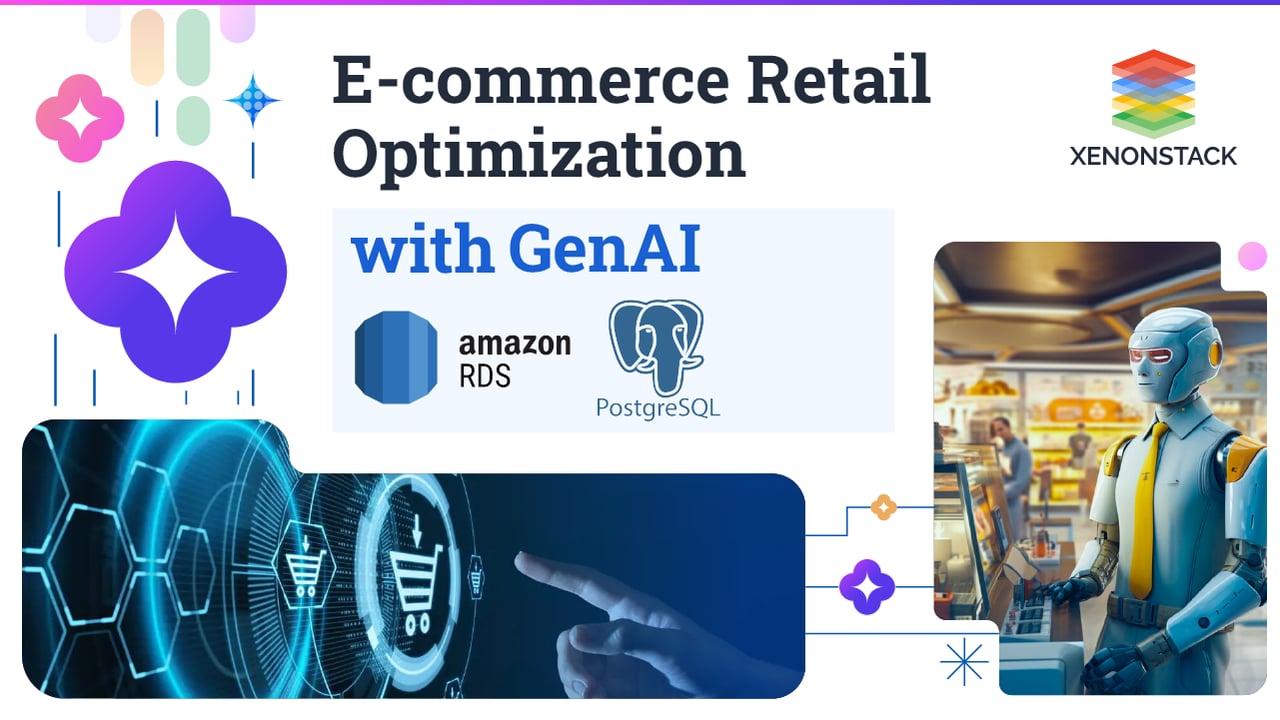
Introduction
The digital age has sparked a notable shift in our lifestyles, establishing e-commerce as a crucial element of our everyday activities. With countless online transactions occurring daily, the amount of data generated by e-commerce platforms is skyrocketing. This surge in data necessitates implementing advanced recommendation systems to cater to individual preferences effectively. Fortunately, the emergence of Generative AI (GenAI) and cutting-edge database technologies such as Amazon RDS for PostgreSQL provide e-commerce retailers with a game-changing arsenal to completely transform their recommendation systems.
This blog delves into GenAI and Amazon RDS's collaborative efforts for PostgreSQL. It highlights how they synergize to empower e-commerce platforms to tailor personalized recommendations to their clientele, drawing from individual preferences and purchase records. Furthermore, we will delve into the advantages offered by these innovations, shedding light on their potential to bolster sales and enhance customer contentment within the e-commerce landscape.
