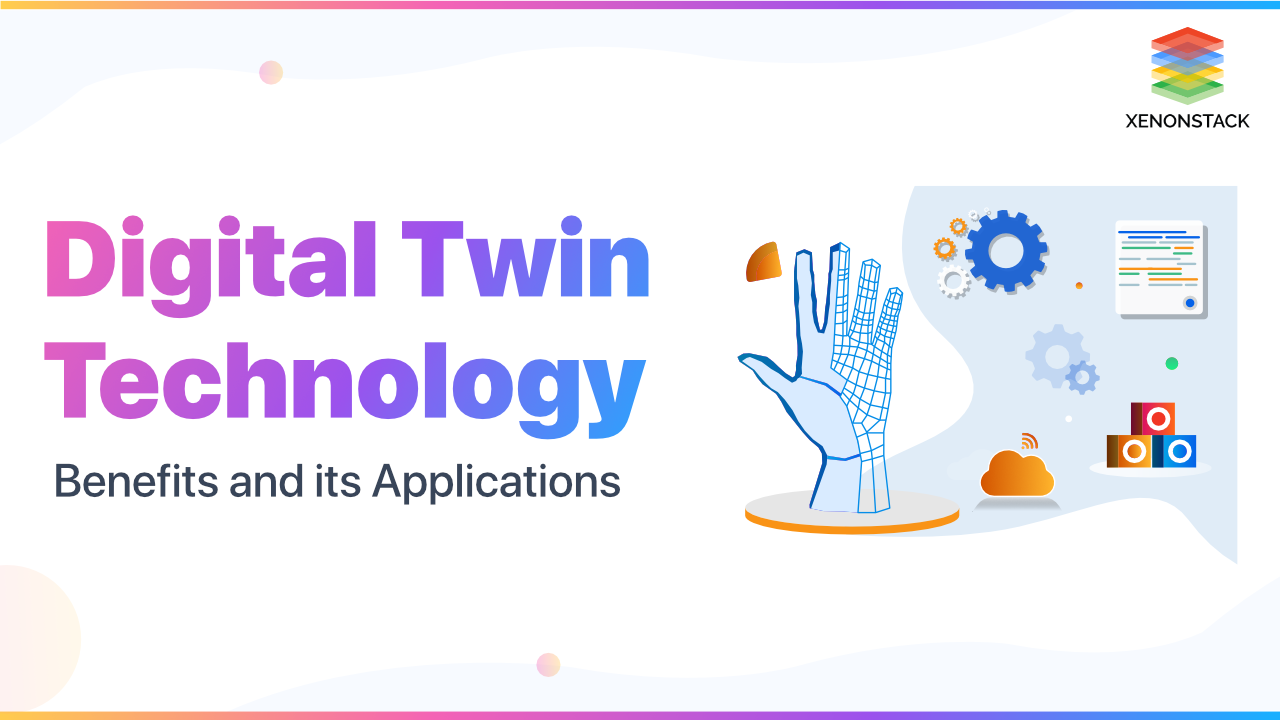
Digital transformation has touched virtually every aspect of our lives. We've witnessed books evolve into e-readers, music transition from physical media to streaming services, and photographs move from albums to cloud storage. While these examples represent digitization in its simplest form, Digital Twin Technology takes this concept to an entirely new level.
This article explores the fascinating world of Digital Twin Technology, unpacking how this revolutionary approach creates virtual replicas that do far more than simply exist in digital space—they evolve, predict, and provide unprecedented insights into their physical counterparts.
What is Digital Twin Technology?
Digital Twin Technology creates dynamic virtual replicas of physical assets, processes, and systems that mirror real-world behavior in real-time. Far beyond static 3D models or blueprints, Digital Twins leverage IoT sensor data to create intelligent, evolving simulations that transform how organizations visualize, analyze, and optimize their operations.
Understanding Digital Twin Technology in the IoT Ecosystem
Digital Twin solutions have emerged as a cornerstone of Industry 4.0, with Gartner consistently featuring Digital Twin implementation among its "Top 10 Strategic Technology Trends" for multiple years. These sophisticated virtual models utilize IoT-connected sensors attached to physical objects to continuously collect and transmit operational data, creating a bidirectional relationship between physical assets and their digital counterparts.
The core value proposition of Digital Twin Technology centers on its ability to build, test, and refine equipment and processes in a virtual environment before deploying changes to physical systems, significantly reducing risks and costs associated with operational modifications.
Data: The Cornerstone of Digital Twins
At its core, Digital Twin technology rests on one critical foundation—data. While implementation appears straightforward, accessing quality data across industrial ecosystems remains the primary challenge. Success demands strategic data management and systematic extraction from physical systems, transforming decision-making from speculation to factual certainty. When data flows freely, Digital Twins deliver their transformative promise; when data falters, the entire construct crumbles.
