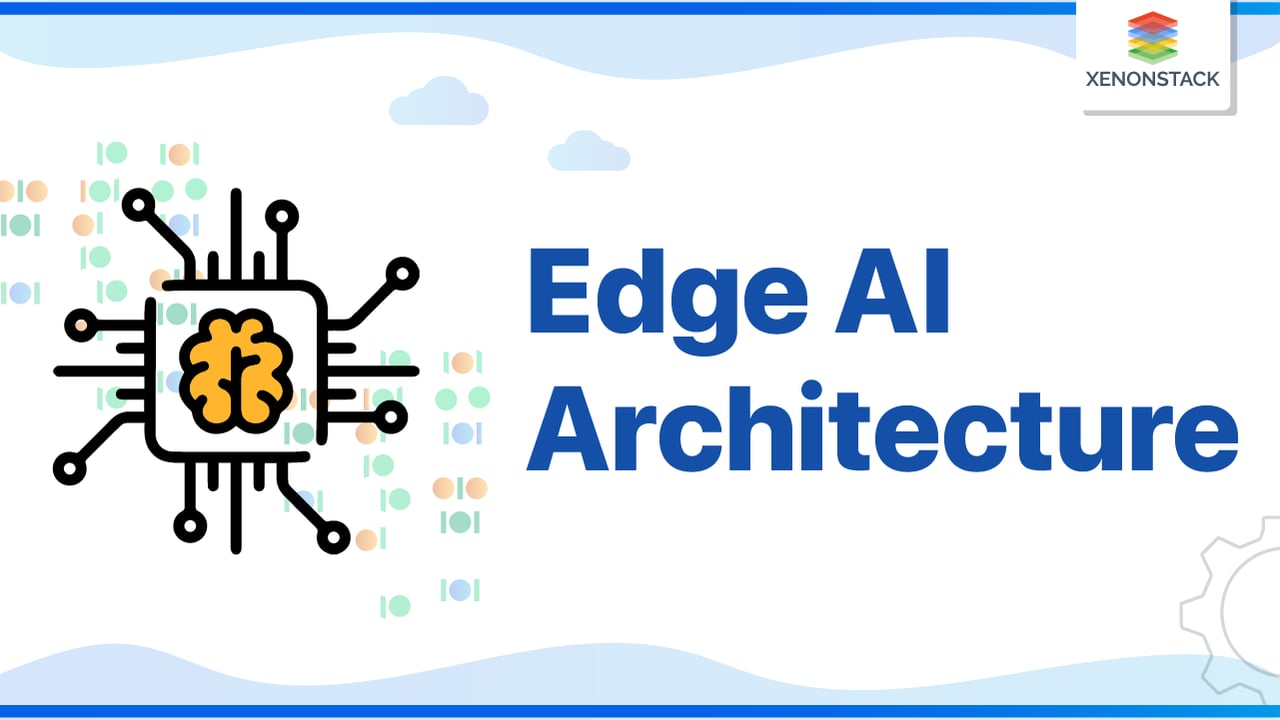
What is Edge Computing?
Edge computing architecture is a distributed computing paradigm that makes necessary computation and data storage closer to the devices where it is collected. Compared to relying on a central location such as a server, Edge Computing enables real-time data not to suffer from bandwidth and latency issues that affect device performance.
To put it more clearly, instead of running processes in the cloud, it runs operations like a computer, IoT system, or Edge Server at local locations. Long-distance communication between a client and server is now reduced by taking computation to a network edge. The capacity for computation and processing is needed to run Edge AI applications and algorithms directly on field devices, allowing ML and DL. The amount of data collected by IoT devices in the field is growing exponentially: Machine Learning and Deep Learning allow Edge AI applications to handle those data in real-time better. It creates Edge nodes where data can be stored, analyzed, sorted, and forwarded to the cloud for further analysis, processing, and integration with IT apps.
Combine it with AI = Edge AI
Edge AI means running AI algorithms locally on a hardware device using edge computing, where the AI algorithms process data generated on the device without any connection needed. It allows us to process data in less than a few milliseconds with the system, which gives information in real-time. We need a computer with a microprocessor and sensors to use Edge AI. Edge AI will allow real-time operations where milliseconds matter, including data creation, decision, and action. For self-driving cars, robots, and many other fields, real-time services are critical. Edge AI will reduce data communication costs because there will be fewer data transmissions.
Edge Computing allows more storage and processing resources and more Artificial Intelligence (AI) to be brought to the Edge, integrating powerful embedded and Edge computers, computational power, and IoT platforms to allow Edge AI. Edge AI means locally running AI algorithms on hardware computers. The algorithms work based on the use of computer-generated data. But because neural networks fuel most AI systems today, much computing power is needed to run these systems at the Edge. The challenge in meeting the AI inference performance requirements is to ensure the high precision efficiency of algorithms within low power consumption. But the advancement in hardware choices, including graphics processing units (GPUs), central processing units (CPUs), application-specific built-in circuits (ASICs), and system-on-a-chip (SoC) accelerators, has made Edge AI possible. Discover about Reimagining Consumer Data Privacy With Edge AI.