Introduction to Generative AI and E-commerce Retail
Generative AI, a revolutionary technology in e-commerce retail, can transform the industry. Generative AI can generate new data by leveraging existing datasets, revolutionizing how retailers engage with customers and drive sales.
Through extensive analysis of vast amounts of data, such as user behaviour, preferences, and past interactions, Generative AI algorithms unveil concealed patterns and connections. This invaluable understanding empowers retailers to provide personalized and captivating product recommendations that deeply resonate with each customer. Consequently, the shopping experience becomes uniquely tailored and effortlessly intuitive.
One of the key advantages of Generative AI is its ability to overcome traditional limitations of recommendation systems. It tackles challenges like the cold start problem and scalability issues by generating synthetic data and simulating user interactions. This means that even new or infrequently active users can receive personalized recommendations, leading to higher customer satisfaction, increased conversion rates, and stronger brand loyalty.
Integrating Generative AI with e-commerce retail represents a change in how retailers utilize data to understand and cater to customer needs. It ushers in a new era of hyper-personalization and customer-centricity, where every interaction becomes an opportunity to forge meaningful connections and drive business growth. Embracing Generative AI is not just a smart move for retailers but a necessary one to stay ahead in the competitive e-commerce landscape.
Challenges in Traditional Recommendation Systems
Problem with Traditional Recommendation Systems
Data Sparsity:
-
Traditional recommendation systems often struggle with data sparsity, mainly when dealing with new or less active users. These users may not have provided enough explicit feedback, such as ratings or purchase history, making it challenging for the system to predict their preferences accurately.
-
Limited data availability for certain users results in sparse user-item interaction matrices, where most entries are missing. This sparsity reduces the effectiveness of collaborative filtering techniques, which rely on similarities between users or items.
Cold Start Problem:
-
The cold start problem arises when traditional recommendation systems cannot provide meaningful recommendations for new users or items with insufficient historical data. Since these systems heavily rely on past user interactions to generate recommendations, they struggle when faced with a lack of such data.
-
New users are particularly affected by the cold start problem as they have no historical interactions within the system. Similarly, new items may not have accumulated enough user feedback to inform the recommendation algorithm.
Scalability:
-
As e-commerce platforms grow and accumulate more user and product data, traditional recommendation systems encounter scalability issues. Processing large volumes of data in real-time to generate personalized recommendations strains system resources and leads to performance degradation.
-
The increasing complexity of recommendation algorithms, which require significant computational resources for training and inference, exacerbates scalability challenges.
Solution Provided to Traditional Recommendation Systems
GenAI Integration with Amazon RDS for PostgreSQL
-
Integrating Generative AI (GenAI) with Amazon RDS for PostgreSQL offers a robust solution to the challenges faced by traditional recommendation systems in e-commerce retail.
-
By leveraging advanced machine learning techniques and synthetic data generation capabilities of GenAI, retailers can overcome data sparsity and the cold start problem. GenAI can generate synthetic user-item interactions, augmenting sparse data in the PostgreSQL database.
-
The integration of GenAI with Amazon RDS for PostgreSQL ensures scalability, as the relational database service can efficiently manage large volumes of synthetic data while providing reliable performance and scalability for e-commerce recommendation systems.
Utilizing GenAI for Contextual Understanding
-
GenAI enhances contextual understanding by capturing subtle cues and nuances in user behaviour within the e-commerce context.
-
By analyzing transaction histories, browsing patterns, and contextual metadata stored in Amazon RDS for PostgreSQL, GenAI can generate synthetic user interactions that reflect diverse user preferences and behaviours.
-
This contextual understanding enables the recommendation system to deliver more relevant and personalized recommendations tailored to specific user segments and browsing contexts, improving user engagement and conversion rates.
Mitigating Overfitting and Bias with GenAI
-
GenAI helps mitigate overfitting and bias by providing more adaptive and fair recommendation algorithms for e-commerce retail.
-
GenAI reduces the risk of models becoming too specialized for the training data stored in Amazon RDS for PostgreSQL by generating diverse and representative training data.
