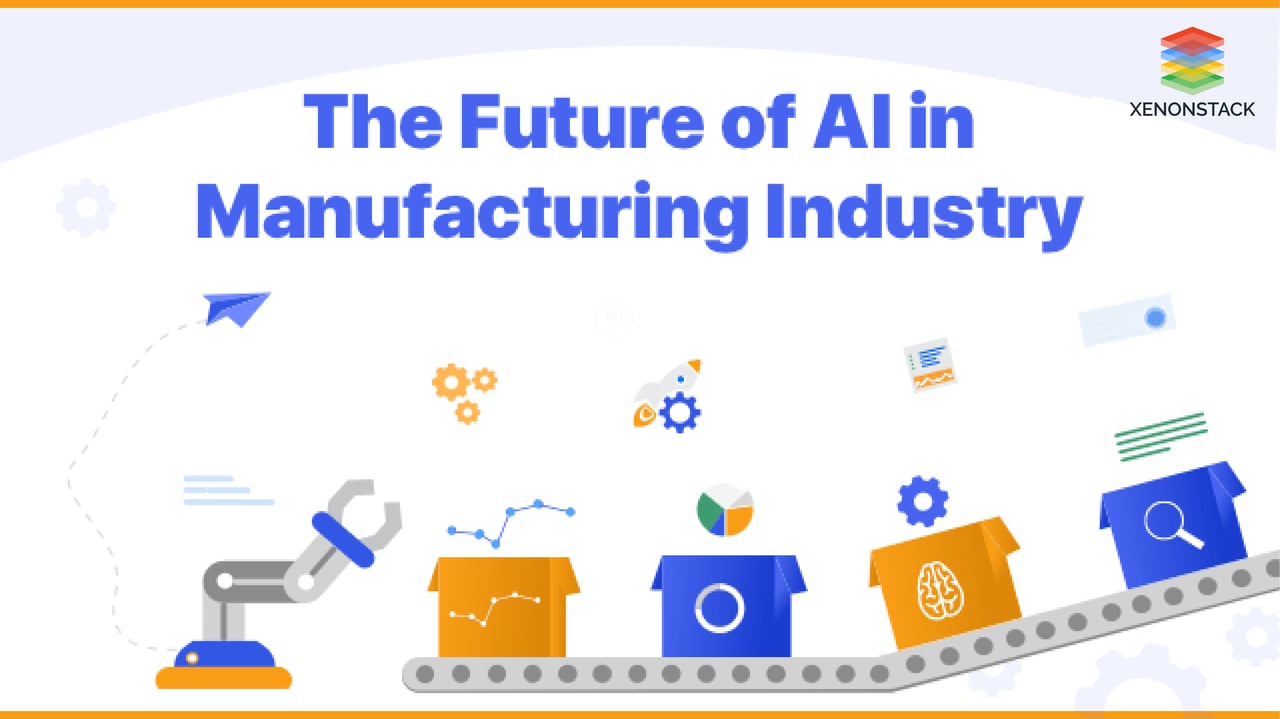
Introducing Explainable AI
Manufacturers are using AI (Artificial Intelligence) to provide high-quality services and products. Explainable Artificial Intelligence in manufacturing improves efficiency, workplace safety, and customer satisfaction by automating tasks. The manufacturing industry uses Explainable Artificial Intelligence in its applications, such as Predictive Maintenance, anomaly detection, real-time quality monitoring, and supply chain optimization. But implementing AI in the manufacturing industry is a challenging process.
What are the Challenges of Implementing AI?
The challenges of Implementing AI in the manufacturing industry are listed below:
- Collect correct data.
- Justifying the working model process.
- Justifying and validating the generated output of the system.
- Strategies Implementation.
- Computing Power.
Most AI systems use black-box approaches to get accurate and correct results. But due to the complexity and less transparency in the systems, it has a lack of accountability in the decision-making process.
The solution to this opaque nature of models is a white-box model. White-box models generate transparency and empower the developer and Customer to execute complex projects with confidence and certainty.
White-box models provide clear explanations of their behavior, such as
- How do they produce predictions?
- What variables influenced the model?
- Is the decision made by the system correctly or not?
Due to their transparent inner-working model process becomes easily interpretable.
Explainability is essential in the manufacturing industry. White box models provide significant benefits. It allows them to build the trust of their customers.
