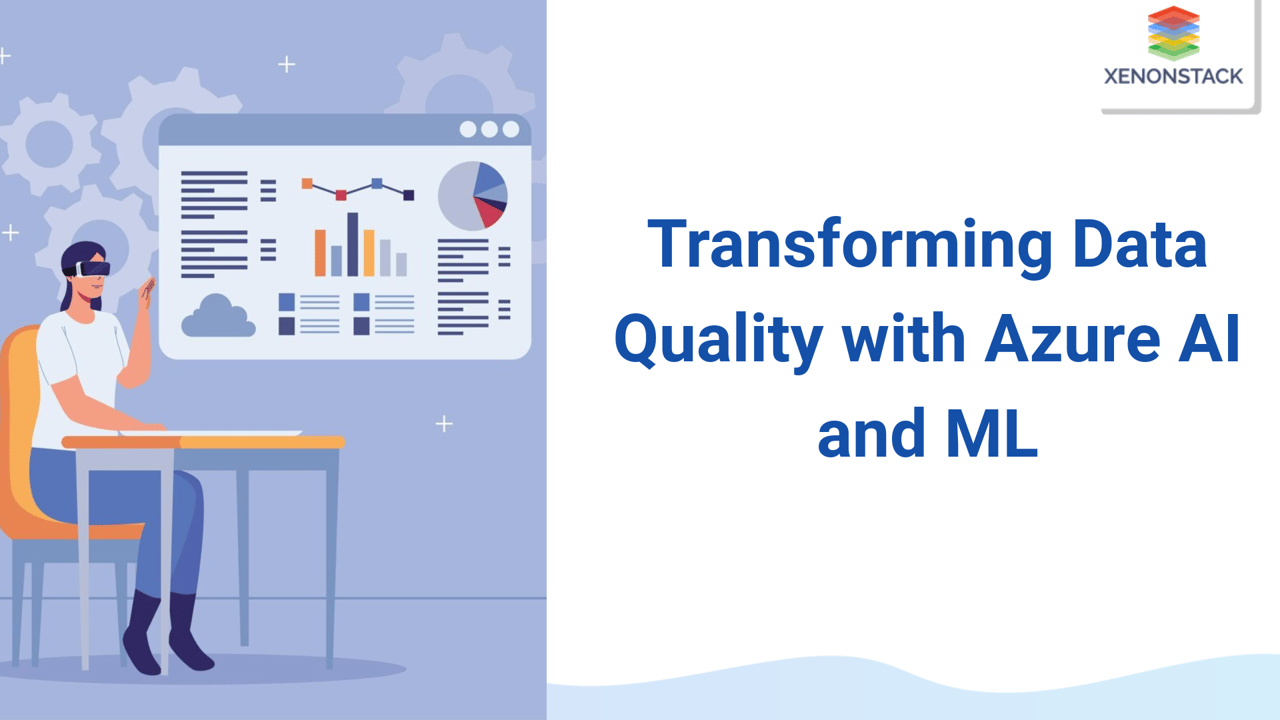
Data creates every decision, so data quality standards must be kept high. Azure Machine Learning not only eases the process of AI models’ development and deployment but also strongly contributes to data integrity. AI is changing how data is checked for quality and how its accuracy, completeness, and reliability are continuously monitored by automating quality checks.
Data is at the core of decision-making, and high-quality data is essential for any successful analytics or AI initiative. Maintaining data integrity is about avoiding errors and building trust in the insights generated.
The Intersection of AI and Data Quality
Key metrics of accuracy, completeness, consistency, uniqueness, and timeliness measure data quality. Unfortunately, all these factors directly influence the model's performance in machine learning. But that includes such faulty insights and poor decision-making. On the other hand, automated quality assessments like null value rate, data type error rate, and out-of-bound error rate can be corrected, and organizations can take a proactive approach to handling these issues with quality.
