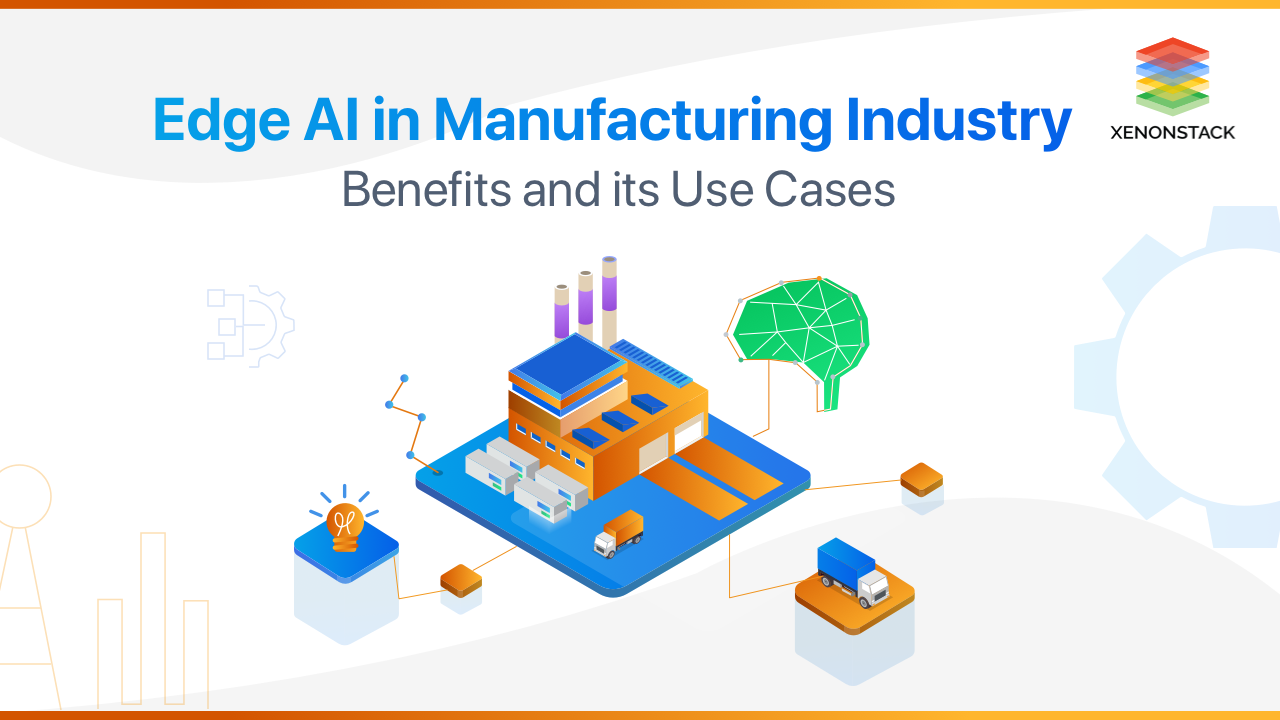
Industry 4.0 and Intelligent Edge Revolution
The Fourth Industrial Revolution, or Industry 4.0, is the fusion of physical and digital systems at the factory level, linking intelligent things and enabling a smooth data flow across operational boundaries. Industry 4.0 is revolutionizing industries by utilizing advanced data capture, analysis at the edge and high-performance computing as the basis for innovative solutions and unparalleled efficiency.
Edge AI represents a fundamental pillar of Industry 4.0, helping glean insights and intelligence in real-time directly from the point of data generation. Processing information locally minimizes latency, improves data security, and keeps operations uninterrupted.
Enabling Technologies Driving Industry 4.0
Open architectures, standards-based solutions, and advancements in edge, network, and cloud technologies are key enablers of Industry 4.0. In many cases, these innovations bridge the gap between IT and OT to create smarter systems, connected pieces that interact with each other.
Why Industry 4.0 Matters
Factories can efficiently manage and optimize their operations with software-defined infrastructures that are flexible and scalable. The deployment of 5G and accessible AI solutions, even for smaller factories, enables this, creating radically new operational and competitive possibilities for them.
Mounting intelligence on edge will allow machines in the production units to make higher-level decisions, act autonomously, and give feedback so that stakeholders can detect flaws.
How Edge AI Works in Manufacturing Units
Edge AI has a simple architecture in which processing units can deploy a pre-trained model on edge, i.e., near the data source. However, one should note that the model on edge will only score the training part where there is no limitation for the computational power.
The process has the following stages:
-
Data Ingestion: This stage will ingest the real-time sensor data from the machines' monitoring systems into the pipeline.
-
Storage of the Data: The stream of the data will be stored locally and securely as no cloud processing is involved here.
-
Processing: This stage of the pipeline will process the data according to the needs of the pre-trained models.
-
Analysis: Here the data will be analyzed by the models, and they will give the results.
-
Results: This stage compiles the results. After these results, the stakeholders can take the response.
Enhancing Factory Operations with Edge AI
There are a variety of systems and machinery powered by factories. Edge AI supercharges these systems, making them smarter and improving operations and outcomes. Examples include:
-
Industrial Robots: Edge AI enables robots to perform precision tasks like assembly, inspection or packaging.
-
Process Automation Systems: Integrating AI in SCADA and PLC systems provides data-driven, adaptive process control.
-
Energy Systems: Edge AI saves energy in real-time by balancing the supply and demand for factories in energy and utilities.
This technological leap brings factory use unparalleled efficiency, quality and responsiveness across industries.
Unlock real-time insights and optimize production with Enterprise Manufacturing Intelligence tools. Explore More.
