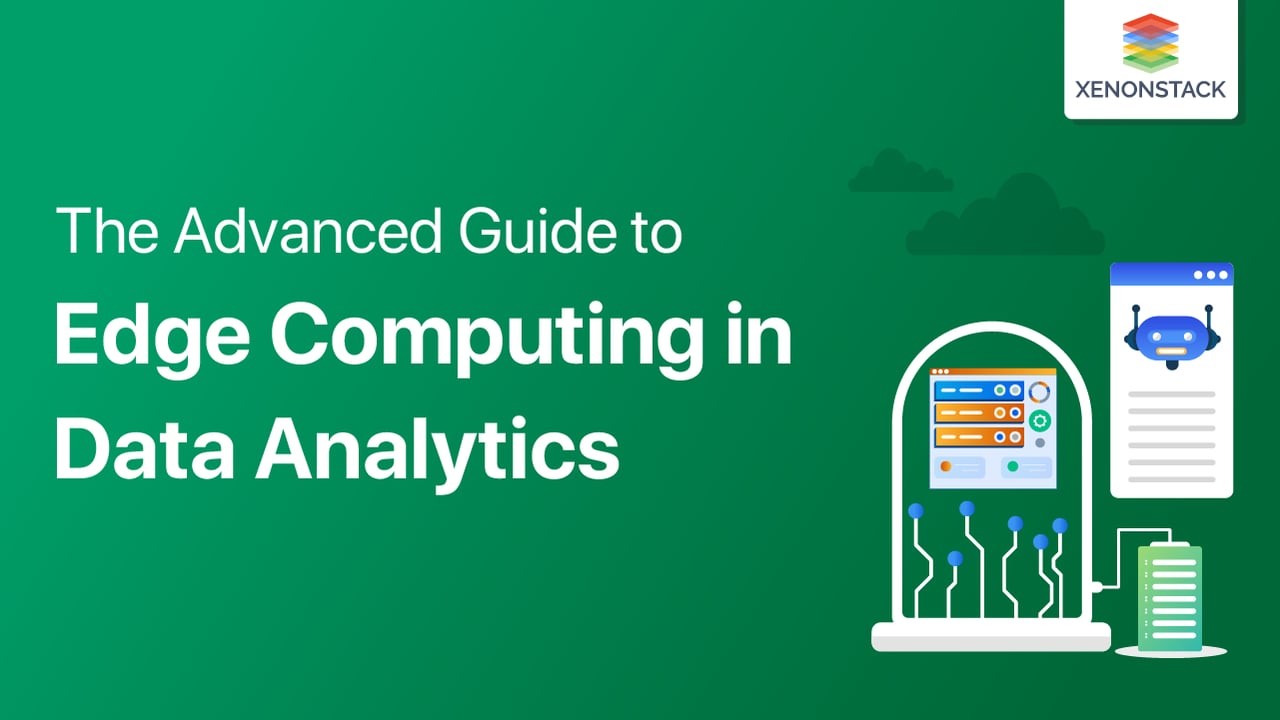
Introduction to Edge Computing Data Analytics
The rising importance of data is one of the most critical side effects of the internet revolution. Advertising, sales, and return on investment metrics have been thrown out in favor of cold hard figures and data that can be acted such as profiles, activities, email addresses, and phone numbers. The data produced from everyday interactions with servers through the IoT can produce insights that can change how businesses go about their processes. In the present scenario, a massive opportunity for businesses to take advantage of the innovations is that kind of market growth and an even more significant opportunity for those who can provide solutions at the edge.
Lots of insights are found on the edge. However, feeding the information back to a cloud platform and then analyzing it is an intensive process. Insights gained from the information on the edge allow reaction in real-time and feed analytic engines that allow decisions on data predicting the future, reducing spending, finding new opportunities, or altering the business model.
Some domains, such as healthcare or industries, promptly observe some parameters by Edge Analytics. It in edge devices execute some analytical algorithms and sends filtered data to the cloud for future or future analysis. So, it has some specialty to give decentralized and quick insights from edge data at the network's edge, in contrast to standard analytical models, the premium on speed and decentralization for traditional extensive data.
IoT Edge Analytic devices have grabbed customers’ attention and became one of the ten most demanding technology. Click to explore about our, IoT Edge Analytics
What is Edge Analytics?
It collects, processes, and analyzes massive data at the network's edge or close to a sensor, network switch, hub, or any other connected device. Nowadays, many devices are connected by the Internet of Things (IoT), and many industries ( retail, manufacturing, transportation, and energy ) generate vast amounts of data on the network's edge.
Why it Edge Analytics important?
Edge analytics offers various vital benefits
- Edge computing reduces the latency of data analytics
In different circumstances, such as Oil rigs, aircraft, and CCTV cameras. In remote manufacturing environments, there may need more time to send data to the central data analytics environment and wait for the outcomes to impact decision-making meaningfully.
- Edge computing helps with the scalability of analytics
Many sensors and network devices are growing, and a large amount of data collect exponentially increases the strain on central data analytics, which process a vast amount of data. Edge computing allows the organization to enhance the scale, processing, and analytics capabilities by decentralizing data collected.
