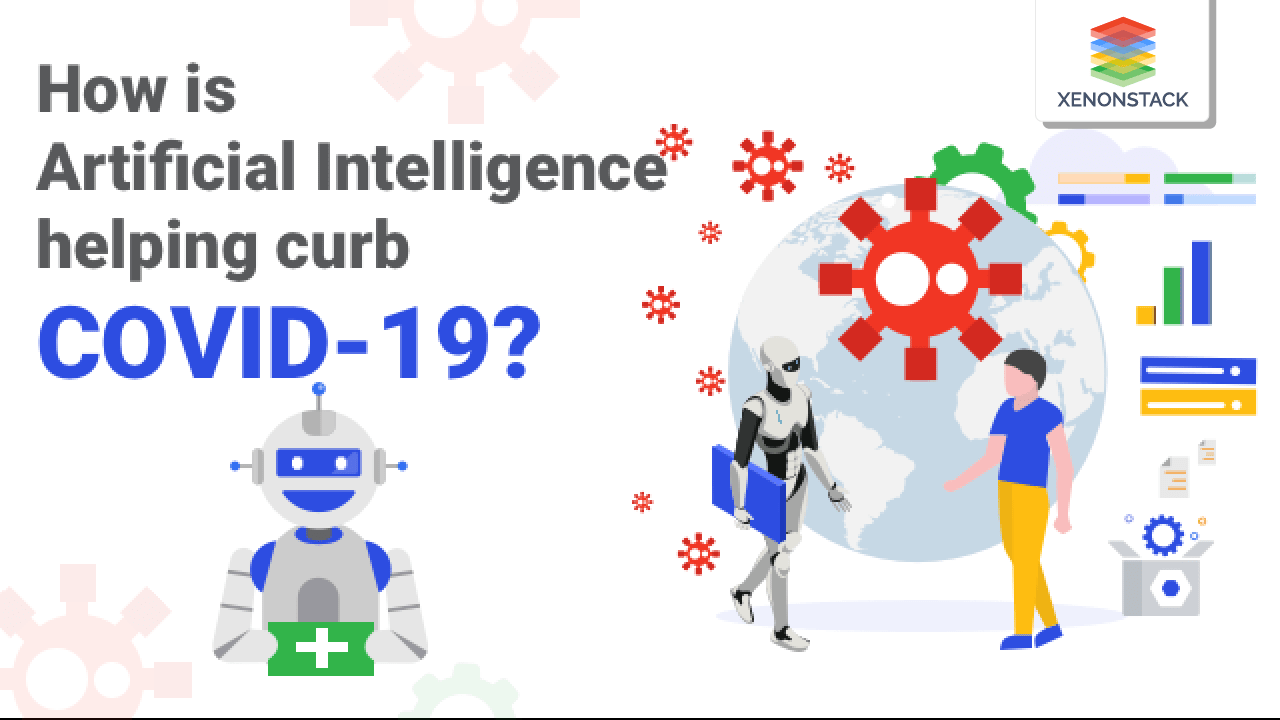
Overview
After a long time, people started their normal life again. They start visiting markets, workplaces, stations, or other public places with minor changes in their lifestyle. The government has defined some rules for the public that they have to follow while visiting public sites to make the condition under control without affecting their running life. But so many people are not following the rules. A little irresponsible behavior of a single person can affect the life of the whole society. It is challenging for the government to monitor each person manually.
Artificial intelligence: A great ally against covid-19
Artificial Intelligence(AI) can help the organization, government, and industries monitor the places and track the people who are not following the rules. The system will generate an alert, and then authorities can take action accordingly and reduce the spread of the virus. This makes it easy for the police to monitor things 24*7.
Such as AI can examine whether the person wears a mask or not, and they are maintaining the required social distance as per the guidelines. When the system recognizes any of the people that are not following the rule, it can alert the authorities. This may make sure that the risk of spreading the virus fairly small.
Akira AI comes with a solution to track and analyze people whether they are following rules or not. It provides the answer to some questions:
- Is the organization taking healthy precautions or not?
- Is there any particular person who is not following the rules?
- What is the particular rule that the person is not following? So that system can track him/her and take action.
Akira AI provides a complete solution to the end customer with its vast features of Explainable AI. "COVID-19 Tracker" system obeys all the Principles of Explainable AI and provides a great customer experience. It provides answers for all user questions by giving the interpretable system that is transparent and easy to understand. Akira AI is using a step by step approach to provide interpretability. Customers can easily understand the working of the system of how the system generates a separate output. By providing transparency to black-box models, Akira AI helps to gain user trust and confidence. It includes a performance with explainability. For the "COVID-19 Tracker" system, Akira AI uses an opaque model to gain better performance and accuracy than the transparent model. But also provide explainability to solve all the user queries.
