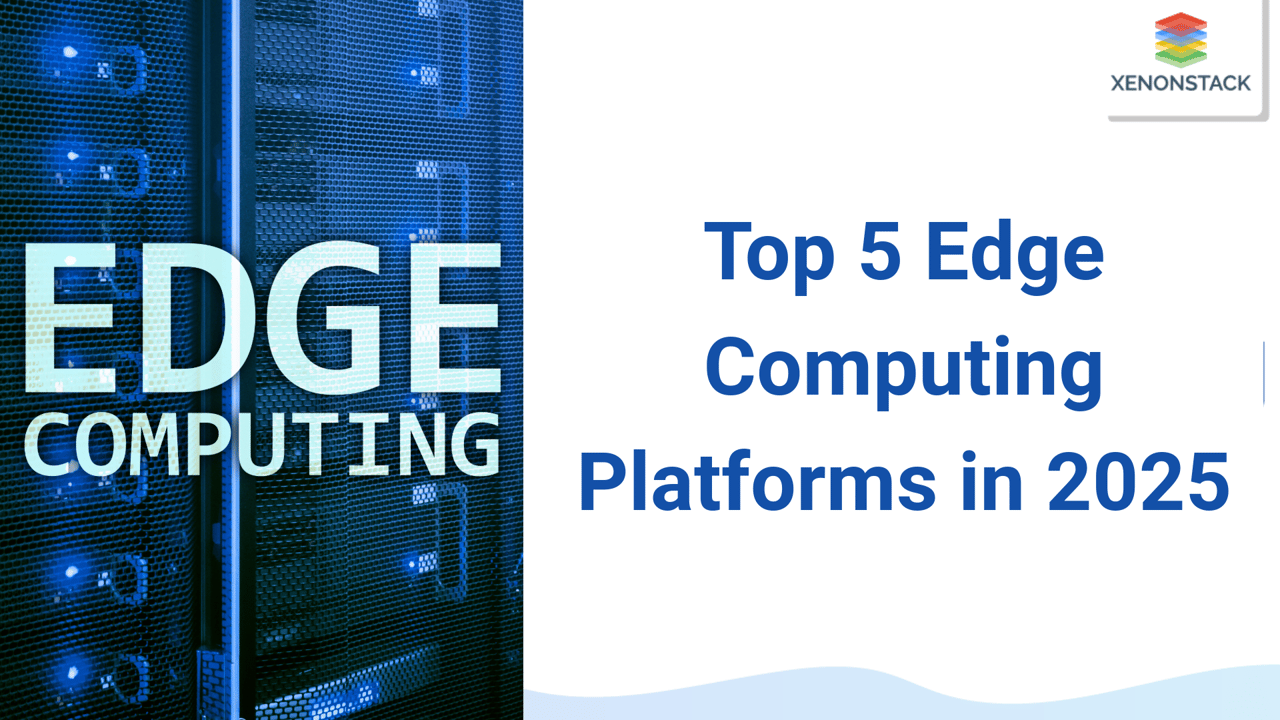
Introduction to Edge Computing Platform
Nowadays, with the arrival of 5G fast wireless, placing compute storage and deployments of Internet of Things (IoT) analytics close to where the data is created makes a case for edge computing. Expanding Internet of Things devices and new applications requires real-time computing power. 5G wireless is considering edge systems to fast-track the support of real-time applications or creations like AI, analytics, robotics, self-driving cars, and many more. Initially, the goal is to address the cost of high frequency for data moving over long distances because of the extension of IoT-generated data.
Edge Systems have opened up opportunities to take a fresh and practical approach to data processing and fuel a range of technology-driven solutions. Click to explore our, Edge Computing Architecture
What is Edge Computing?
It is a networking ideology focusing on conducting computing as close to the origin of data as possible to decrease bandwidth and latency use. Conducting computation to the network's edge decreases the amount of long-distance communication that has to happen between a server and a client. A shared computing framework brings venture applications closer to data sources like local edge or IoT devices. The presence of data at its source can have strong business benefits, improved response times, faster insights, and better high-frequency availability.
Why do we need Edge Computing?