- Edge computing helps the problem of low bandwidth networks
The bandwidth needed for transforming data to collecting by thousands of these edge devices will grow exponentially, increasing the number of these devices. So, it reduces these problems by delivering analytics capabilities in these remote locations. It also reduce overall expenses by minimizing bandwidth, scaling operations, and reducing the latency of critical decisions.
Edge Computing brings data storage and processing close to where data is being generated or gathered. Click to explore about our, Applications of Edge Computing
When should Edge Analytics be considered?
In IoT, it is used for Oil Rigs, Mines, and Factories which operate in low bandwidth, low latency, and richer forms of data such as video analytics. For example, One car carries 150-300 sensors. The current airbus model has nearly 6,000 sensors and generates 2.5 Tb of data daily. In wind turbine farms, the nearest IoT sensors generate a large amount of data daily. They also use edge computing and edge analytics monitors or detect data outliers, detect trends over time, and analyze and archive different aggregations.
An XML-based predictive model interchanges format but predictive Model Markup Language ( PMML ) provides a way to describe and exchange the analytic applications produced by data mining and machine learning algorithms.
In peer-to-peer nodes, communication may be a real possibility over time: IoT sensors are currently deployed through silos. In Edge network offers peer-to-peer communication by the Edge devices if sufficient processing capability.
The most significant promise of it may be its ability to offer energy providers insights about assets at a given time, thereby enhancing the ability to perform predictive maintenance. Maintaining and buying equipment is a significant investment for any company. Repairing or replacing energy-producing assets malfunction can exponentially increase operating expenditures.
In Edge computing, apart from intelligent sensors and connected IIoT devices to collect data, it needs hardware and software platforms to store data, prepare and train the data by the algorithms, and process the algorithms.
The descriptive analytics segment holds the largest market share, owing to the streaming need for descriptive data to enhance predictive analytical models and identify new deployment across numerous places ( construction, industrial, and public places ) that are looking forward to driving the market for historical edge analytics.
The predictive analytics segment is to grow at the highest rate during the forecast period due to the high demand for preventive measures to be taken to avoid dangerous and harmful situations, which affects the growth of its industry.
How to deliver it?
It is a short process task, and it typically takes the analytical model, deploying and executing it at the edge. Many decisions need to be made for collecting data, preparing data, selecting the algorithms, training the algorithms continuously, and deploying/redesigning the models. The storage capacity plays a key role, including decentralized and peer-to-peer deployment models with pros and cons for each.
How is it different than regular analytics?
It have similar capabilities as regular analytics, except for the various situations where the analysis is performed. The main difference is that its applications need to work on edge devices with more memory, processing power, or communication impediment.
What are the Use Cases of Edge Analytics?
The edge analytics use cases are described below:
Retail Customer Behavior Analysis
Retailers can collect data from various sensors, including car parking lot sensors, shopping cart tags, multiple store cameras, and CCTV. By applying analytics to the collection from these types of devices, retailers can offer personalized solutions with the help of behavioral targeting analytics.
Remote Monitoring and Maintenance for various Industries
Today's industries, such as energy and manufacturing, need instant response when machines fail to work or require maintenance. Without centralized data, analytics organizations can identify the failure faster and take action before it can arise within the system.
Smart Surveillance
Businesses can benefit from real-time intruder detection edge services for security. From security cameras, by collecting raw images, we can detect and track any auspicious activity through edge analytics.
What are best Tools for Edge Analytics?
Some edge analytics tools are:
- AWS IoT Greengrass
- Cisco SmartAdvisor
- Dell Statistica
- Microsoft Azure IoT Edge
- Inter IoT Developer Kit
- Oracle Edge Analytics (OEA)
- IBM Watson IoT Edge Analytics
What are the Benefits of Edge Analytics?
It has received more attention with the Internet of Things (IoT) spread. For all businesses, streaming data from disparate IoT sources creates a massive amount of data which tough to manage. The analytics algorithm filters all data created at the network's edge parameters to decide whether it is worth migrating to the cloud or data store.
Analyzing data generated decreases latency in the decision-making process as better. If an IoT component of a system suffers a failure, the algorithm interprets that data and automatically shuts it down. This saves lots of time transporting data to a centralized store, reducing or avoiding equipment downtime.
Its algorithms are applied to sensors and devices that relieve the hassle of management and analytics systems, anyway. The number of connected devices and the network size allow the system to enhance quickly and easily, no matter how much the data grows.
What are the limitations of Edge Analytics?
It is a relatively new and trending technology. Not all hardware is capable of storing data or performing complex processing.
Businesses also consider whether or not it makes sense to invest in it, best suited for scenarios that need to minimize speed, security, or efficiency. With new technology and architectures, some engineering obstacles remain to deploying an edge application successfully.
Conclusion
As far as Edge Analytics is an exciting area. Every organization in the Industrial Of Things (IIOT) is increasing yearly investments. Many famous companies are aggressively investing in this technology in specific segments such as retail, manufacturing, energy, and logistics that deliver the quantifiable business benefits by reducing the latency of decisions, solving the bandwidth problem, and potentially reducing expenses.
- Discover more about EdgeOps Platform Benefits and Working
- Click to read about Edge Computing vs Cloud Computing