Artificial Intelligence can help create machines to perform specific tasks that normally require human intelligence. Click to explore our, AI Adoption Challenges and Solutions
Why is Explainable AI in Manufacturing Industry Necessary?
The need for Explainable Artificial Intelligence in manufacturing by a case study of Predictive Maintenance (PdM) scenario for manufacturing. This process can save a lot of money and time by reducing and predicting machine breakdown. It can predict machine errors or tool failures.
"A factory loses between 5% and 20% of its manufacturing capacity due to downtime,"-According to the International Society of Automation."
Traditionally machines are required to be repaired after a particular interval of time or usage for preventive maintenance processes. However, it still results in significant equipment failure instances resulting in idle workers, lost revenues, and customer trust loss. Besides, it may replace parts that still have a significant working life, wasting time and money.
AI-based Predictive Maintenance uses various data and determines which components should be replaced before they break down. Models can look for patterns in data that indicate failure modes for specific components. Thus it generates more accurate predictions of the lifespan of a component. When specific failure signals are observed or component aging criteria are met, the components can then be replaced during scheduled maintenance.
This replacement and maintenance cost is high. Therefore, it is necessary that the System would provide correct and reasonable results. Therefore Explainable AI is required to know the mistakes that the System can make and the safety measures.
Principles of Explainable AI in Manufacturing
To implement Explainable Artificial Intelligence in our AI system, it should obey four principles of Explainable Artificial Intelligence. These are listed below in brief:
Explanation
According to this principle, the System will provide a reason for each decision. The predictive maintenance system will tell that an error or tool failure will occur and need maintenance or equipment replacement. It costs a lot for maintenance and replacement. Their customer can query whether the outcome that is generated by the System is correct or not. In explanation, the System will focus on the three major questions: these three factors help to create the output.
- What algorithm is being used?
- How does the model work?
- Which inputs or parameters of the data are taking part in determining an output?
These questions help to understand whether the System is working correctly or not. So they can make decisions about whether they will use that System or not. They can also give the reason for the output.
Meaningful
The explanation that the system provides is meaningful. Information will be understandable by the targeted user. The System provides different explanations for different user groups, for the end-user and the developers, according to their prior knowledge and experience. If a user can understand the information, it means it is meaningful. The explanation that the System provides should be understandable to the targeted recipient. For example, the System explains the model's inner workings and algorithm to debug the System easily. And also understand whether it works correctly or not. But for the developer, they require how the System reaches its decision, so for them, justification of the model through SHAP can be provided. That can gain their trust in the System.
Explanation Accuracy
This principle states that explanations should be accurate. Our System explains the same procedure the AI system used to generate output. Because if it generates the wrong output, then it is of no use. False justification can lose a customer's trust and reduce revenue by making wrong decisions and actions. We should use the correct tool and the correct way to represent the explanation of the System.
Knowledge Limits
Knowledge limits prevent the System from giving an unjust and fallacious result. Hence users can assure that the System will never mislead. It will merely show the outcome that will be set for the System. For example, it may be possible that the System will get a different feature or scenario rather than giving false results about which System should tell that this data is wrong. Handling this System can be designed as if the System encounters a different situation and can generate results "out of the topic case."
The process of using production management software or RPA tools to operate manufacturing. Taken From Article, Manufacturing Process Automation
Benefits of Explainable AI in the Manufacturing Industry
- It bridges the transparency and trust gap between AI technology and its consumers.
- Its tools and techniques allow customers to get justifications for their results in a format that humans can understand. Hence they can understand the rationale behind each decision. Thus it makes this easy to validate automated decisions.
- Manufacturing industries can improve their operational efficiencies and provide better and cheaper services through AI - this is only possible if they have enough trust in the models.
- Transparent AI systems allow ML models to be debugged. They can check the logic and fairness of data, which leads to better development of ML models.
- Its tools and techniques advance the manufacturing industry services by building humanly interpretable, trustworthy, and effective AI systems.
How Explainable AI Transforms Manufacturing Operations?
- Predictability, Agility, Resiliency
Developed Manufacturing processes reduce operational complexity, cost, and risks and maintain continuity in the business processes. - Transparency and visibility
Reduce disruption by providing complete transparency of the system. - Deliver Customer Promises
Preserve customer promises by solving their doubts and query using Explainable AI. - Manufacturing Efficiency
Production efficiency is essential for the manufacturer. An increase in production efficiency produces more goods in less time and also helps to increase revenue.
