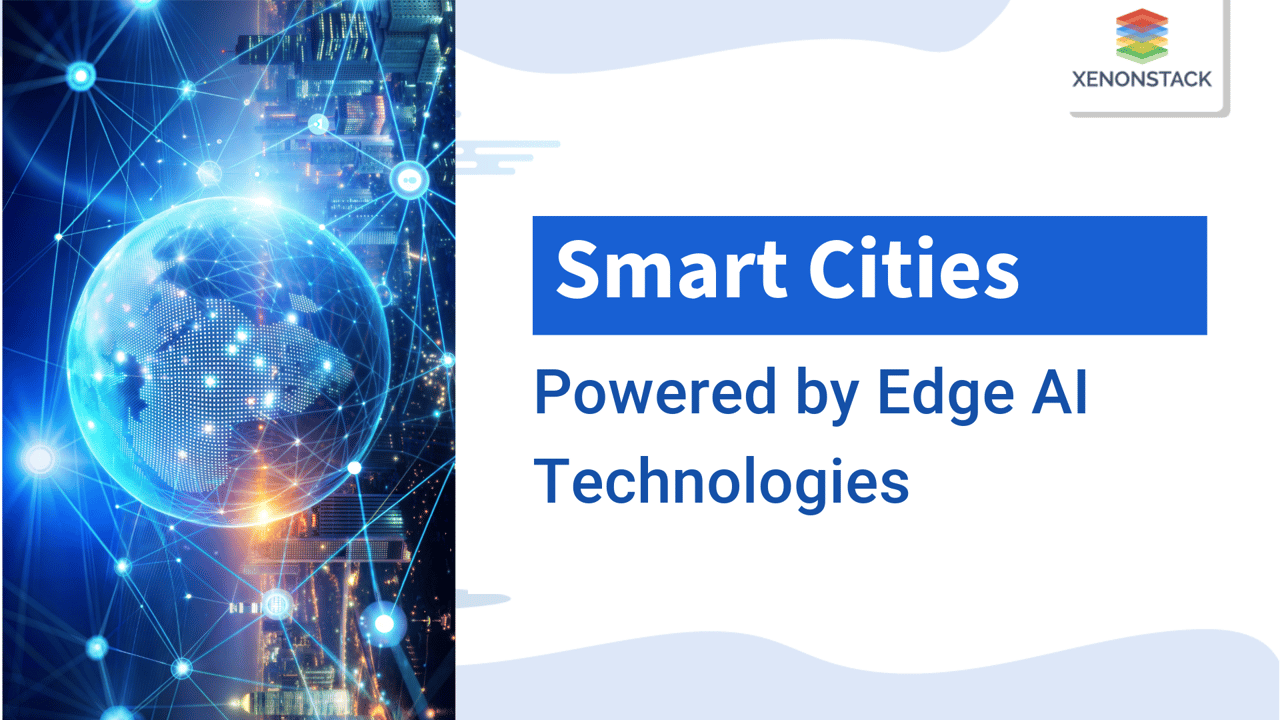
Environmental Monitoring and Sustainability
Edge AI allows cities not only to control and improve air quality, noise degree, and water quality with the help of sensors but also to process the collection of data they obtain simultaneously. That information helps cities to act correspondingly and prevent the violation of environmental legislation and deterioration of public health, as well as to promote sustainable development of cities. Further, Edge AI is used to sense the life of infrastructures in urban areas, predicting the wear out constantly.
Transforming Urban Data Processing with Edge AI
Smart city systems leverage the decentralized computing offered by Edge AI to avoid problems of high latency and large bandwidth demands related to the cloud. By deploying Edge AI, smart cities can achieve:
-
Reduced Latency: Analyzing data closer to the source is significantly more effective for real-time reaction to events.
-
Enhanced Privacy: There is no need to upload information to cloud servers or anonymize the data it processes. Sensitive data can be processed locally.
-
Bandwidth Optimization: Reducing data traffic to central servers helps conserve each network’s infrastructure, which, when optimally utilized, is essential.