Overview of Edge Analytics
Requirements for Industrial Automation and Edge AI demand that decisions be taken in real-time. Data analytics must, therefore, be conducted at the Edge to provide immediate answers to critical issues. The IoT Edge platform offers an application development framework that is user-friendly, easy to digitize properties, and handles twins for advanced analytics and data management. Edge analytics is the collection, processing, and analysis of data either at or near a sensor, a network switch, or some other linked computer at the Edge of a network.
With the increasing proliferation of connected devices as the IoT grows, many industries, such as retail, manufacturing, transportation, and electricity, produce edge computing. Edge computing is a distributed computing paradigm that brings necessary computation and data storage closer to the devices where it is collected in large quantities at the network's Edge. Edge analytics is real-time data analytics or analytics at the platform where data collection occurs. Edge analytics may be descriptive, analytical, or predictive.
How does Edge AI work?
-
Data centres are also clustered servers, where the cost of real estate and electricity is lower. Data can’t fly faster than the speed of light except on the zippiest fibre-optic networks. This physical gap creates latency between data and data centres.
-
Edge AI takes this distance forward.
-
Edge AI can be run at multiple network nodes to close the data-processing gap, reducing bottlenecks and speeding up applications.
-
Billions of IoT and multiple devices run on lightweight, embedded processors at the periphery of the networks, which are suitable for simple video applications.
That would be fine if today’s industries and municipalities were not applying AI to IoT device info. However, they are designing and running compute-intensive models, and they need new conversational edge computing approaches.
The exponential growth of Artificial Intelligence-driven applications necessitates increased technical requirements for data centers, resulting in substantial cost implications.Read more about Enabling Artificial Intelligence (AI) Solutions on Edge.
Benefits of Adopting Edge AI
There are the following benefits of Edge AI -
-
Increase in levels of Automation
IoT devices and machines at the Edge can be trained to perform autonomous tasks. -
Digital Twins for Advanced Analytics
Digital data for real-time and remote management of devices in the field. -
Real-Time Decision Making
Real-time analytics to take action instantly and automate decision-making.
-
Edge Inference and Training
The application of training models and inference happens directly on the Edge device. -
Enable automated security management and performance management in real time With integrated Platform Services for process automation. Know more about IoT Application Development Services.
The Importance of Scalability in Edge AI
Another large concern regarding edge AI architectures relates to scalability because the capacity to scale up or down workload or user traffic or to incorporate innovations is a measure of flexibility with these sorts of systems. As organizations extend out more form factor devices and services that inhabit the edge, those businesses need architectures that can scale up, down or out without much cost in speed, accuracy or security.
Scalable Component on the Edge AI Architecture
Edge Devices
Network edges refer to the systems involved in the creation, collection, analysis and control of data and are situated at the boundaries of the network. These can include sensors and cameras, cars and industrial machines. In the end, it is possible to include Smartphones. Key characteristics of edge devices include:
-
Computational Power: AI algorithms set the constraint that at least most of the computations must be done by the devices in question.