Deployment of ML models by collecting data from sensors can develop a robust AI that can alert when any equipment's performance degrades and needs maintenance. Hence it can reduce machine downtime and improve its efficiency. - AI-driven insights
ML model helps align the products to customer interest by understanding customer behavior patterns, product recall data, and demands. AI provides a granular view of the products they manufacture. - Customer Interaction
A human-friendly approach to explaining the model makes customer interaction better. Akira AI uses dashboards to justify the model output and model working. Thus it makes it easy for the Customer to understand it. - Improve the Customer's journey
Customers get frustrated when the system cannot justify the output. But hassle-free services with Explainable AI enhance Customers' journeys. Local and Global Explanation allows for justifying the particular output as well as the model's performance.
What are the Features of AI in the Manufacturing Industry?
- Customer Satisfaction: It is necessary to provide customer satisfaction to improve the customer experience. Companies must deliver proactive, seamless, and personalized services to their customers to enhance their experience.
- Trust: Explanation is required for the decision-maker to trust the system. The degree of confidence in the system to decide and the factors that influence its decision must be explained. A completely transparent system can place the Customer's faith in their system. It is hard to provide such transparency in opaque models, but some can use alternatives to clarify them.
- Fairness: Fairness is a must to remove the bias in the system. It is a central requirement of the system. Most of the time, the system would be biased due to an improper dataset. It should be evaluated to check fairness. Various measures can be used to make a fair dataset, such as resampling and calibration.
- Transparency: Transparency is a major feature that Explainable AI provides. The transparent nature of the AI system helps to understand that and also trust in that.
What needs to be explained?
Justification of the model's output is not enough to solve all customer's queries. It is required to explain every factor of the AI system.
Data: It explains the data collected from IoT sensors embedded in machine equipment, machine operations, environment, etc., used in the Manufacturing industry's AI systems, their correlation, and EDA (Exploratory Data Analysis) to understand the hidden data patterns behind the data. It tells how the data is to be used for the AI system.
Algorithm: The System explains the algorithm used for the System and its comparison to other algorithms.
Model: It provides a detailed explanation of model performance and works in a user-friendly manner so that customers can track the performance of their systems.
Output: Justifies the System's decision, such as predictive maintenance, that the System says that component 1 of a particular machine needs to be replaced.
A process to address potential issues before leading to breakdowns in operations, processes, services, or systems with AI. Taken From Article, Explainable AI for Predictive Maintenance
How does XenonStack help Manufacturing Industry with Explainable AI?
The way in which XenonStack help Manufacturing sector with Explainable AI are:
Predictive Maintenance
AI systems can predict equipment failure signs well before they happen using data such as electrical current, vibration, and sound generated by manufacturing equipment. It helps to improve the efficiency of the maintenance process and reduce overall maintenance costs and time.
Demand Forecast
AI systems can predict future sales more accurately than traditional forecast methods. Using market data, product data, and sales trends can predict sales in the market and then plan things accordingly. AI systems make this process more efficient and productive.
Quality Management
Using the data on materials, production status, and other environmental variables, the quality of products can be modeled. AI systems can reduce the cost of inspection by reducing the lead time and cost of inspections by inspecting only higher-risk areas. Akira AI provides dashboards to track the factors detected much earlier, improving the overall yield.
Supply Chain optimization
Machine learning makes it possible for manufacturers to provide more accurate capacity planning, productivity, high quality, lower costs, and greater output. This process ensures the arrangement of raw materials for manufacturing plants in the least amount of time possible in the most cost-effective manner. Then delivered, the finished product timely. AI makes this process reliable and better using ML. It helps to make it fast and traceable.
Yield Production
Yield production directly impacts the manufacturer's performance. AI systems can help the manufacturer to improve the yield production system. It can predict the changes in the output by changes in the temperature, equipment, raw materials, etc. Manufacturers can understand the differences and track the reason behind this change and make further decisions according to those changes.
Conclusion
Akira AI helps increase revenue growth, innovation, and operations excellence by implementing AI in manufacturing companies. Akira AI provides a unique combination of SHAP, IME, PDP/ICE, Anchors, and Rules extraction methodologies in interactive dashboards to explain the models.
- Read here about Enable Artificial Intelligence in Retail
- Discover here Responsible AI Tools and Framework