Traditional Cloud-Based AI vs. Edge AI in Smart Cities
Figure 1: Traditional Cloud-Based AI vs. Edge AI in Smart Cities
Explanation:
As for the characteristic of traditional cloud-based AI, the data from IoT devices first transmits to clouds for processing and thus experiences the time for network bandwidth and data transmission. Unlike central AI, where data collected from the users’ side assets such as sensors and cameras are transmitted to the cloud server where data analysis is performed, in Edge AI, a portion of this data is processed at the edge nodes. This configuration increases real-time reaction capabilities, reduces bandwidth consumption rates, and fosters swift decision-making precisely at the point of generation.
Technical Architecture of Edge AI
Edge AI systems for smart cities typically follow a three-tier architecture: These are classified into Edge Devices, Edge Servers, and what is referred to as Centralized Administrative Control. Here’s a breakdown of these components:
Edge Devices
At the edge level, information acquisition tools such as cameras, sensors, IoT devices, and gadgets embedded with intelligent computing systems acquire and initially pre-process the data. Most of these devices possess compact AI models that enable the performance of real-time analysis at the site.
Edge Servers (Local Gateways)
Node locations are deployed within the city as local centres, collecting information from various edges. These servers make more complex AI models with increased capability for data processing operations such as video analysis, abnormality detection, and predictive maintenance. They also do edge aggregation, where only important data is passed through to the main servers if required.
Centralized Management and Cloud Integration
While most of it is managed locally, central management servers compile data from different edges and produce insights, forecasts, and long-term reports. This tier is often also responsible for updating the models, overseeing devices, and analyzing various system performances for ongoing enhancement.
Edge AI Infrastructure
Figure 2: Edge AI Infrastructure for Smart Cities
Explanation:
Sensors and cameras of smart cities are gathering data all the time, and such information is sent to the closest edge server, which possesses AI algorithms. These edge servers are responsible for processing information, identifying problematic cases, and responding to them. Such critical insights are then communicated to centralized servers for further analysis of the entire city, as well as for archiving and reporting. This format enables cities to perform partial processing, which saves bandwidth and offs mainly while potentials simultaneously perform measurements of city-wide trends in control centres.
Benefits of Implementing Edge AI
-
Improved Responsiveness
Edge AI performs calculations right at the source, enabling immediate reaction to particular events. This is especially important in emergencies, where waiting for a response can be life and death. -
Data Privacy and Compliance
Academics also suggest that Edge AI enables organizations to adhere to especially strict data privacy laws because most data never leave the local area. -
Cost Efficiency
In transforming some processing tasks traditionally done centrally on the cloud, edge AI saves a significant amount of data traffic to and from the cloud, thereby lowering the cost of cloud storage and data transmission. On a scale, in smart cities especially, this can mean significant cost optimizations. -
Scalability
Edge AI also enables the deployment of smart city solutions on a large scale by distributing the processing of AI tasks among many edge devices and servers while generating a need for large-scale investment in cloud infrastructure.
Challenges and Considerations
-
Device and Model Optimization
AI models' decisions at the edge device must be optimized for low power and memory requirements, which requires considerable effort in model training and tuning. -
Security Vulnerabilities
New security challenges arise at the edge of AI because the opportunity to manipulate local devices can be higher. Measures must be implemented and enforced for these devices to utilize sound encryption and secure access. -
Maintenance and Model Updates
It is challenging to manage a substantial number of edge devices distributed throughout a city due to constant conditions, new urban issues, and models that require changes.