End Customer Perspective
It is a human tendency that whenever we use machines, we ask for it working on how it works only then we can believe in it. Numerous questions come into the customer's mind when he/she uses AI systems. But it becomes difficult for the system to answer all these questions when the model is opaque. Some of these questions are:
- Which modal should be picked?
- Which modal is selected, and why is that selected? Is it offering the best trade-off in terms of accuracy vs. explainability? Is it possible to enhance modal explainability without damaging performance?
- Is this system transparent or opaque?
- How does the system predict an individual output?
- How does output vary by changing value?
- What is the threshold of distance that needs to be maintained to obey rules?
- What Mr. Jain has to do so that he could follow the rules, and thus the system also recognized him under the category who are following rules?
- How do they handle raw data, and does its absence affect the prediction or not?
- What rules model follow to alert that Miss Mendala is not following the rules?
"How to take your business back to better, not just back to normal" - E&Y Company
Solution
Explainable AI helps to answer all the questions of the customer and provide a transparent and interpretable system. Akira AI provides Explainable AI that is using various frameworks and methodologies to answer those questions. There is a list of methods that can be used to answer this question. These are given in the following table:
Implementation
To implement those methodologies, we have various packages and libraries. These libraries help to provide an answer to the customer by implementing the methodologies.
Pillars of Explainable AI
There are seven pillars based on Explainable AI that can help the government and industries track a person's irresponsible behavior. Below listed are the critical 7 AI pillars in brief:
Transparency: It provides complete transparency of the model, algorithm, and features used for prediction.
Example: What is the likelihood Mr. Jain has obeyed all the rules and taken COVID-19 precaution?
The system says that Mr. Jain is not following the rules because he is not wearing the mask. The interpretation of the system that provides using visualization.
- Transparency of the system can vary according to the user. To provide transparency of the model's formulas and inner working to the end customer who is not a technical person is not of any use because he would not understand that.
- Transparency can be different for different people.
- Transparency of the model output can be useful for the end-user. A simple rule on which system takes decisions can also be helpful for the end-user. But if we talk about the developers, then transparency will be different. Here we can use the technical terms and model formulas to explain the working of the model.
- Various approaches can provide transparency; they can be model-specific or model agnostic, such as LIME, SHAP, in Trees.
Domain Sense: The explanation that the system provides makes sense in the application's domain and easy to understand for the user that will use it. It is of no worth if the user is not able to understand it. Therefore, Akira AI provides an explanation using visualization so that end-user can easily interpret it.
Consistency: Explanation should be consistent with any number of runs. It should not be changed when values are the same for each run of the code. An explanation will not change with any number of the run. It will always give the same result.
Parsimony: The explanation that the system provides should be straightforward. As there is a question of the end-user, what is the general rule that the system follows? The model provides its output in the simple text that easy to understand as if
Wear Mask= YES ‘AND’ Social distance >=100cm
It means a person is following the rules.
But it is not necessary to always provide the simplest explanation. Sometimes, it may be possible that there are various features if we use the same method(text). Also, then it becomes difficult for the user to understand that.
Generalizability: Explanation of the AI system should be generalized.
Example: The system decides that Mr. Jain is not following rules and explains it using features because he does not wear the mask. This explanation is not general for people's whole organization as some are detected because they do not maintain social distance. There are some stages of generalizability:
Local Model Generalizability: Instance level generalizability is known as local generalizability—examples: LIME, SHAP, etc.
Global Model Generalizability: Model-level generalizability is known as Model Generalizability. —examples: decision trees, rule-based model
Cohort level model: This is a type of global model. Here the explanation is generated at the level of the cohort.
Trust/Performance: With the model's trust and explainability, the model should also perform well.
As Figure depicts that with increasing explainability level of model accuracy decreases, that is not acceptable. Therefore it is vital to choose the model that should provide accuracy with explainability because we cannot compromise its accuracy.
In this use case, we use the Random forest algorithm that provides good accuracy and explainability. We use various methodologies that are already discussed.
- We can precisely choose the model that can balance user trust and model performance.
- There are various terms based on which we can check model performance, such as AUC, ROC, Gini NOrm, Recall, Precision, etc.
- We can choose the model that provides us with good accuracy, and for an explanation, we can use Explainable AI frameworks. It will help to balance both the explainability and accuracy of the model.
Fidelity: It is necessary to have alignment between model and explanation.
- If it is not, then there can be chances of incorrect explanation. There can be a lack of consistency and non-determinism.
- This can be due to problems in data.
- Explanations should align with the predictive model as close as possible.
- Ante-Hoc: The prediction model and explanation model is the same.
- Post-Hoc Models: Here prediction model and explanation model are different.
- Special Case: There can be mimic models.
Conclusion
Explainable AI is an excellent approach to gain customer's trust and confidence. It makes the system more trustable and interpretable. With this approach, we can make our system more productive by tracking performance, fairness, errors, data, etc.