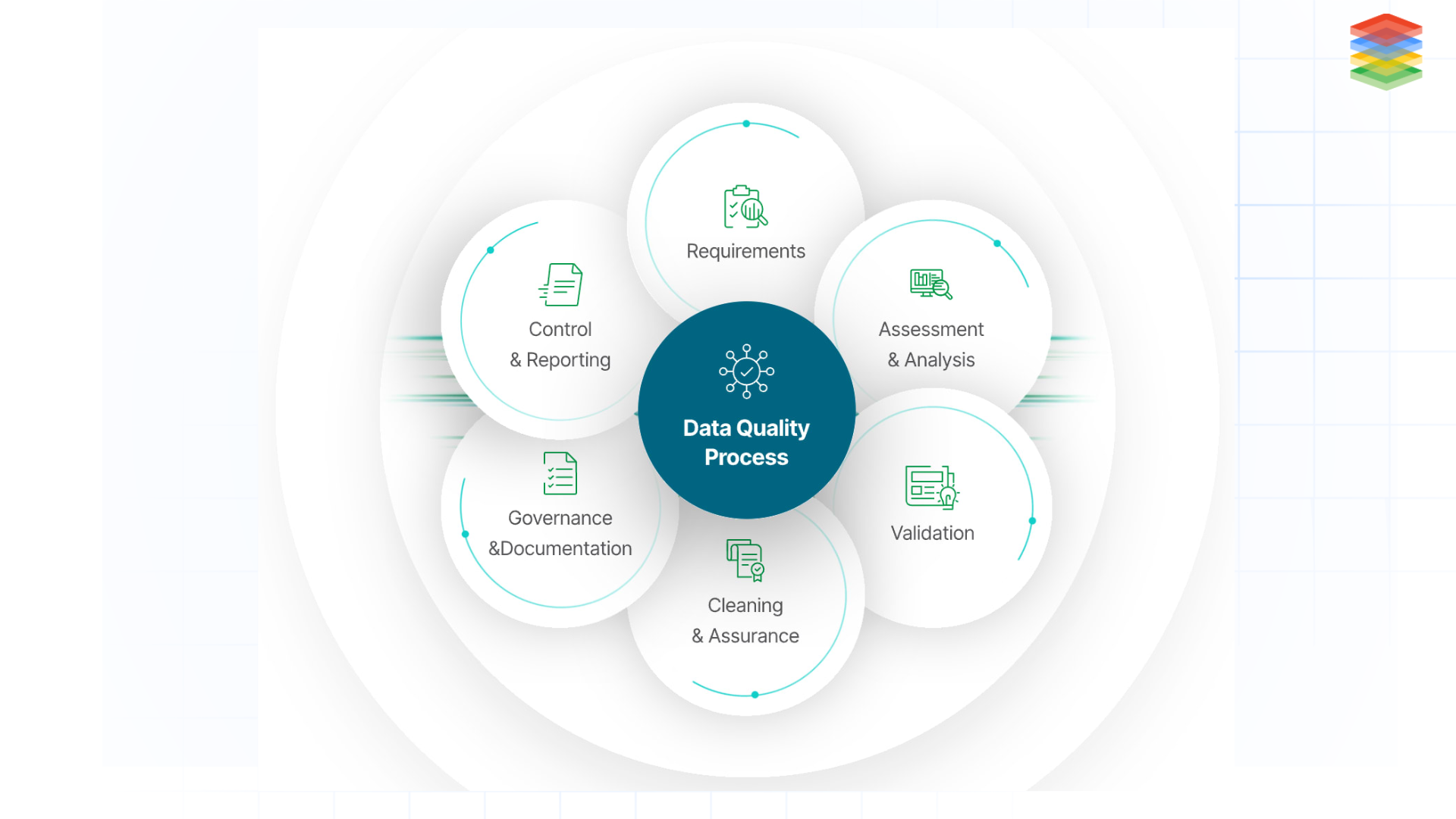
Key Data Quality Metrics
-
Accuracy and Completeness
-
Consistency and Uniqueness
-
Timeliness
Azure Machine Learning: A Catalyst for Data Integrity
Azure Machine Learning serves organizations with an enterprise-grade system that assists through every step of machine learning operations, including data intake, model deployment, and continuous model surveillance. Its core features include:
-
Accelerated Model Development and Data Preparation: The organization can achieve accelerated model development through rapid data preparation procedures and reusable features stored in central repositories.
-
Automated Processes with AutoML: AutoML minimizes human error and creates better models from high-quality data through automated processes that reduce manual training procedures.
-
Continuous Production Monitoring: Production monitoring throughout the system enables ongoing data quality indicator surveillance. This includes observing schema alterations, the appearance of null data, and outlier events that produce alerts through threshold crossings. The capabilities produce faster value delivery and maintain an ongoing feedback process that secures accurate data for decision-making.
The Role of AI in Ensuring Data Quality and Integrity
AI takes care of various critical tasks in data quality management by automating key processes:
-
Anomaly Detection
Machine learning algorithms function as anomaly detectors, flagging deviations from standard data patterns such as missing values and type mismatches—issues that might otherwise be overlooked. Azure Serverless Computing.
