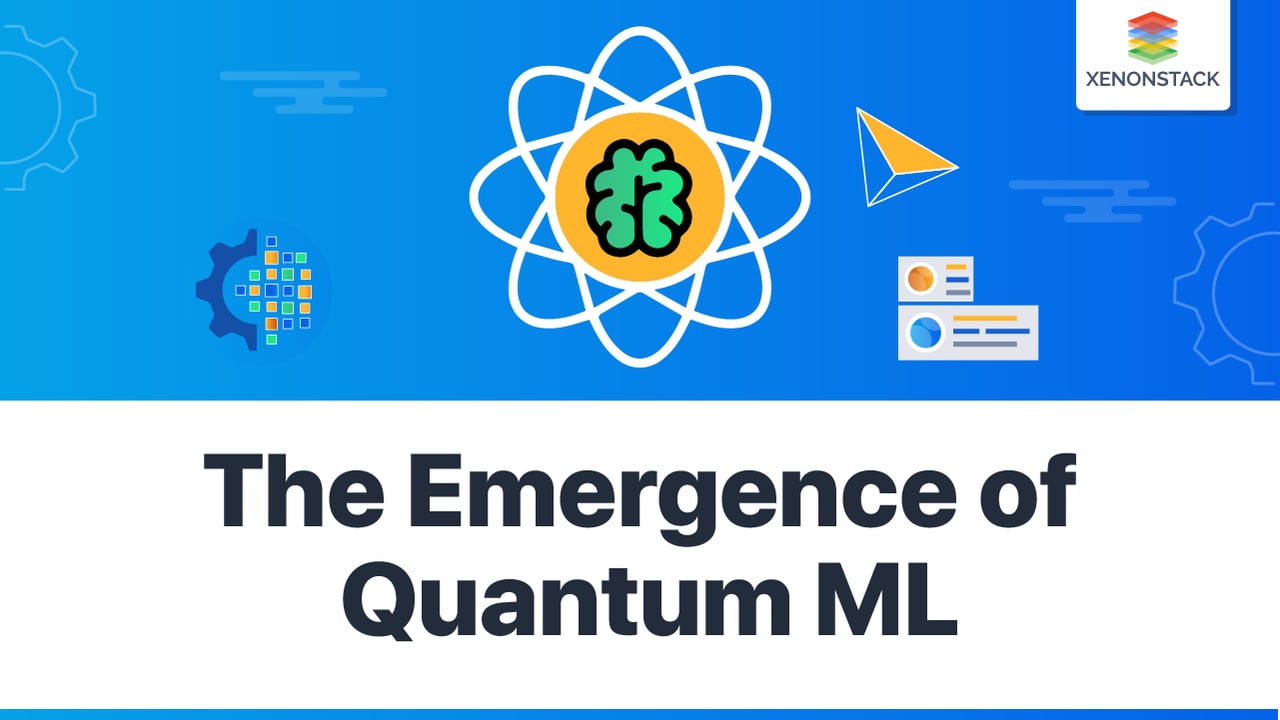
Classical vs Quantum ML: Key Differences
Classical machine learning algorithms are based on mathematical optimization and probability theory and train models that can make predictions or decisions based on input data. Examples include decision trees, support vector machines, and neural networks. These algorithms search for patterns in the data and use these patterns to make predictions. Quantum machine learning algorithms, on the other hand, are based on quantum mechanics and are used to train models that can make predictions or decisions based on quantum states.
These algorithms take advantage of the unique properties of quantum mechanics, such as superposition and entanglement, to perform impossible calculations with classical algorithms. Examples of quantum machine learning algorithms include quantum neural networks, quantum support vector machines, and quantum principal component analysis. Overall, the main difference between classical and quantum machine learning algorithms is the underlying mathematical framework they are based on and the types of calculations they can perform. While classical algorithms are based on classical physics and are limited by the computational resources available, quantum algorithms are based on quantum physics and can potentially perform certain calculations exponentially faster than classical algorithms.
Challenges in Quantum ML
Quantum machine learning (QML) is a relatively new field that combines the power of quantum computing with machine learning capabilities. However, several obstacles and limitations are currently impeding the development and practical application of QML.
Lack of large-scale quantum computers
The main challenge for QML is the need for large-scale quantum computers. Current quantum computers are in their early stages of development and can handle only a limited number of qubits (quantum bits). As a result, most QML algorithms can only be executed on small-scale quantum computers, limiting their practical applications.Lack of understanding of quantum algorithms
QML is based on quantum algorithms, which still need to be discovered. This makes it difficult to design and develop efficient QML algorithms that fully exploit quantum computing's unique properties.- Noisy quantum devices
Quantum devices are extremely sensitive to noise and errors, which can lead to errors in QML algorithm results. The "noise problem" is one of quantum computing's most significant limitations.Limited availability of quantum software and libraries
There needs to be more quantum software and libraries for QML; implementing QML algorithms is difficult for researchers and practitioners.Lack of standardization
QML is still in its early stages, and the algorithms and techniques must be more standardized. This makes comparing and evaluating different QML approaches difficult.Despite these obstacles and limitations, QML remains an active area of research, with numerous ongoing efforts to overcome them. As large-scale quantum computers are developed and our understanding of quantum algorithms grows, QML is likely to become a more practical and powerful tool for solving complex problems in the future.
Quantum ML in Industry
Various industries, including finance, healthcare, and energy, can benefit from quantum computing. Some specific use cases of quantum computing in the enterprise include:
-
Drug Discovery: Molecular simulations with quantum computing can speed up the discovery of new drugs.
-
Financial modelling: Using quantum computing to solve complex financial models and optimize portfolios is possible.
-
Supply Chain Optimization: Quantum computing can optimize logistics and transportation in supply chain management.
-
Cybersecurity: Using quantum computing, new, more secure encryption technologies can be developed and used to break prevalent encryptions.
-
Energy: Quantum computing can help reduce environmental impact by optimizing the design and operation of energy systems like power grids and batteries.
-
Machine Learning: Quantum computing can train and run machine learning models on massive data.
Quantum ML: Key Insights
Data availability profoundly changes the question when assessing the capabilities of quantum computing to aid in machine learning. In our work, we create a practical set of tools for investigating these concerns, which we then use to create a new projected quantum kernel method with several advantages over prior approaches. We progress towards the largest numerical demonstration of potential learning advantages for quantum embeddings to date, 30 qubits. While a comprehensive computational advantage in a real-world application needs to be observed, this research lays the groundwork for the future. We invite any interested readers to read the paper as well as the accompanying TensorFlow-Quantum tutorials, which make it simple to build on this work.
Machine Learning pipeline Microsoft Azure machine learning services Machine learning tools