Evolution of Digital Twin Technology
Though formally named in 2010, digital twin concepts have deeper historical roots. In 1991, David Gelernter laid theoretical groundwork with his publication "Mirror Worlds," exploring virtual replicas of physical reality. The practical application to manufacturing emerged in 2002 when Dr. Michael Grieves (then at the University of Michigan) formalized the digital twin software concept as a manufacturing methodology.
The term "digital twin" itself wasn't coined until 2010, when NASA's John Vickers introduced it to the technological lexicon. Interestingly, NASA had already been implementing the core principles decades earlier. During the space exploration missions of the 1960s, NASA created exact earthbound duplicates of each spacecraft in orbit. These physical replicas served as simulation environments where flight crews could study, troubleshoot, and test scenarios—essentially functioning as early, physical manifestations of what we now recognize as digital twin technology.
What are the benefits of enabling Digital Twin technology?
Digital twins deliver numerous strategic advantages across industries. Here are the key benefits they offer:
-
Enhanced Performance - Digital twins provide real-time insights that optimize equipment, plant, and facility performance. By addressing issues as they emerge rather than after they escalate, organizations maintain peak operational efficiency and minimize costly downtime.
-
Predictive Maintenance - With comprehensive digital visualization of your entire operation—from manufacturing plants to commercial buildings—digital twins leverage smart sensor data to monitor each component's performance. This enables proactive intervention at the first sign of irregularities, preventing full equipment failures and extending asset lifespans.
-
Remote Operations - The virtual nature of digital twins enables secure remote monitoring and control of facilities, reducing on-site staffing requirements. This capability proves particularly valuable when overseeing hazardous industrial environments, enhancing both operational flexibility and workplace safety.
-
Accelerated Development - By creating virtual replicas before physical construction begins, digital twins dramatically compress product and facility development timelines. Organizations can simulate various scenarios, test responses to potential failures, and implement design improvements—all before committing resources to actual production.
Types of Digital Twin
There are different digital twin types, which can often run side by side within the same system. While some digital twins replicate only single parts of an object, they're all critical in providing a virtual representation. The most common types of digital twins are the following.
-
Component Twins - Virtual replicas of individual elements (like motors or sensors) within a larger system, enabling detailed monitoring of specific parts.
-
Asset Twins -Digital models of multiple components working together as a single entity, showing interactions and generating performance data to inform maintenance decisions.
-
System Twins- Higher-level simulations showing how various assets operate together, providing visibility into system-wide performance and identifying optimization opportunities.
-
Process Twins - Comprehensive digital replicas of entire operational ecosystems, integrating all components, assets, and systems to visualize complete workflows and enable enterprise-wide improvements.
How Digital Twin Work?
Digital twins create virtual replicas of physical assets, accurately reproducing their functionality, characteristics, and behavioral patterns. These dynamic digital counterparts are developed using data collected by smart sensors embedded within the physical asset. The resulting virtual model serves throughout the asset's entire lifecycle—from initial testing and development through operational deployment and eventual decommissioning.
The functionality of digital twin technology relies on several key technological pillars:
Internet of Things (IoT)
Digital twins depend fundamentally on IoT infrastructure—networks of connected devices that enable seamless communication between physical objects, cloud systems, and other devices. The proliferation of affordable computing chips and high-bandwidth telecommunications has created an ecosystem of billions of internet-connected devices.
These IoT sensors continuously transmit operational data from physical assets to their digital counterparts. The information flows into specialized software platforms or analytics dashboards that visualize and process the data in real-time, creating a living digital representation that evolves alongside its physical twin.
IoT is the backbone of Digital Twins, enabling real-time data flow between physical assets and their digital replicas.
Artificial Intelligence and Machine Learning
Artificial Intelligence represents the branch of computer science focused on developing systems capable of performing tasks that typically require human intelligence—including learning, reasoning, and pattern recognition. Machine learning, a subset of AI, creates statistical models and algorithms that enable computer systems to improve through experience without explicit programming.
Within digital twin ecosystems, machine learning algorithms process vast quantities of sensor data to identify meaningful patterns and generate actionable insights. These AI/ML capabilities provide critical intelligence regarding performance optimization, predictive maintenance requirements, emissions monitoring, and operational efficiencies.
Digital Twins vs. Simulations
While both digital twins and simulations create virtual model-based representations, important distinctions exist between these technologies:
Simulations typically serve design purposes and offline optimization scenarios. Engineers introduce variables to simulations to observe hypothetical outcomes in controlled environments. These models operate independently from physical assets and represent static scenarios.