Top Edge AI Use Cases in Manufacturing
Predictive Maintenance
Predictive Maintenance refers to the ability to pre-emptively detect the failure of machines using machine learning predictive algorithms.
Predictive Maintenance has been in the industry for some time, but it has also been difficult to implement. Edge AI can play the role of catalyst to smooth out this process as it can process the data near the edge, making it simpler and more efficient to implement.
-
Real-time fault predictions
-
Helps in building advanced breakdown strategies
-
Cloud-less predictive results
Condition-based Monitoring
Manufacturing units face challenges simply trying to fetch the data from their machines, processes, and system. One of the biggest hurdles is that each manufacturing units have their data streams, now firstly, all of these streams, whether of use, are sent to the cloud. Then processing is done. If some initial filtering can be done, then only useful data streams can be utilized in the cloud or locally, this can be achieved with edge ai near the data generation streams.
-
Condition Monitoring can help increase the revenue as less cost is needed for maintenance.
-
Condition monitoring makes the processing of these manufacturing more agile.
-
One can utilize multiple streams to achieve the decisions as processing is on edge.
Precision Monitoring and Control
One of the main goals of Industry 4.0 is to use the data from multiple machines, processes, and systems from the manufacturing unit and use them for smart controlling and making precise decisions in real time. This precision monitoring and controlling system uses a large amount of data Machine learning algorithms. Edge Computing is a perfect fit for it as it can collect, aggregate, and filter the data used by the AI/ML algorithms.
-
Distributed processing
-
Making Industry 4.0 more achievable
-
Direct the manufacturing unit to more autonomous and sell-tolerant machines, aka smart machines.
Edge AI Applications in Various Industries
Power and Utilities
-
Grid Optimization: Balances electricity supply and demand in real-time, helping to keep power plants stable and reducing energy waste.
-
Asset Management: Constantly tracks critical infrastructure, such as transformers and lines, alerting users of pending problems before they become apparent.
Pharmaceuticals
-
Batch Monitoring: Tracks production processes to ensure compliance with stringent industry regulations and ensure product quality and safety.
-
Drug Development: Speeds up research and decreases costs, providing real-time feedback during experiments.
Automotive
-
Smart Manufacturing: Monitors vehicle components on production lines to achieve precise assembly, reducing rework.
-
Safety Testing: Detects inconsistencies in crash tests or part quality to help improve overall vehicle safety.
Energy
-
Renewable Integration: Controls outputs from solar and wind farms when spiking.
-
Predictive Analytics: Monitors energy transmission systems in advance to avoid blackouts or failures.
Food and Beverage
-
Quality Assurance: Detects contaminants or problems in real-time to ensure food products meet safety and quality standards.
-
Energy Efficiency: Optimizes refrigeration and production with minimal energy consumption.
Logistics and Warehousing
-
Route Optimization: Efficient delivery routes are calculated on the fly to save time and fuel.
-
Inventory Tracking: Uses AI to monitor stock levels and predict when to restock for optimal inventory management.
The Benefits of Edge AI in Industrial Operations
Enhanced Process Efficiency: Real-time insights drive seamless process adjustments, reducing inefficiencies and optimizing output. Factories can respond to operational changes instantly, boosting productivity. Proactive Maintenance: Unplanned downtime disrupts industrial workflows and costs millions annually. Edge AI enables predictive maintenance by analyzing sensor data to anticipate failures, minimizing equipment downtime and repair costs. Improved Product and Service Quality: Continuous data analysis identifies quality issues early, enabling immediate corrective actions and ensuring products meet stringent standards consistently. Streamlined Supply Chains: Creates real-time transparency throughout supply chains, from raw materials to finished goods, improving inventory management and logistics efficiency. Increased Workplace Safety: Safeguards workers by monitoring industrial environments for hazards, ensuring compliance with safety standards and minimizing risks. Cost Optimization: From lowering energy consumption to minimizing waste, Edge AI reduces operational costs while enhancing ROI. Factories also save on cloud data transfer expenses.
Challenges in Edge AI Deployment
Despite its potential, deploying Edge AI in industries comes with challenges:
-
Legacy Systems: Integrating Edge AI with older equipment requires careful planning.
-
Data Silos: Cross-functional data sharing can be limited in some industries.
-
Skill Gaps: Implementing Edge AI requires specialized knowledge.
By collaborating with experienced solution providers, industries can overcome these barriers to unlock the full potential of Edge AI.
The Future of Edge AI in Factories and Beyond
Edge AI is poised to transform industrial ecosystems by enabling smart, autonomous factories that operate with unparalleled efficiency and precision. As these systems evolve, they redefine operations, making industries more resilient and adaptive to global demands.
Industries that embrace Edge AI will:
-
Enhance responsiveness and innovation
-
Meet sustainability and compliance goals
-
Lead the way in a competitive global landscape
Implementing Edge AI at scale requires collaboration and expertise. Businesses should work with experienced partners capable of integrating AI into existing workflows. These partnerships accelerate time-to-value while minimizing risk.
Why Choose XenonStack for Edge AI Solutions?
With the rise of Industry 4.0 and the subsequent proliferation of edge AI, manufacturing operations are being redefined. Edge AI empowers real-time analytics, predictive maintenance, and smarter operations, improving efficiency and driving innovation. When this transformative technology is adopted, industries will be ready to thrive in an ever-changing environment.
XenonStack possesses the experience to develop and deploy such out-of-the-box solutions. These solutions require a team of highly skilled and experienced professionals. XenonStack provides you with a dedicated team to develop customized solutions that help you fulfil your business requirements the way you want.