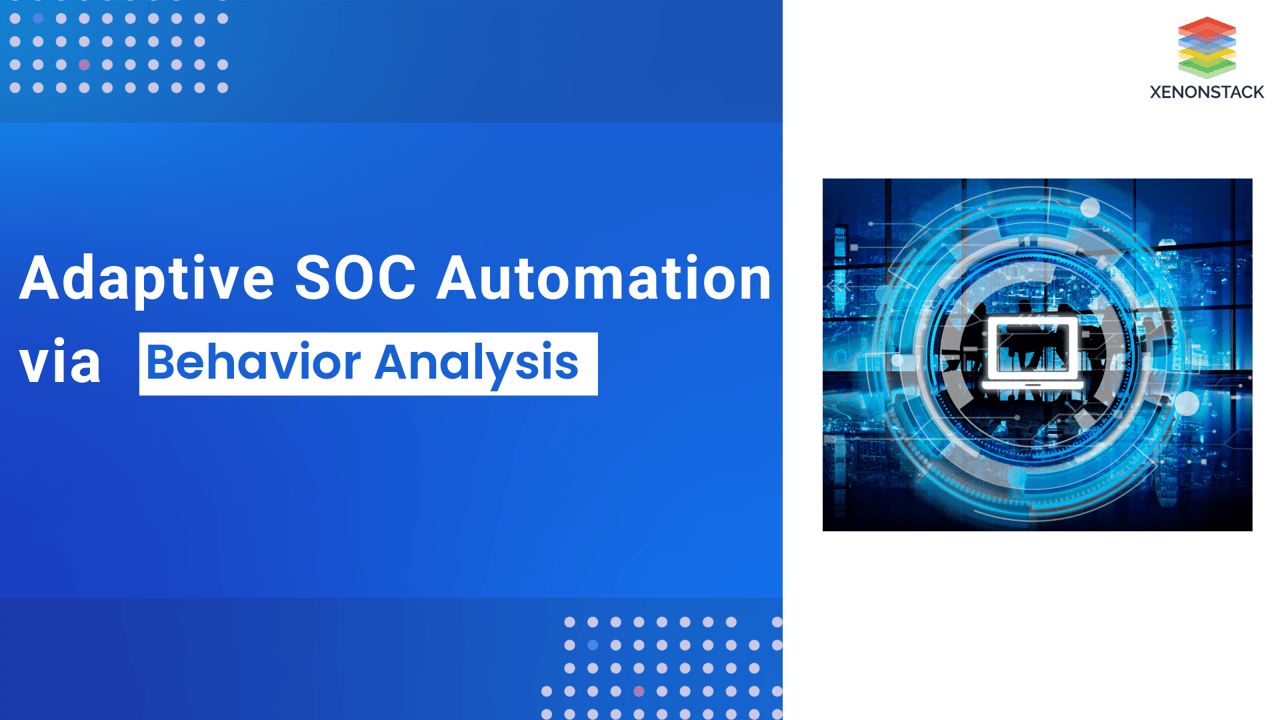
Behavioural analytics for automating SOC is a revolutionary concept that uses UEBA to strengthen SOCs in terms of velocity, effectiveness, and accuracy. It is integrated with machine learning technologies that perform analysis of User and Device Activities and detect unfamiliar performed patterns, which can pose security risks. SOC automation incorporating UEBA as an added feature can help organizations achieve a preventive security approach that not only eliminates threats but also enables a quicker and more efficient approach to threats.
Understanding the Role of UEBA
UEBA helps SOC automate its processes by analyzing users and device activity in a network space. The UEBA discipline was first introduced by Gartner in 2015 as an evolution of the UBA concept to include other objects like servers, routers, IoT devices, etc. Where UBA mainly concentrates on the actions dedicated to the user activity, UEBA is an enhanced security approach that can help detect sophisticated threats to the internal user, for instance, insider threats or stolen credentials, which are smart enough to bypass the basic security system of an organization.
As a result of analyzing various patterns within the standard activity, UEBA reveals threats unnoticed by typical security solutions. It is mostly integrated with other enterprise security solutions like Security Information and Event Management (SIEM), Endpoint Detection & Response (EDR), Extended Detection & Response (XDR), and Identity & Access Management (IAM), enhancing its behavioural intelligence to SOC solutions.
Types & Features in Cybersecurity
Depending on the aspects of an organization, behavioural analytics can be divided into several types. Here are the most common types of behavioural analytics used in organizations today:
User and Entity Behavior Analytics (UEBA)
With UEBA, attention is paid to users and entities, including devices like routers, servers, applications, and others, to identify abnormalities in their behaviour. Such systems keep track of pre-existing user accounts, devices, and applications, inspect their usage patterns, and notify when they show signs of being part of the attack.