Digital twins, by contrast, create complex, interactive virtual environments that update continuously based on real-time data from physical counterparts. They offer broader applications and greater sophistication than traditional simulations.
Consider automotive applications: A driving simulation provides immersive training experiences and scenario-based learning without connecting to an actual vehicle. A digital twin, however, maintains a persistent link with its physical counterpart, incorporating comprehensive vehicle data—performance metrics, maintenance history, component replacements, sensor-detected issues, service records, and real-time operational status.
IoT has a significant impact on the Industrial sector too. Several industries are accepting this change to increase productivity and efficiency. Click to explore about, IoT Data Analytics for Intelligent Maintenance
What are the challenges of adopting Digital Twin?
-
3D Modeling Requirements: A fundamental prerequisite for creating effective digital twins is comprehensive 3D modeling. Despite industry surveys indicating that many users consider 2D illustrations adequate, developing functional digital twins is impossible with only 2D drawings. This presents a significant barrier as numerous industrial sectors still predominantly operate using 2D technical documentation.
-
Digitization Gaps: The success of digital twin implementation depends heavily on complete digitization across the value chain. While larger organizations may have adopted digital technologies, many small-scale suppliers lack the awareness, resources, or capabilities to embrace digital transformation. This creates disconnects in the data ecosystem necessary for comprehensive digital twin functionality.
-
Data Management Complexity: Unlocking the full potential of digital twins requires a holistic approach to storing, managing, and processing product data throughout its lifecycle. Organizations must establish robust engineering change management processes to ensure perfect synchronization between physical assets and their digital counterparts. Without this alignment, digital twins cannot deliver their promised value.
Discover how Digital Twin simulations and AI agents are transforming manufacturing by optimizing operations, enhancing predictive maintenance, and improving efficiency. Read the full blog on Akira AI
What are the Use Cases of Digital Twin?
With the existence of Industry 4.0, connecting things or digitization is well recognized in every business. The development of IoT results in the representation of physical objects digitally. The concept predicts the present as well as the future of the product or thing. It reduces operational costs, increases productivity, efficient production cost, performance improvement, and predicts behaviour. The primary application area of the technology is as follows -
-
Manufacturing - Digital Twin had a significant impact on designing and manufacturing the product. It changes the old process of directly producing the product after getting the information.
-
Space Research - During its first days in space, NASA had to face lots of hurdles. One significant way to diagnose and repair the craft in the area was to create a Digital Twin of the spacecraft where all information was stored, and they simulated the conditions. That way, they solved their problems.
-
Automobile - Digital Twins can also be designed to create virtual models of vehicles before their actual construction. This helps capture the car's behaviour when it is working. We can get periodic feedback about performance and maintenance requirements. Thus, it helps deliver the best facility to the customer.
-
Wind turbines - General Electric(GE) uses the digital twin to monitor and control turbines on its wind farms. They resolve the problem when it generates some updation about turbines.
-
Healthcare - Digital Twin provides healthcare by analyzing reports and patient history. This makes it easy for doctors to give proper medication to their clients' digital twin healthcare.
-
Industries IoT - Industries are now widely using Digital twins to check the position of their complicated machinery. This property of predictive maintenance helps predict future operations and anomalies.
-
Retail - Appealing to consumer experience is critical in the retail sector. Implementing digital twins can play a crucial role in augmenting the retail customer experience by creating virtual twins for customers and modelling their fashions. It also helps in better in-store planning, security implementation, and energy management.
How can Xenonstack help with digital twin technology?
Xenonstack's Digital Twin Platform enables organizations to create comprehensive virtual replicas of physical assets, processes, and systems to optimize operations and drive innovation. Our end-to-end solution combines IoT integration, advanced analytics, and visualization capabilities to deliver intelligent digital twins that evolve with your business.
Xenonstack's expertise helps you seamlessly implement digital twin technology across your enterprise, from initial concept to full-scale deployment. With Xenonstack's Digital Twin solutions, you can do the following:
-
Accelerate digital transformation initiatives with scalable, enterprise-ready twin implementations.
-
Enhance operational visibility through real-time monitoring and predictive analytics.
-
Optimize asset performance by identifying inefficiencies and implementing data-driven improvements.
-
Reduce maintenance costs through condition-based monitoring and preventive maintenance scheduling.
-
Simulate process changes in virtual environments before implementing in physical systems.
-
Enable remote operations management with comprehensive digital representations accessible from anywhere.
Next Steps for Implementing Digital Twin Technology
Talk to our experts about implementing Digital Twin technology and discover how industries and various departments leverage virtual replicas to enhance decision-making and operational efficiency. Digital Twins integrate AI, real-time data, and predictive analytics to optimize IT support, streamline workflows, and improve responsiveness, driving smarter and more proactive business strategies.