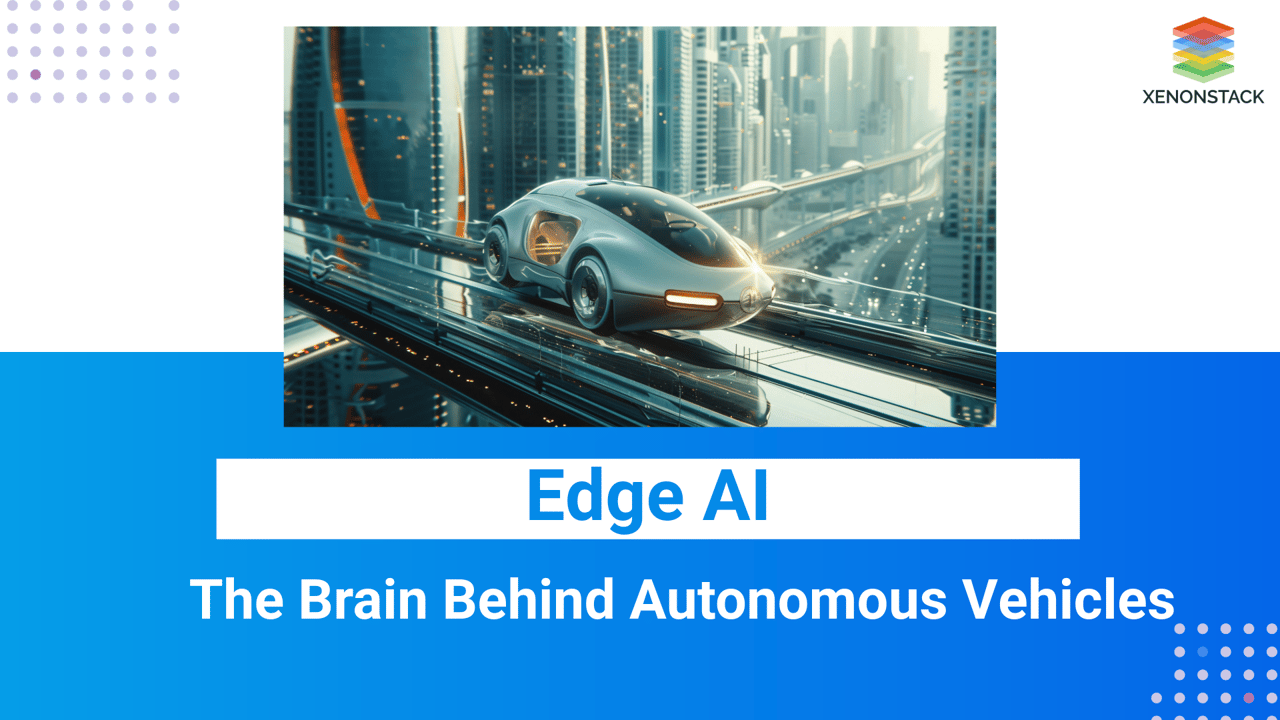
Overview of Autonomous Vehicles
While kickstarting the journey towards the creation of autonomous vehicles, Edge AI is gaining prominence. In general, cloud computing has been used to support the development of artificial intelligence and machine learning, but to support autonomous driving, there are new demands for real-time decision-making, low latencies, and constant connectivity. That is where Edge AI comes into play and revolutionizes in-vehicle computing by processing data on the edge, in proximity to the data source. This change has opened what has the potential to be a safer, more reliable, and more efficient autonomous driving experience.
How Edge AI Works in Autonomous Vehicles
Onboard AI allows self-driving cars to decide on their next action instantly through the information collected by their sensors without additional dependence on cloud systems, thus allowing them to realize greater safety and effectiveness. Fully with LiDAR, radar, cameras, and GPS, the vehicle is collecting data on obstacles, road status, and paths constantly, and the Edge AI module processes all of these inputs. This makes it possible for the vehicle to perform precise actions in new settings where appropriate, such as steering away from an obstacle or adapting speed and providing predictive decisions from historical and real-time data.
On the other hand, real-time processing is executed at the Edge AI level; the car interacts with the cloud regarding non-real-time processing, model updates, and diagnostics using V2X technology over the 5G network. This makes it possible for the vehicle to receive constant improvement through learning from local model updates through the cloud. The system also provides data privacy through the local processing of personal data and is feasible in areas of low network coverage. Edge AI enables the vehicle to operate autonomously at the edge and use cloud computing resources only for other non-time-sensitive operations. In addition to this, 5G high-speed network connectivity to other vehicles and Roadside Units improves the level of situational awareness, while the Vehicle Control Unit handles important vehicle systems such as acceleration, braking, navigation, etc.