It helps undertake high frequency, addressing cost and latency issues across many IoT applications. Here are the three key reasons why there is a need for edge computing:
Reduce the Signal to Noise Ratio
It helps reduce the signal-to-noise ratio, allowing companies to prioritize their data. For example, they can focus on crucial data that needs to be processed, stored, and analyzed shortly. For example, they can monitor a refrigeration unit. The data collected is machine-dominated, and machine-generated data is okay at measuring state data. From time to time, the machine generates an event. For example, it will generate an impression that it is not okay, so here, the observing company will care about this. Everything else will be noise data. It helps classify the data that needs attention.
Reduce the amount of Data Stored and Transmitted in the cloud
Nowadays, the amount of data continuously produced at the edge is growing faster than networks' potential to process it. Rather than sending data to a remote data centre or the cloud to do the work, sending it to endpoints should communicate data to an Edge computing device to process that data. The goal is to decrease latency and cost while governing the network bandwidth. An advantage is that it reduces the data stored and transmitted in the cloud.
Reduce the lag time in Processing/Transmission
Edge computing lowers the lag between the processing, transmission, and the action required at the end. Event processing and analysis can be done desperately and cost-effectively because much of the source data does not need to be operating in the cloud to be analyzed and processed. Cloud data centres can be thousands of distances far away from any connected device. The particular kinds of intermission for autonomous vehicles, precision manufacturing, and robotics surgery have a relative lifetime. This cycle can be reduced to just a few milliseconds with Edge Systems.
Best Edge Computing Platforms of 2025
Platforms can be established for an extensive range of use cases, such as healthcare, for making clinical decisions in industrial environments like mining, manufacturing, and telecom. These platforms can be either open-source or paid, and the selection of platforms should depend on the organization. Here are the top 5 edge computing platforms.
Azure IoT Edge
Azure IoT Edge is part of Microsoft's intelligent cloud-to-edge solution suite. It allows you to securely and remotely manage and deploy cloud-native workloads, such as Azure services and AI, to run directly on your IoT device.
What are the features of Azure IoT Edge?
The features of Azure IoT Edge are described below:
-
IoT Enablement: The Azure IoT Edge platform is built for IoT applications and can be capitalized alongside containers, certified IoT hardware, and Azure Stream Analytics.
-
Analytics Insights: The Microsoft Azure Admin Center comprehensively understands Edge operations.
-
Edge security: Azure IoT Edge platform is unified with Azure Defender for IoT, and Edge devices can be casually monitored.
-
Cloud to Edge Infrastructure: One can obtain Azure's IoT Hub, which supports architecture provisioning and Zero-touch devices.
Eclipse fog
This platform is a complete edge computing platform that provides all of the pieces needed to run and build applications at an edge at an enterprise scale. It is an integrated development environment backed by IBM and built by the Eclipse Foundation.
What are the features of Eclipse ioFog?
-
IoT enablement: It can be adjusted to various use cases through Kubernetes development.
-
Analytics insights: The controller hub gives you analytics insight and remote visibility.
-
Edge security: It has an open infrastructure, which means it has properties. You can connect it with third-party security services.
-
Cloud-to-edge infrastructure: Eclipse ioFog lets you connect cloud infrastructure to the edge using built-in connectors.
ClearBlade
ClearBlade provides edge computing software for expensive IoT applications in industrial environments. It is an Austin-based company that was founded in 2007.
Best features of ClearBlade
The features of ClearBlade are described below:
-
IoT enablement: It is adaptable to most IoT devices, protocols(ZeroMQ, ZigBee, BlueTooth), and IoT systems
-
Analytics insights: From these insights, one can easily stream and filter the data at the edge and obtain acumen from the consolidated edge platform.
-
Edge security: In Analytics insights, edge access is safe through multiple authorizations, authentication, and encryption layers.
-
Cloud-to-edge infrastructure carries code portability, which can be expanded in the cloud and directed to the edge.
Edge AI will allow real-time operations, including data creation, decision, and action where milliseconds matter. Click to explore How to Implement Edge AI?
Alef private Edge Platform
Alef private Edge Platform gives edge connectivity products for healthcare, governments, the industrial sector, and education. It was founded in 2009 by a New York-based edge computing company.
The features of Alef's private Edge Platform
-
IoT Enablement: Alef Private Edge Platform powers a combination of ideal for running IoT devices, 5G, and edge network connectivity.
-
Analytics Insights: Alef's private Edge Platform allows one to manage the environment from the perspective of operational analytics and a centralized platform.
