In today’s fast-growing and expanding Artificial Intelligence market, Edge AI is transforming how real-time object detection is done across sectors. Edge AI allows AI computing abilities at the edge device itself, cutting down latencies, maximising efficacy and yielding real-time results that are important for use cases, including self-driving automobiles, surveillance and smart cities. In this, we will discuss what it is and is not, what the challenges are, and what the main Edge AI architectures are, and we will give tips for using it to perform real-time object detection.
Edge AI is the AI where the AI model is run at the edge devices and not in a central Cloud Computing platform. Edge AI processes data near the source of its origin and hence does not involve the time delay of sending data to the cloud for analysis. This technology is reemerging in a key role as industries move toward more self-contained, self-sufficient systems that need information interpreted immediately and acted upon.
The Role of Edge AI in Object Detection
One widespread use case of CV is Object Detection, a task to locate objects within an image and/or video. In traditional object detection tasks, the main approach was to use cloud computing for the analysis, but with the current advancements in Edge AI, this has changed. Edge-based object detections enable real-time analysis and can be applied in real-time decision-making processes, such as autonomous vehicles or surveillance systems.
Importance of Real-Time Object Detection
Real-time detection of objects is crucial in many sectors, including automotive, healthcare, and industrial automation, where decisions must be made instantly. For instance, self-driving cars must recognise pedestrians, adjacent automobiles, and hindrances within the street they intend to drive on in real-time. Likewise, timely identification of objects or suspicious activity is important for security and reaction to a breach in smart surveillance systems.
Reducing Latency for Faster Decision-Making
Object detection is one area of application that benefits significantly from the low-latency feature of Edge AI. Recent AI models are based in the cloud, and for data to be processed, it has to be sent to the cloud servers, leading to a lot of latency. Edge AI solves this problem by processing the data in the edge environment; hence, the response time is nearly instantaneous. In related areas such as autonomous driving, eliminating latency could be the between a successful manoeuvre on the road and an accident.
Reducing Bandwidth and Enhancing Privacy
Another advantage of Edge AI is bandwidth and privacy. As data is processed locally, only the required information is transferred to the cloud for storage or further analysis. This minimizes the volume of data that has to be transmitted and, thus, the available bandwidth, particularly for systems with modest bandwidth. Also, storing data on local units, such as video feeds or personal information, reduces the vulnerability of such information leaking during transit.
Key Considerations for Edge AI Object Detection
This paper identifies various aspects of choosing an object detection model for an edge AI use case. These include:
Resource Constraints
Acting devices routinely lack computational capability, storage, and power consumption ability in the cloud. The selected object detection network has to be relatively small to fit into such limited devices while providing high accuracy. Therefore, single-stage object detectors such as You Only Look Once (YOLO), and Single Shot Detector (SSD) are mainly used in edge environments because they offer enhanced performance in terms of time complexity and memory requirements.
Performance Requirements
For real-time applications, the generated object detection model should be able to detect new frames at a frequency sufficient to meet the application's response time. This model must have a high frame rate while still having a good accuracy to computational load ratio. For example, YOLOv5 achieves more than 30 frames per second (FPS) on entry-level gadgets, which explains why it is frequently used for edge-based object detection.
Post-Processing Efficiency
Additional procedures, such as Non-Maximum Suppression (NMS), are relatively complex and require much computation, making them unfeasible in edge devices. Selecting architectures that are as free from intra-connection tasks at higher levels helps increase performance. For instance, CenterNet, an anchorless object detection framework, does not employ NMS, making it ideal for edge AI usage.
Major Architectures for Object Detection on the Edge
Several object detection architectures are commonly used in edge AI applications, each with its own strengths and weaknesses:
YOLO (You Only Look Once)
The YOLO family of object detectors is fast and easy to use. YOLO works perfectly well since it passes through all the images simultaneously, making it fast and recommended for real-time applications. YOLOv5, the latest in the series, is designed to work well on edge devices with better accuracy, faster detection, and efficiency in terms of space, memory, and energy consumption.
SSD (Single Shot Detector)
SSD is a multi-scale object detection architecture that can easily detect objects of different sizes. Because the feature maps are utilised in multiple levels of pyramid layers, it is exhaustive to predict objects in a variable resolution; thus, it is suitable for applications in cases of different sizes of objects. The model is also lightweight to enable deployment at the edge of devices with constrained computational power.
CenterNet
CenterNet is an anchorless object detection framework where we directly predict bounding box dimensions from feature maps. Yolov3 streamlined the process by completely eliminating the anchor box, which has been known to cause a lot of computational work. It turned out that CenterNet is particularly effective in edge devices as it doesn’t require non-maximum suppression (NMS), which increases the algorithm’s efficiency even more.