Architecture Diagram of Edge AI for Autonomous Vehicles
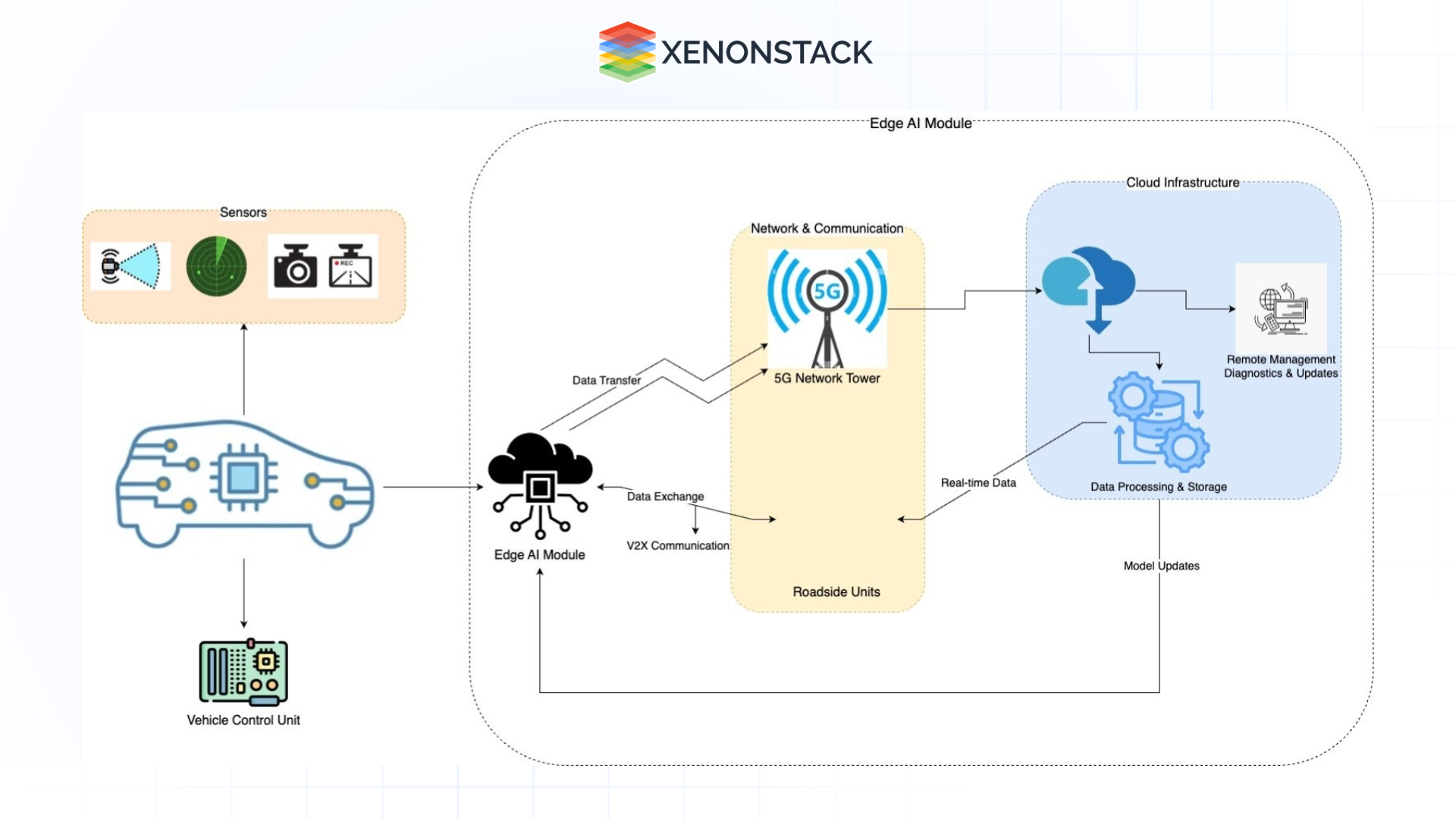
Diagram Overview
Vehicle Components:
-
Sensors: An accurate scanning device, such as LiDAR, RADAR, GPS, and a high-resolution camera.
-
Edge AI Module: Real-time data handling and decision-making processes are performed using artificial neural networks.
-
Vehicle Control Unit (VCU): Oversees the fundamental functionality of a vehicle’s systems acceleration, braking, steering, and routing in accord with choices made by the Edge AI and info derived from the sensors it controls.