-
Edge Security: In this platform, one can easily control to reduce the risk of exposure.
-
Cloud to Edge Infrastructure: This platform makes deploying edge architecture from the cloud easy without understanding 3GPP standards.
Google Distributed Cloud Edge
Google Distributed Cloud Edge is a completely managed product that brings service and Google Cloud infrastructure. It was Launched in 2021 as part of the Google Distributed Cloud suite.
Best features of Google Distributed Cloud Edge
The following are the best features of Google Distributed Cloud Edge:
-
IoT Enablement: In this Google Distributed Cloud Edge platform, one can purchase serverless infrastructure and containers to run on IoT applications.
-
Analytics Insights: Google's AI data analytics solutions help gather data insights and enable edge observability in these databases.
-
Edge Security: It accommodates privacy and security requirements and can be combined with third-party providers.
-
Cloud to Edge Infrastructure: This platform allows one to transition from the edge using Google Cloud architecture services easily and from on-premise to the cloud.
What are the benefits of Edge Computing?
Cost savings
It helps minimize server resources and bandwidth use. Cloud resources and Bandwidth are expensive and finite. Nowadays, every office and household is enhanced with thermostats, smart cameras, printers, and toasters. Statista predicts that by 2025, there will be over 75 billion IoT devices installed worldwide.
Performance
Another remarkable benefit of moving processes to the edge is to lower the discontinuation. Every time a device needs to communicate with another server, that creates a delay. If this process is conducted to the edge and the router is in charge of moving intra-office chats, this delay would not exist anymore. It is the same as when users of all web applications run into processes that have to communicate with an external server; they will experience delays. So, these delays can be escaped by bringing more processes to the network edge.
New functionality
Additionally, edge computing can develop new functionality that wasn't previously available. An organization can use it to analyze and process its data at the edge, making it feasible to do so in real-time.
Challenges of Edge computing
-
One main drawback is that it can grow attack vectors. With the extension of more smart devices, like IoT devices and edge servers with built-in solid computers, there are new events for malevolent attackers to arrange these devices.
-
The second drawback is that it requires more local hardware. For example, while an IoT camera needs a built-in computer to address its raw video data to a web server, it would need a much more refined computer with more processing power to run its motion detection algorithm. However, the reduced hardware cost makes building smarter devices expensive.
AI Edge Computing Ecosystems
An ecosystem in AI edge computing may be defined as the collection of platforms, devices, and services that support AI implementation. Some leading ecosystems are unity between the hardware creator, software developer, and cloud solution.
Some of the existing AI Edge Computing Platforms
NVIDIA Jetson
Overview: An end-to-end approach for deploying AI methods on specially designed computer hardware and software.
Features
-
Compatible with TensorFlow and PyTorch.
-
Information to be pre-trained models as well as transfer learning.
Use Case: NVIDIA Jetson-based self-organizing drones with real-time navigation and obstacle detection.
Google Coral
Overview: A site providing edge-specific hardware boosters and solutions.
Features
-
Compatibility with TensorFlow Lite.
-
Low-power TPUs for edge execution of AI tasks.
Use Case: Coral devices can be used for retail analytics, such as tracking the number of people in a store.
AWS IoT Greengrass
Overview: An adaptive runtime corresponding to AWS that brings computing extension to devices.
Features
-
This supports deploying AI models to target devices and managing the latter.
-
Secure operation integration from cloud to edge.
Use Case: They include industrial IoT for predictive maintenance in factories as their type.
Intel OpenVINO
Overview: A suite of software tools created to maximize and operationalize AI models to the edge.
Features
-
Practical methods and technology in model size reduction.
-
BMC offers hardware support in the form of Intel processors and accelerator information.
Use Case: Real-time diagnosis of high-quality healthcare imaging solutions employing Open VINO.
The adoption of cloud computing has brought data analysis to a new level. Cloud connectivity has enabled the most accurate way to capture and analyze data. Still, things are now working very well with a computer on edge. As a result, the level of business activity has become huge. Edge computing is an effective solution for data-driven tasks that require fast results and a high degree of flexibility, depending on the current situation.
Next Steps with Edge Computing Platforms
Ready to explore the future of real-time data processing with edge computing? Learn how this innovative solution can reduce latency and improve efficiency. Our expert is here to guide you through the next steps—let’s connect and discuss!