-
Predictive Remediation
Using historical data, AI can forecast potential data quality issues before they become problematic, thus facilitating proactive remediation.
-
Automated Data Cleansing
Azure Data Factory or Synapse Analytics integrates with AI-driven capabilities to deduplicate, normalize, and enrich datasets, ensuring that only high-quality data is fed into analytics and decision systems. This shift from reactive fixes to proactive quality management makes AI central to a sustainable data governance strategy.
Challenges in Data Quality Management
Organizations often struggle with challenges such as data silos and rapidly changing data formats, which can hinder their ability to scale operations. To address these issues:
-
Centralized Data Governance
Utilize Azure Purview and Synapse Analytics as centralized platforms to generate a unified overview of data assets across the enterprise.
-
Automated Schema Validation
Ensure that when data pipelines require adjustments for schema changes, schema validation runs automatically, and pipelines evolve to support these modifications.
-
Scalable Data Quality Processes
Design data quality processes to scale with changes in data volume and complexity. Azure’s cloud-native services provide pipelines with the capacity to manage data expansion while maintaining optimal performance levels.
Integration within the Azure Ecosystem
The Azure ecosystem offers a complete set of tools that cover all aspects of data quality improvement:
Data Cataloging and GovernanceAzure Purview delivers data cataloging, lineage tracking, and automated classification to enforce governance. Orchestration with Data FactoryAzure Data Factory orchestrates data pipelines that execute ETL processes alongside quality control systems. Large-Scale Data ValidationAzure Synapse Analytics offers powerful querying and data validation capabilities essential for maintaining large-scale data consistency. Real-Time MonitoringThrough Azure Monitor, organizations can track the real-time status of their pipelines and quality irregularities, enabling quick issue resolution. Integrating Azure Machine Learning with these tools ensures that all stages—from data ingestion to model deployment—adhere to strict data quality requirements.
Beyond Azure: Integrating Third-Party Tools for Comprehensive Data Quality
While the Azure native ecosystem is robust, many enterprises extend their capabilities with third-party tools:
Enhancing Data Testing and Validation
Great Expectations and Debt: Improve complex modern data systems' data testing and validation capabilities.
-
Apache Griffin: Offers additional real-time data quality monitoring capabilities.
-
Informatica and Talend: Provide advanced data cleansing and integration features for specific needs.
Organizations can customize their data quality strategies by integrating these third-party tools to fill functional gaps within Azure’s native functionalities.
The Business Impact of AI-Enhanced Data Integrity
Implementing AI to improve data quality delivers specific advantages to businesses:
Operational Efficiency and Cost Reduction
-
Efficiency Gains: Resource reduction enables strategic project development through decreased manual data cleansing operations.
-
Cost Savings: High-quality data minimizes the need for compliance penalty reimbursements and reprocessing expenses.
Enhanced Decision Making
-
Reliable analytics drive better business strategies, and consistent, accurate data improves customer experience by ensuring a seamless interaction flow.
-
Industries such as finance, healthcare, and retail are already witnessing business expansion and competitive edge through these improvements.
Future Trends: The Evolution of AI-Driven Data Integrity
Data quality management continues to evolve as a critical element of modern business expansion:
-
Autonomous AI Systems
AI systems are increasingly assessing newly obtained IoT data in real-time and autonomously fixing data issues without human supervision.
-
Ethical AI and Data Governance
Future systems will likely implement ethical controls for AI text handling to ensure transparency and ethical data treatment. As organizations expand their cloud strategies, they must ensure that data quality systems effectively integrate with all platforms in their IT environment.
Conclusion: Transforming Data Integrity with Azure Machine Learning
Azure Machine Learning is an AI modelling service and a complete data quality management system. By deploying Azure data tools for quality monitoring, organizations can turn raw data into valuable business insights.
As data volumes increase and data quality rules tighten, AI systems will increasingly be responsible for data quality. Enabled by Azure best practices and integrated tools, the implementation will deliver enhanced outcomes, delivering secure, high-quality data that is the basis for reliable decision-making.
Next Steps with Azure ML & AI
Talk to our experts about implementing Azure ML & AI solutions to ensure data quality and integrity. Discover how industries and various departments use these technologies to become decision-centric, leveraging AI to automate and optimize IT support and operations, enhancing efficiency and responsiveness.