Deploying and Updating Models
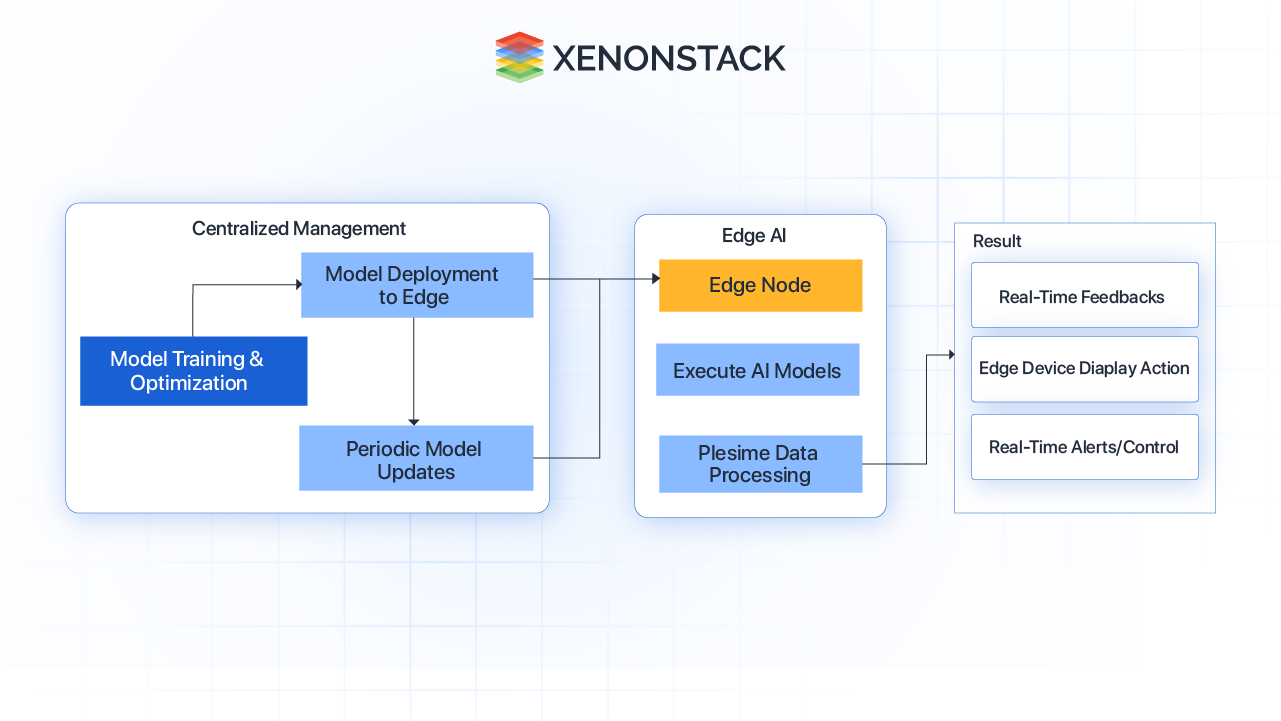
Figure 3: Edge AI Model Deployment and Update Cycle
Explanation:
Central management creates, builds, and deploys AI projects specific to the edge and communicates these models to edges all across the city. Once deployed, these are edge devices, and they parse the incoming data in real-time. This is synchronized from the central server to the edge nodes to update them on the new trends of data models required at the nodes. This architecture helps models remain current, ensuring real-time analysis can be performed continuously without over-reliance on the cloud.
Concluding Insights
Edge AI is a revolutionary concept and a key enabler of smart city development because it means that the data processing takes place where it matters the most, on the edge. This decentralized, real-time processing allows cities to act quickly towards important matters and on multiple aspects of life within safety, resource, and environmental domains. The Edge AI has been revealed to strike a balance by minimizing latency and narrowing down the data transmission to some centralized cloud systems, making it possible for cities to operate optimally and securely to meet the ever-evolving needs of modern society.
Therefore, Edge AI is the solution for effective, sustainable management of cities depending on population increase and the migration pattern of cars. As seen in smart mobility and energy consumption, the capabilities of Edge AI include managing efficient traffic flow, minimizing jams, successful energy consumption, and waste minimization. Such advancements not only optimize the construction of physical structures that constitute a city but also support current global sustainable development objectives, such as minimizing the effects of climate change, as well as the improved use of resources.
However, like any new concept, the implementation of Edge AI has its drawbacks – especially regarding security, scalability and system maintenance. Strengthening the future viability and prospective size of reported systems, which should connect to the cities, is important for sustained success. Furthermore, Edge AI’s integration with upcoming technologies, such as Blockchain, can create more opportunities, such as developing reliable, secure and transparent systems in smart city sub-areas, such as traffic control, energy supply, and data protection.
Read About Edge AI vs Federated Learning- Learn More Edge AI Architecture and Benefits