Network Infrastructure:
-
5G Network Tower: This tower supports vehicle-to-everything (V2X) communication, thus providing superior and efficient speeds and minimal latency for transferring vectors between vehicles and the cloud server.
-
Roadside Units (RSUs): The C2X communication between vehicles will exchange information with fixed objects and deliver information about traffic conditions, obstacles, and other important information in real-time view.
Cloud Infrastructure:
-
Data Processing Center: This is used for offline data analysis and model updating.
-
Cloud Storage: Helps to maintain history to analyze or store it for future use.
-
Remote Management Interface: Used to update software, diagnose, and perform general maintenance
Role of Edge AI in Autonomous Vehicles
Self-driving cars have to operate with and react to information from LiDAR, radar, cameras, and GPS in real-time. In using cloud servers, though, latency is involved, which does not allow AVs to make the split-second decisions required for a vehicle to move safely. These are limitations that Edge AI helps to overcome because, in this model, vehicles process and analyze the data they require without necessarily querying the cloud for information. Here’s how Edge AI directly addresses the unique challenges of autonomous vehicles:
-
Latency Reduction
Edge AI can perform computations within the vehicle instead of suffering communication latency like cloud models. Because data processing is done locally, the AV can respond immediately to its environment, including perceiving objects on its path and speed or the meaning of traffic signs. -
Data Security and Privacy
Self-driving cars produce big data. Data preprocessing helps maintain data privacy by allowing the least transfer possible in the cloud, thereby minimizing interception scenarios. Moreover, local processing assists AVs in addressing the privacy issue by reducing the chance of data leakage: firms must manage data within a constrained environment and adhere to data protection legislation. -
Bandwidth Efficiency
Constantly transmitting sensor data to the cloud requires immense bandwidth, which isn’t always feasible on the move. Edge AI reduces the bandwidth demand by processing raw data on-site and only sending selected, processed insights to the cloud as needed. This reduces costs and enhances network efficiency. -
Enhanced Reliability
Cloud connectivity may vary due to bad signals in some areas or many people using the internet in densely populated buildings such as apartments. This is because, with Edge AI, the computations that are critical to the functioning of the AVs are done at the edge when network coverage is not up to standard.
Technologies Behind Edge AI in Autonomous Vehicles
Edge AI for autonomous vehicles is a system that combines high-performance computing and AI algorithms with robust small design. Vendors like Lanner deliver AI-ready edge computer systems for application in autonomous and intelligent driving. The main components and features making Edge AI viable include:
- High-Performance In-Vehicle Computing Systems: There are ready AI hardware platforms, such as Lanner’s NCA-5710 and NCA-5520, which range from intelligent autos; these come with Intel Xeon processors ideal for running demanding AI operations in automobiles. These edge systems ensure real-time data processing and analysis, converting mere sensor data into useful information.
- Sensor Data Aggregation and Compression: Self-driving cars have many onboard sensors, such as radar, LiDAR, and cameras, emit data constantly. Edge AI gathers and condenses this data before analyzing and making decisions, thus shortening the process time.
- Scalable Storage and Connectivity Options: Since the edge computing systems developed for AVs are portable, they incorporate options for both HDD / SSD / NVMe storage and LTE / 5G connectivity. They accommodate the constant high-speed connection required in AV management.
- Rugged Design for Extreme Conditions: Incidentally, edge computing devices are ideal for vehicle environments that are rugged, possibly with built-in batteries, as in the case of Lanner’s V3S series. These units meet designations such as MIL-STD-810G, mainly to guarantee their operations in various environments.
Key Edge AI Applications for Autonomous Driving
Edge AI’s applications within autonomous vehicles are wide-ranging and crucial to achieving self-sufficiency in AVs:
-
Real-Time Object Detection and Tracking
Edge AI supports real-time object detection and tracking, such as pedestrians, other vehicles, and road obstacles, within the automotive environment. It is important to avoid collisions during movements within and across facilities, corridors, or tunnels. -
Predictive Maintenance and Diagnostics
Edge AI tracks vehicle performance and can easily predict when some components may fail, leading to maintenance scheduling. Thus, through early problem detection, vehicles can prevent certain unanticipated breakdowns, improving the overall reliability of the fleet. -
Traffic and Environment Analysis
The edge AI allows the AVs to perceive the possibility of traffic congestion, road conditions, and even climate conditions like weather. Some of this data aids in route planning, so vehicles can change routes flexibly depending on conditions on the ground. -
Driver and Passenger Safety Monitoring
Edge AI always observes the driver and passengers to ensure their safety. Should a driver become distracted or tired, the system will remind him or even take control of him if necessary.