-
Storage: In the more detailed sense, edge devices should have enough storage in the form of data parameters.
-
Connectivity: The WAN, which includes Wi-Fi and cellular connectivity, is required when accessing other devices or cloud services.
Edge Gateways
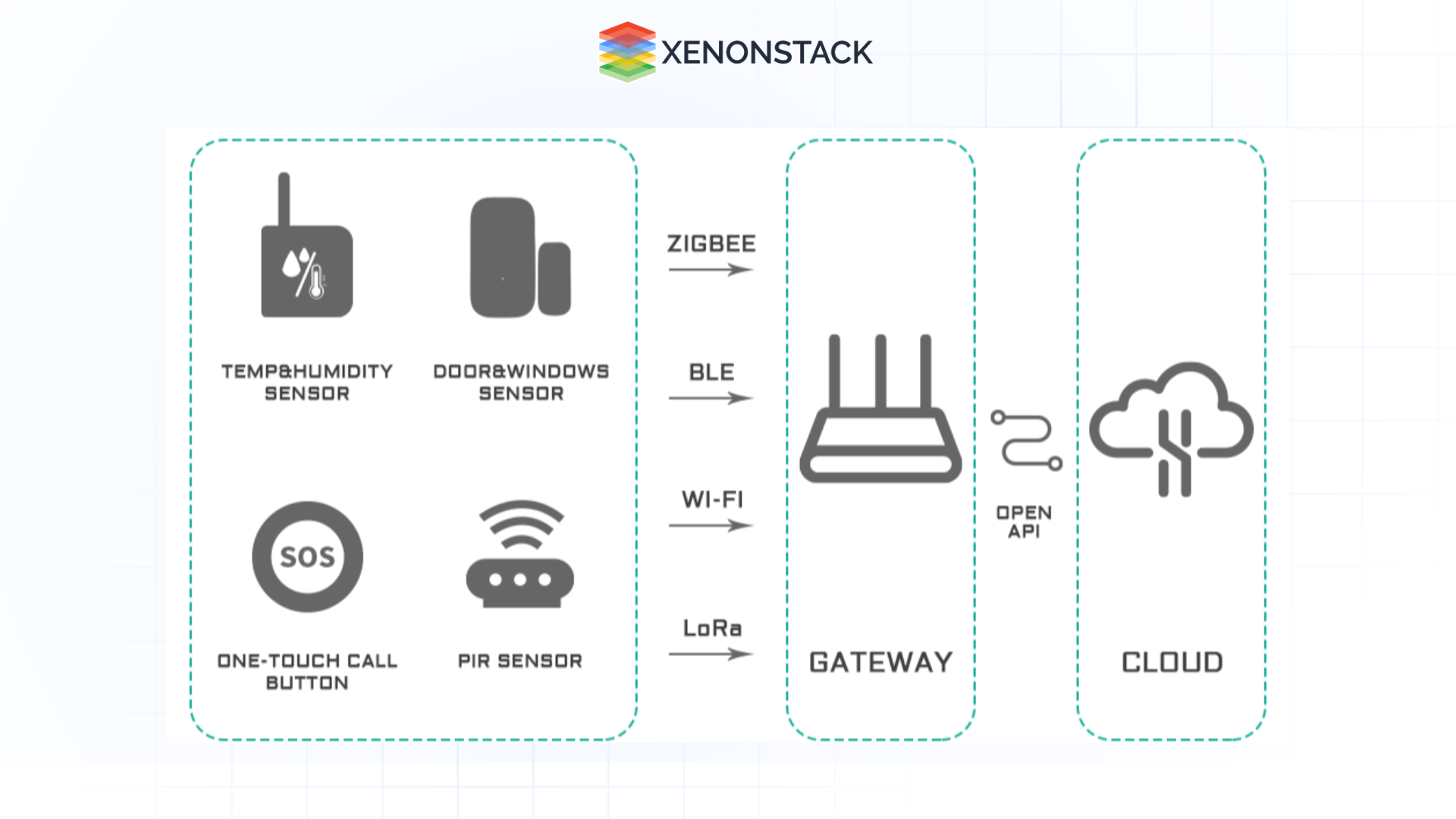
Edge gateways are centralized devices between the edge devices and the cloud or centralized data centres. Thus, edge devices gather information from other edge devices, perform computations, and transmit the data for further analysis or storage. Important functions of edge gateways include:
-
Data Aggregation: To collect information, combine all received data from several sources.
-
Protocol Translation: Thus, it requires the support of various communication protocols to make it compatible.
-
Security Management: The existence of proper measures intending to prevent the act of data shredding during data transmission.
AI Models and Frameworks
Scalable edge AI architectures try to use machine learning models for edge computing, which are optimized for edge conditions. These models require they are efficient, easily portable, and can perform inference on edge devices at high speeds. Existing AI frameworks for the edge are Tensorflow Lite, PyTorch mobile, and ONNXC. It offers tools to optimize models, quantize the models, and deploy tools, hence enabling developers to build scalable solutions.
Management and Orchestration Middleton
Accomplishing the AI workloads and managing their execution on the edges thus signifies capable management and orchestration tools. These tools help in:
-
Device Management: Supervising the health and standing of the edge devices.
-
Model Deployment: Coordinating access and distributing new AI-based models to various devices.
-
Data Management: Data accuracy and data protection, as well as regulatory policies.
Cloud Integration
While edge computing emphasizes local processing, cloud integration is crucial for certain functionalities, including:
-
Model Training: Sophisticated training exercises are conducted in the cloud, while minor models are conducted on the edge.
-
Data Storage: This is used to maintain large files to train the models and analyze the large data set.
-
Analytics: Conveying enterprise-level data analysis and collecting and consolidating data from several viewpoints at edge computing devices.
Scalable Edge AI for Future Growth
Customizable edge AI structures are now the leading breakthrough in industries globally, driven by digital advancement. These architectures allow organizations to achieve real-time data processing, improve decision-making, and optimize resource use to harness the benefits of AI for edge computing. There are still barriers to implementation, but the advantages of bringing scalable edge AI solutions are quite apparent. In the era of developing technologies and a progressing digital environment, companies must adapt to these processes to remain relevant within the global market dominated by large amounts of data.
Hence, by investing in strong and sound solutions, encouraging partnerships, and enhancing security, businesses can transform and embrace the path to an intelligent and efficient future. At this point, there can be no doubt that using intelligent, scalable architectures at the edge will be a key enabler in the evolution of the next generation of industrial systems, healthcare, smart cities, and other Edge AI applications.
Next Steps for Scalable Edge AI Architecture
Talk to our experts about implementing Scalable Edge AI Architecture to enhance decision-making across industries and departments. By leveraging agentic workflows and decision intelligence, organizations can become more decision-centric. Scalable Edge AI optimizes IT support and operations, significantly improving efficiency and responsiveness while enabling seamless deployment and growth.