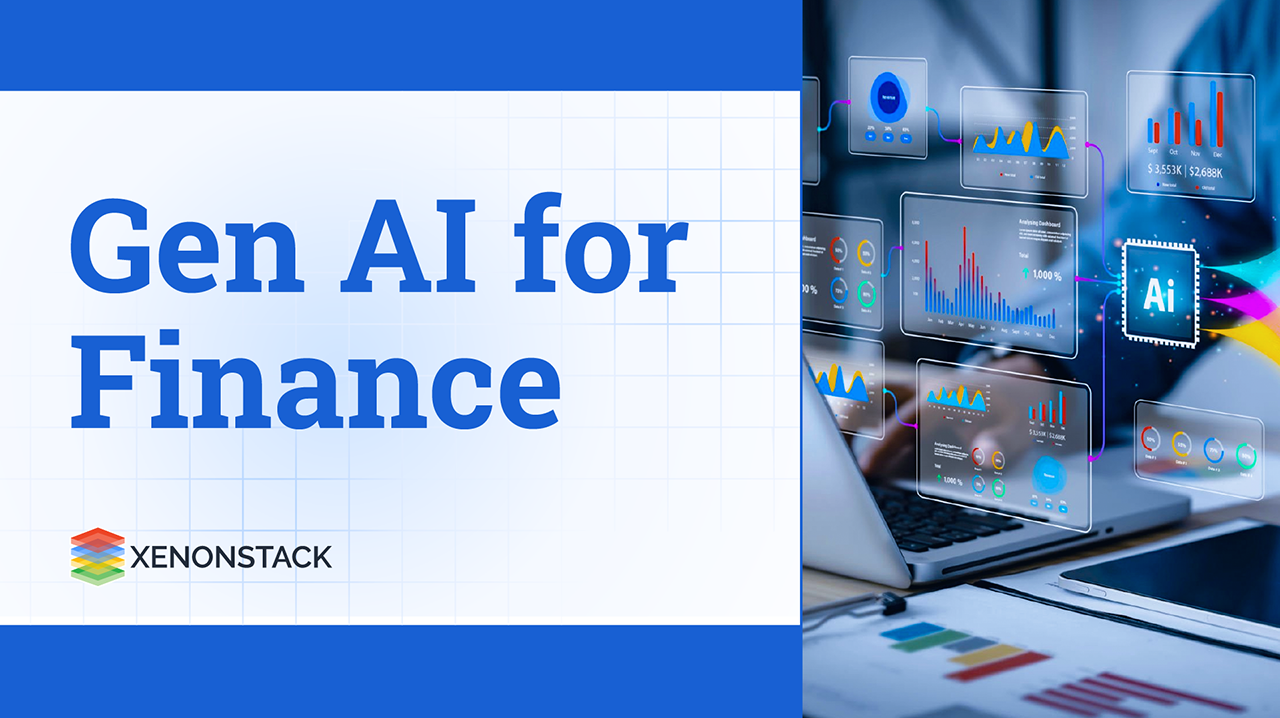
RL in Quantitative Finance
In quantitative Finance, RL is beneficial for:
- Developing Trading Strategies: RL agents can learn to execute trades by observing market conditions and adjusting strategies to maximize returns.
- Portfolio Management: RL optimizes the allocation of assets in a portfolio, dynamically adjusting to market changes and investor goals.
- Risk Management: RL models can predict and manage risks by learning from historical market data and current trends.
Fundamental Techniques in RL
- Q-Learning: An off-policy RL technique that helps understand the value of taking certain actions in specific states, aiding in decision-making for trading strategies.
- Policy Gradient Methods: These methods optimize the policy directly to improve expected rewards, making them suitable for continuous decision-making processes in portfolio management.
- Deep Reinforcement Learning (DRL) combines neural networks with RL, allowing for handling high-dimensional data and complex financial models.
Inverse Reinforcement Learning (IRL) as a Framework to Explain Asset Allocation Decisions
Understanding Inverse Reinforcement Learning
Inverse Reinforcement Learning (IRL) is a process where the reward function is not predefined but inferred from observed behaviour. This technique is precious in understanding the decision-making processes of experts in each field. By analyzing the actions of experts, IRL provides insights into why certain decisions are made under specific circumstances, effectively revealing the underlying motivations or objectives guiding those decisions.
For instance, consider an expert driver driving a vehicle through heavy traffic. We can analyze this driver's actions (such as accelerating, braking, or steering at precise moments) to infer their priorities (such as safety, speed, or fuel efficiency) through IRL. By modelling these priorities as a reward function, we can gain deeper insight into the decision-making process involved in expert driving. This inferred reward function can then be applied to develop autonomous driving systems that emulate human-like decision-making patterns, enhancing their performance and safety in real-world scenarios.
IRL in Finance
In the context of Finance, IRL can:
- Explain Expert Decisions: By analyzing the actions of successful investors, IRL can deduce the underlying principles and preferences driving asset allocation decisions.
- Enhance Transparency: IRL explains their rationale and helps make automated investment strategies more transparent.
- Improve Strategies: Insights gained from IRL can refine existing strategies, aligning them more closely with proven successful practices.
Techniques in IRL
- Behavior Cloning: Mimics the behaviour of experts to learn the underlying strategy.
- Bayesian IRL: Uses probabilistic methods to incorporate prior knowledge and make inferences about the reward function.
- Maximum Entropy IRL: Ensures the most unbiased explanation for observed behaviors, maintaining consistency with known constraints.
Combining RL and IRL for Better Asset Allocation and Portfolio Optimization
- Integrated Approach: Financial institutions can develop and refine robust asset allocation strategies by combining RL and IRL. Here's how:
- RL-Based Strategy Development: RL can be used to create a base strategy that adapts to market conditions and maximizes returns. The agent learns through continuous interaction with market data and improves over time.
- IRL-Based Strategy Refinement: IRL analyzes the behaviour of successful investors to understand the reward functions that drive their decisions. This information is used to fine-tune the RL-developed strategies, ensuring they are aligned with proven effective practices.
- Hybrid Strategy Optimization: The integration of RL and IRL leads to adaptive and explainable hybrid strategies. This combination enhances performance, better manages risk, and aligns with real-world successful behavior's.
Benefits of Combining RL and IRL
- Enhanced Performance: More practical and robust asset allocation strategies.
- Risk Management: Better risk assessment and mitigation.
- Transparency: Improved transparency and understanding of automated decisions.
- Strategic Alignment: Strategies that reflect successful real-world practices.
Practical Implications and Case Studies
- Algorithmic Trading: RL algorithms are already being used in algorithmic Trading. They adapt to market dynamics and outperform static strategies. Case studies show significant improvements in trading performance and efficiency.
- Portfolio Management: Companies like Betterment and Wealthfront are exploring RL to optimize portfolio management, enhance asset allocation, and maximize returns while managing risks.
- Robo-Advisors: Robo-advisors leverage IRL to enhance their decision-making frameworks, learning from human financial advisors to offer more personalized and practical investment advice.
Conclusion
Integrating Reinforcement Learning (RL) and Inverse Reinforcement Learning (IRL) in asset allocation and wealth management represents a significant advancement in quantitative Finance. Financial institutions can achieve more effective, transparent, and robust investment strategies by developing adaptive strategies through RL and refining them with insights from IRL. As technology continues to evolve, the combination of RL and IRL will likely become a cornerstone of advanced financial decision-making, driving innovation and efficiency in the industry.
- Explore Role of Generative AI To Generate Synthetic Data
- Know more about Benefits of Generative AI For Industries