Real-World Edge AI Solutions for Autonomous Vehicles
With the advancements of edge AI, automotive manufacturers and tech providers are increasingly implementing edge solutions in autonomous driving:
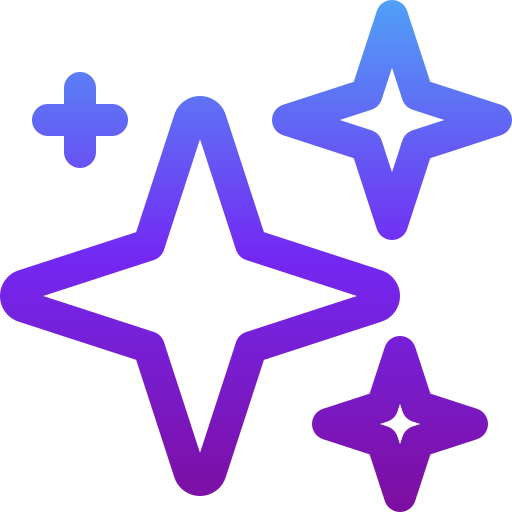
Lanner’s NCA-5710 Edge AI Platform
The NCA-5710 uses an Intel Xeon processor with Deep Learning Boost to efficiently process AI computations for autonomous vehicles (AVs). It enables real-time decision-making without cloud dependency.
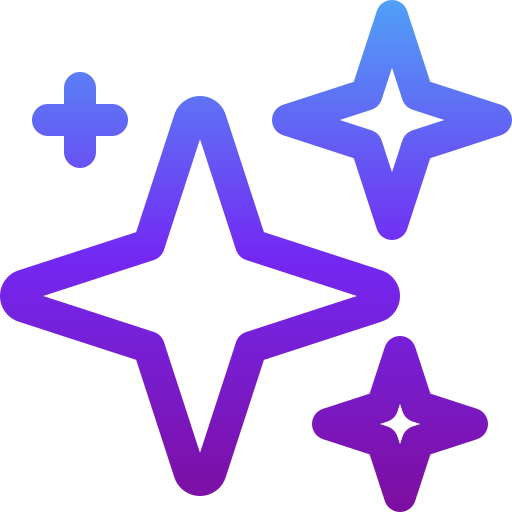
V3S Ruggedized Edge Solution
The V3S is a compact, low-power solution with multiple I/O ports for sensor integration. MIL-STD-810G certified, it provides reliable performance in extreme conditions, ensuring AVs can operate in both city traffic and off-road.
The Future of Edge AI in Autonomous Driving
With the advancement of AV technology, the possibilities and opportunities for edge AI will also increase. Some anticipated developments include:
-
Increased Integration with 5G and Future Networks
Although Edge AI solves many latency concerns, as 5G and beyond come into the picture, their latency will be even less than ever. This will give AVs a chance to process more data without any delay, which in turn will help give them more autonomy and safer operation. -
Advancements in AI Algorithms for Autonomous Decision-Making
As AI evolves progressively, edge systems will take on additional higher-level tasks such as gesture recognition and intention identification so that AVs can make more subtle decisions in real-time. -
Collaboration with Cloud Computing for Hybrid Solutions
It looks like a model based on the intelligent use of both edge and cloud computing may become the norm. This setup will enable AVs to function autonomously, but with the cloud auxiliary data processing, the less imperative and bursty ones like renewed map data and intricate routing algorithms.
Key Takeaways
Autonomous vehicles are set to be transformed through Edge AI. However, with Edge AI, the computations are done nearer to the source of the data, eliminating latency, bandwidth, and security as factors that will harm the efficiency of an autonomous vehicle. The ability of vehicles to operate through Edge AI, including Lanner’s computing platforms, makes it easier for automobile manufacturers to continue developing autonomous vehicles that are safer, smarter and capable of handling real-world scenarios. The combination of AI and edge computing is the future of autonomous vehicles, moving us closer to a future where self-driving cars are not just a possibility but a reality that holds real promise of being an efficient and safe conveyance meant for transport to citizens.
Next Steps for Autonomous Vehicles
Talk to our experts about implementing Edge AI for Autonomous Vehicles and how industries leverage compound AI systems to enhance decision-making. By utilizing agentic workflows and decision intelligence, organizations create a decision-centric approach. Edge AI automates and optimizes processes, enabling real-time data analysis and operational efficiency, which is crucial for autonomous vehicles to navigate safely and effectively. This scalable solution improves responsiveness, making it a game-changer in the evolving automotive landscape.