Examples of malicious user and entity behaviours include:
-
An account login session that comes from an anonymous IP address, a different operating system, a different web browser, or a country different from the usual could be a sign of account compromise by a bad actor. Consecutive logins for an account, together with multiple failed login attempts for a user who has never failed before, are also indicative of unauthorized access attempts.
-
This might be observed when a user who has no permission attempts to read files, directories or resources in the account of a privileged user; this is an indication of privileged account abuse.
-
An authorized user who uses the computer normally begins downloading large, uncharacteristic files that are often an indicator that he/she is downloading malware.
-
A user unusually transfers huge amounts of data and may leak data from the system.
-
A user enters nonstandard instructions or invokes scripts not usually associated with their position, for instance, a marketer using the SQL language to search for a database.
-
An application receives traffic that is thousands of times higher than normal outside the official utilization time, which indicates a DDoS attack.
The behaviour of networks is analyzed in the context of network behaviour analytics (NBA), where the focus is to identify unusual traffic and traffic that is headed toward known malicious websites. The most common malicious network traffic behaviours include:
Network Behavior Analytics (NBA)
Abnormal operation with Non-Standard applications such as HTTP, SMTP or FTP.
When many users access or transit through a domain name or an IP address, that is of a dubious nature.
For example, a user who endeavours to map or scan the network topology shows that the network has an intruder seeking weaknesses to exploit.
Horizontal traffic within the network may indicate that a compromised user or the involved system is searching for more access.
Opening/Downloading scripts or folders containing.exe files from unknown sources.
Communicating an excessively large amount of information to other systems or from the network.
How Behavioral Analytics Transforms SOC Automation
Behavioural analysis enhances SOC automation since it enables threat prediction. One of the major challenges of SOC teams is that they are flooded with alerts, which makes it difficult to pay attention to the most important threats. Behavioural analytics solves this problem by sifting through huge amounts of user and entity data, selecting only those abnormal activities.
Key benefits include:
-
Reduced false positives: By focusing on behavioural deviations, UEBA reduces the number of unnecessary alerts that give the SOC analysts real threats to work on.
-
Improved response times: High-speed data analysis and immediate risk scoring allow quick response to threats.
-
Enhanced zero-trust security: Behavioral analytics complement current approaches to zero-trust security architectures, which require constant validation of all users and entities.
Key Components for SOC
For successful SOC automation with behavioural analytics, the least requires data sources, machine learning models, and scoring mechanisms. Key components include:
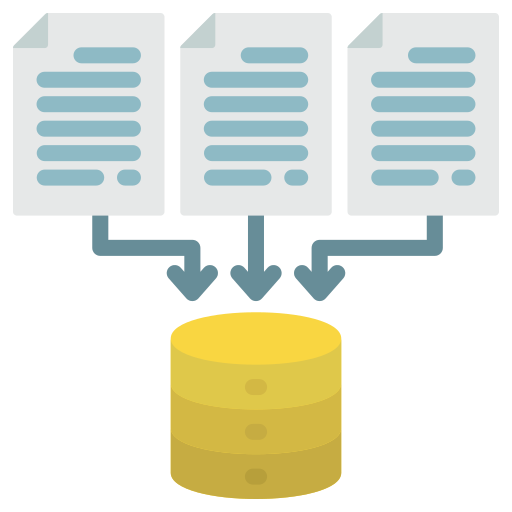
Data Collection and Integration
UEBA uses data from various sources to build a big picture of the network's activity. These sources include Network devices such as firewalls and VPNs, security tools such as antivirus software, EDR, and SIEM, and identity and access management (IAM) databases such as Active Directory.
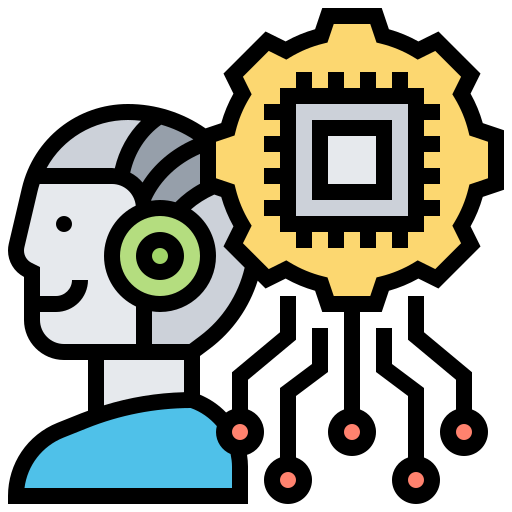
Machine Learning and Baseline Modeling
UEBA uses machine learning to calculate baseline behaviour models by analysing users’ activity data. This sets a general reference that can be used to determine any variation.