Advantages of Edge AI for Object Detection
Edge AI offers several advantages over traditional cloud-based AI systems for object detection:
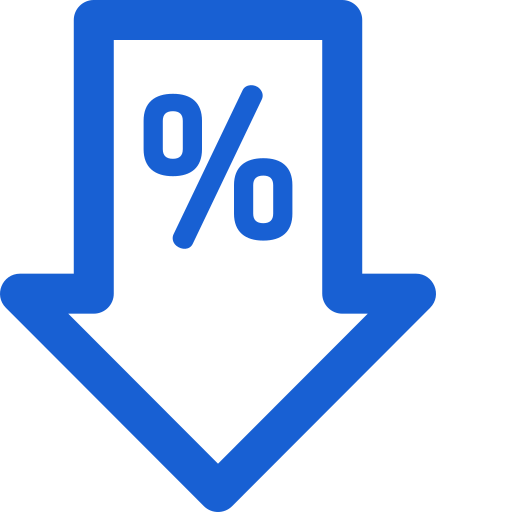
Low Latency
Minimizes significant delays in data transmission to the cloud, thereby enabling real-time object detection and analysis
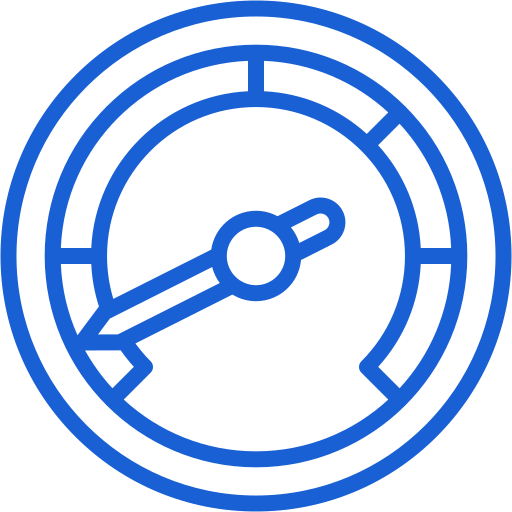
Bandwidth Efficiency
Processes data at the network's edge, reducing cloud transmission and network load
Architecture Diagrams and Flow Charts
To illustrate the flow and interaction between various components in an Edge AI object detection system, the following diagrams provide a clear understanding of the architecture:
Diagram 1: Basic Flow of Edge AI Object Detection
Explanation:
-
Edge Device - This is possible because edge devices collect and analyze images or videos using local AI models, enabling sensor-based real-time inference.
Examples: Raspberry Pi, NVIDIA Jetson, Google Coral, Mobile Phone, IoT Camera. -
Object Detection Model - Edge AI models applied in this case can detect and classify objects present in captured images or videos with desirable accuracy and at a fast rate.
Examples: YOLO (You Only Look Once), SSD (Single Shot Detector), MobileNet, EfficientDet.
-
Inference at the Edge—Inference means using the trained model locally on the edgeware to make predictions, such as object detection.
Examples: TensorRT, OpenVINO, TensorFlow Lite, ONNX Runtime
-
Detected Objects - Recognised objects are people or vehicles, and they are assigned labels with bounding boxes. These cause actions, including alerts or displays of the results.
Examples: Person, Vehicle, Animal, Package
-
Send Results to Cloud (Optional) - Data generated and processed may include objects, meta-data, etc., and may be output and transferred to the cloud for further processing.
Examples: AWS IoT Core, Azure IoT Hub, Google Cloud IoT Core
-
Cloud Storage/Processing - Edge data is retained on cloud platforms for analysis, additional model training, and integration of an ML model with enterprise solutions.
Examples: AWS S3, Azure Blob Storage, Google Cloud Storage, AWS Lambda, Azure Functions
Diagram 2: YOLO Object Detection Workflow on Edge Devices
Explanations:
-
Image Preprocessing on Edge Device - Object coordinates, width, and height are estimated by extracting video frames and resizing them to the dimensions YOLOv5 accepts as inputs.
-
YOLOv5 Model Inference - The obtained images are then resized to be processed using the YOLOv5 model, which is an object detection model.
-
Bounding Box and Class Prediction - The model then gives the bounding box and labels the objects detected in the image.
-
Display Results on Edge Device - The detection result's output is shown locally in an edge device, such as a screen or a mobile application.
-
Optional Data Transmission to the Cloud - Processed data may be streamed to Big-data storage solutions such as Amazon S3 or Azure Blob storage for further computation or archival.
-
Model Retraining and Evaluation - The system also incorporates a retraining unit and an evaluation module. These modules allow the model to be updated when new data is received, thereby facilitating increased object detection accuracy.
Diagram 3: End-to-End Architecture for Real-Time Object Detection on Edge
Explanation:
-
Sensor/Camera (Data Capturing) - This captures raw data, such as an image or a video stream.
-
Edge AI Device (Run Object Detection Model) - The edge device then has the capability of running AI models to process the data in real-time.
-
Inference Engine (Detected Objects & Predictions) - The AI model provides the output from the input data in the form of predictions it will make and manages to outline objects.
-
Cloud for Further Analytics (Optional) - The processed data can be forwarded to a cloud to be analyzed and or for storage space.
-
Cloud Storage/Analysis - Data is kept in the archives for further analysis or review in the future.
-
Edge Device Display/Action - Alerts are interactive, shown with the detection results in real-time on the edge device or activated certain actions.
-
Real-Time Alerts/Control - Detections are linked to alerts or actions taken by automation.
Challenges of Implementing Object Detection on the Edge
While Edge AI offers numerous advantages, it also comes with several challenges:
-
Limited Computational Power
The computing power available on edge devices is generally lower than on cloud systems. Thus, the types of AI techniques that can be applied are limited. -
Energy Constraints
In addition, most IoT devices are mobile and rely on batteries to power the edge device; hence, AI models must be power efficient to enable the longest battery life. -
Heat Dissipation
Executing extended sets of deep AI computations on the devices requires efficiency, which can lead to the generation of heat, which is a real threat to the devices or their lowered performance.
Final Thoughts
Edge AI continues to disrupt real-time object detection since it allows for quicker and more secure data processing on edge devices. Given that sectors such as automotive, healthcare, and smart cities are embracing Edge AI solutions, such factors as compact, highly efficient, and light object detection models will continue to be sought after.
In choosing the rightful architecture and adapting to limitations inherent in edge devices, organisations can develop real-time object detection to its optimum and pave the way to future innovations in autonomy and responsiveness.
Explore about Retail Surveillance Using Edge AI Discover more Edge AI in Video Analytics
Next Steps
Talk to our experts about implementing compound AI system, How Industries and different departments use Agentic Workflows and Decision Intelligence to Become Decision Centric. Utilizes AI to automate and optimize IT support and operations, improving efficiency and responsiveness.