evolving. Clear regulations are needed to ensure responsible and ethical use of this technology.
Integration of Amazon HealthLake with GenAI
Generative AI holds immense potential to revolutionize healthcare. However, unlocking this potential requires access to high-quality, secure healthcare data. This is where Amazon HealthLake steps in, acting as a critical bridge between real-world healthcare data and the world of generative AI models.
-
Centralized Data Repository: HealthLake provides a secure and centralized repository for healthcare organizations to store all their health data, from electronic health records (EHRs) to medical images. This eliminates the need for disparate data silos, making it easier for developers to access the data they need to train generative AI models.
-
Improved Data Quality and Compliance: HealthLake's built-in data transformation tools ensure the data used for training generative AI models are clean, consistent, and compliant with healthcare regulations like HIPAA. This leads to the creation of more reliable and robust AI models.
-
Streamlined Data Sharing: HealthLake facilitates secure data sharing with authorised institutions and external AI developers. This fosters collaboration and empowers developers to leverage real-world data from a wider pool of sources, enriching the training process for generative AI models.
-
AWS Generative AI Innovation Center: Amazon's initiative further strengthens the link. The centre focuses on developing and deploying cutting-edge generative AI solutions for healthcare. It leverages HealthLake's data infrastructure to train and deploy these AI models, accelerating innovation in synthetic image creation for medical diagnosis.
-
Real-World Data Storage: It securely stores the real-world data used to train generative AI models.
-
Synthetic Data Storage: HealthLake also acts as a repository for the synthetic data generated by these models. This facilitates easy access and management of both data types within a single platform.
-
Generative AI Model Access: Amazon HealthLake can provide controlled access to generative AI models for healthcare institutions and researchers. This accelerates advancements in the field by enabling a broader community to leverage these powerful tools.
-
Beyond Storage: HealthLake goes beyond just storing data. Its built-in analytics capabilities allow researchers to gain insights from the data, potentially informing the design of generative AI models for specific healthcare challenges.
New Capabilities of Amazon HealthLake for Advanced Imaging Analysis
Amazon HealthLake is taking medical imaging analysis to the next level for traditional methods and the burgeoning field of generative AI. Here's how:
New Capabilities for Advanced Analysis
-
Amazon HealthLake Imaging: This HIPAA-eligible feature simplifies storing, accessing, and analyzing medical images at a petabyte scale. Imagine sub-second retrieval times for medical images within your existing workflows, accessible securely from anywhere. Additionally, HealthLake Imaging allows you to leverage existing medical viewers and analysis applications directly on a single, encrypted copy of the data in the cloud. This streamlines analysis and potentially reduces medical imaging storage costs by up to 40%.
-
Amazon HealthLake Analytics: Harnessing the power of multi-modal data (combining medical images with electronic health records and other sources), HealthLake Analytics unlocks deeper insights. It facilitates querying and deriving insights from this complex data at scale, enabling exploration on both individual and population levels. Secure data sharing across your organization allows for collaborative analysis and empowers the development of advanced analytics and machine learning for medical imaging.
Empowering Generative AI
The latest advancements in Amazon HealthLake, specifically Amazon HealthLake Imaging, further empower generative AI in medical imaging analysis:
-
Standardized Image Management: Amazon HealthLake Imaging facilitates the organization and management of medical images in a standardized format, streamlining data access for generative AI models. This ensures consistency and reduces the need for data pre-processing, a common bottleneck in AI development.
-
Improved Interoperability: It enhances interoperability between different medical imaging systems, allowing for the seamless integration of diverse image data for AI model training. This broader data pool leads to more robust and generalizable generative AI models.
-
Scalability and Performance: Designed for scalability and performance, Amazon HealthLake Imaging efficiently handles large volumes of medical images, enabling the creation of robust generative AI models. This is crucial for training complex AI models requiring vast data.
Challenges and Limitations of Generative AI in Healthcare
Despite its immense potential, generative AI in healthcare has challenges and limitations. Here are some key areas to consider:
-
Data Bias: Generative AI models are only as good as the data they're trained on. If the training data contains biases, these biases can be perpetuated in the synthetic data generated by the model. This could lead to unfair or inaccurate medical decisions, particularly for underrepresented groups.
-
Data Security and Privacy: Generative AI can create highly realistic synthetic data, including medical images or patient records. This raises concerns about data security and privacy. Malicious actors could misuse synthetic data to create deepfakes or commit medical fraud.
-
Explainability and Interpretability: Generative AI models can be complex and difficult to understand. This lack of transparency makes explaining how the model arrived at a particular result challenging. This is especially crucial in healthcare, where decisions can have life-or-death consequences.
-
Regulatory Uncertainty: The regulatory landscape surrounding synthetic data in healthcare is still evolving. As generative AI becomes more widely used, clear regulations need to be established to ensure this technology's ethical and responsible use.
-
Technical Limitations: Generative AI models are still under development, and they can sometimes struggle to capture the full complexity of real-world data. This can lead to the generation of synthetic data that is not entirely realistic or accurate, potentially impacting the reliability of AI-powered healthcare applications.
-
Cost and Infrastructure: Implementing and maintaining generative AI models can be expensive, requiring significant computing power and expertise. This may limit the accessibility of this technology for smaller healthcare institutions.
Future Trends and Innovations in Generative AI for Healthcare
The future of generative AI in healthcare is brimming with exciting possibilities. Here are some trends to watch:
-
Increased Accuracy and Explainability: Generative AI models are constantly evolving, becoming more accurate and interpretable. This will make them even more valuable for healthcare applications.
-
Integration with Electronic Health Records (EHRs): Generative AI will likely become more integrated with EHRs, allowing healthcare professionals to leverage its capabilities directly within their workflow.
-
Focus on Privacy-Preserving Techniques: As generative AI becomes more powerful, there will be a growing emphasis on developing privacy-preserving techniques to protect patient data.
-
New Applications in Genomics and Proteomics: Generative AI has the potential to revolutionize our understanding of genomics and proteomics, leading to breakthroughs in personalized medicine.
-
Wider Adoption in Drug Discovery and Development: Generative AI is poised to play an increasingly important role in drug discovery and development, accelerating the identification and development of new drugs.
Conclusion
The potential of generative AI in healthcare is truly transformative. From accelerating drug discovery to unlocking new possibilities in medical imaging analysis, this technology promises a more efficient, personalized, and effective healthcare system.
Imagine a future where AI models, trained on vast amounts of synthetic data, can design new drugs faster and more accurately. Generative AI can also empower radiologists by automating tasks and aiding in detecting subtle anomalies in medical images. This leads to earlier diagnoses and potentially life-saving interventions.
Personalized medicine is another exciting frontier. Generative AI can analyze a patient's unique data to create custom simulations, predicting disease progression and tailoring treatment plans for optimal outcomes. This shift towards a more patient-centric approach to healthcare can potentially improve the quality of life for millions.
Of course, challenges like data privacy and model interpretability remain. However, as the field of generative AI continues to evolve, we can expect solutions to these challenges to emerge. By harnessing the power of generative AI responsibly, we can unlock a brighter future for healthcare, one where innovation leads to better care for all.
-
Explore the Future Trends of Generative AI in the Education Industry
-
Click here to learn about our Generative AI Consulting Services and Solutions