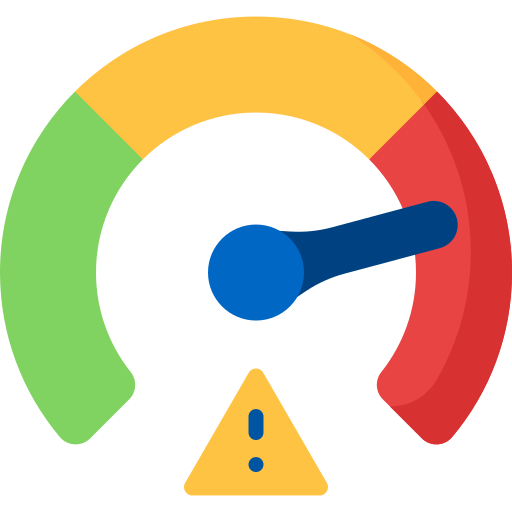
Risk Scoring and Alerting
UEBA provides risk scores for anomalous behaviour. A low-risk score suggests that the issue differs slightly from normalcy, while a high-risk score suggests the opposite. The risk score acts as an alert list that guides the SOC analyst on what actions to take.
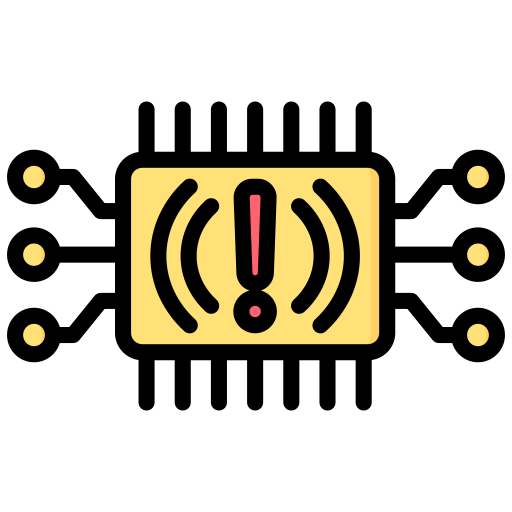
Threat Intelligence Integration
External threat intelligence feeds are known to be useful and can be integrated into UEBA systems to boost the recognition of identified threats, including those presented in MITRE ATTACK.
Architectural Diagrams and Flow
The architectural diagrams demonstrate how behavioural analytics integrate with SOC systems to automate threat detection and response.
Behavioral Analytics in SOC Architecture
This architectural information shows that UEBA extracts data from network equipment, security tools, and databases and transforms it into behavioural profiles.
Figure 1: Behavioral Analytics in SOC Architecture
-
Data Collection Layer: Originals like firewalls, VPNs, authentication logs, etc.
-
Processing and Analytics Layer: Baselines and real-time anomaly detection models using learning algorithms.
-
Alert and Response Layer: Risk score on specific alerts for SOC teams.
Workflow for UEBA in SOC Automation
The following diagram describes UEBA's SOC automation and shows how its workflow functions from data ingestion to anomaly detection and, finally, threat alerting.
Fig 2: Workflow for UEBA
-
Data Ingestion: Information in any format from different resources may be acquired and analyzed.
-
Behavioural Baseline Modeling: UEBA utilizes machine learning to model typical user and device behaviours in the environment.
-
Real-Time Analysis: Information is checked and compared to the baseline to determine whether there are current abnormalities.
-
Risk Scoring and Alerting: Anomalies are given a score, and high-risk events are passed on to the SOC.
Strategic Benefits and Use Cases
UEBA also enriches tactical and strategic Security Operations Centre SOC-level security regarding detection and compliance. Key use cases include:
-
Insider Threat Detection: UEBA assists in identifying cybercriminals, often insiders who exploit their privileged accounts for ill intent.
-
Compromised Credential Detection: Criminals who exploit stolen credentials make themselves hard to detect since they use valid access credentials. UEBA makes alerts on their activities noticeable, which will prevent data breaches.
-
IoT Device Security: In industries with high IoT device usage, for example, healthcare UEBA detects potentially infected IoT devices that can be leveraged to provide unauthorized access to the networks.
-
Data Exfiltration Prevention: UEBA shows signs of data theft, such as changes in the rate of data access or downloads, which may help alert security personnel of an upcoming data theft incident.
-
Compliance with GDPR and Other Regulations: For compliance, UEBA assists in checking user access to sensitive information and guarantees the implementation of data protection standards.
Conclusion
In turn, behavioural analytics is critically important for creating a proactive and robust SOC regime. When UEBA is implemented UEBA as a part of SOC automation, these organizations will detect insider threats, detect compromised credentials, and prevent data breaches. With modern security tools such as SIEM, EDR, and IAM, behavioural analytics provide SOC teams with valuable information on the network’s activity and facilitate the adoption of a zero-trust security model. The application of SOC automation will progress, and with the help of behavioural analytics, the primary objective of threat detection and response will be more accurate and efficient.
Discover More Setting up Security Operations using Azure Sentinel