-
Additionally, GenAI can detect and mitigate biases in the data or algorithms, ensuring that recommendations are fair and unbiased across different user segments, demographics, and product categories.
Key Impact with the Solution
Enhanced Recommendation Accuracy:
Integrating Generative AI significantly enhances recommendation accuracy by addressing data sparsity, the cold start problem, and scalability challenges. The system can provide more accurate and relevant recommendations tailored to individual user preferences and contextual factors.
Improved User Experience:
Generative AI enhances the user experience by delivering more relevant and personalized recommendations, increasing user satisfaction and engagement. Users are more likely to discover products of interest, leading to higher conversion rates and repeat purchases.
Brand Reputation Enhancement:
Generative AI contributes to a more inclusive and trustworthy recommendation system by mitigating biases and unfair recommendations. This enhances the brand's reputation and fosters customer loyalty, as users perceive the platform as fair and respectful of their preferences—generative AI for the Energy Sector.
Role of Generative AI in Recommendation Enhancement
1. Personalization
-
Generative AI facilitates crafting personalized suggestions by extensively analyzing user data and generating synthetic data to mimic user interactions.
-
By understanding individual preferences and behaviours, Generative AI algorithms can tailor recommendations to each user's unique tastes, enhancing their relevance and effectiveness.
2. Overcoming Data Sparsity
-
Traditional recommendation systems often struggle with data sparsity, especially for new or infrequently active users.
-
Generative AI addresses this challenge by generating synthetic data to augment the existing dataset, ensuring that recommendations remain relevant and personalized even for users with limited historical data.
3. Addressing the Cold Start Problem
-
The cold start problem arises when traditional recommendation systems struggle to provide recommendations for new users or items with minimal historical data.
-
Generative AI mitigates this issue by leveraging synthetic data generation to simulate user interactions and preferences, enabling the system to make meaningful recommendations even for new or less active users.
4. Improving Recommendation Quality
-
By leveraging generative AI algorithms, hidden patterns and relationships in data can be revealed, resulting in more precise and pertinent recommendations.
-
By analyzing user behaviour and preferences, Generative AI can identify unique combinations of items that traditional algorithms may overlook, resulting in improved recommendation quality and increased user satisfaction.
5. Enhancing User Engagement
-
Personalized recommendations generated by Generative AI algorithms enhance user engagement by presenting users with products or content that align with their interests and preferences.
-
Generative AI increases user engagement, encourages exploration, and drives conversions and sales by providing relevant and engaging recommendations.
6. Adapting to Changing Preferences
-
Generative AI continuously learns from user feedback and adapts to changing preferences and trends over time.
-
By analyzing user interactions and adjusting recommendations accordingly, Generative AI ensures that recommendations remain relevant and up-to-date, maximizing user satisfaction and retention.
7. Scalability
-
Generative AI offers scalability by generating synthetic data to augment existing datasets, enabling recommendation systems to efficiently handle growing volumes of user data.
-
This scalability ensures that recommendation systems can continue to deliver personalized and relevant recommendations even as the user base and data size increase.
In summary, Generative AI is crucial in enhancing recommendation systems by enabling personalization, overcoming data sparsity and the cold start problem, improving recommendation quality, enhancing user engagement, adapting to changing preferences, and ensuring scalability. By leveraging Generative AI, recommendation systems can deliver more accurate, relevant, and personalized recommendations, driving business growth and enhancing the user experience of Generative AI for Retail.
Overview of Amazon RDS for PostgreSQL
Amazon RDS (Relational Database Service) for PostgreSQL is a fully managed relational database service offered by Amazon Web Services (AWS). It provides a scalable, high-performance, and reliable database solution for e-commerce retailers to store and manage their product catalogues, user profiles, and transaction data. With features such as automatic backups, failover support, and seamless scalability, Amazon RDS for PostgreSQL simplifies database management and allows retailers to focus on delivering exceptional customer experiences.
Integrating Generative AI with Amazon RDS
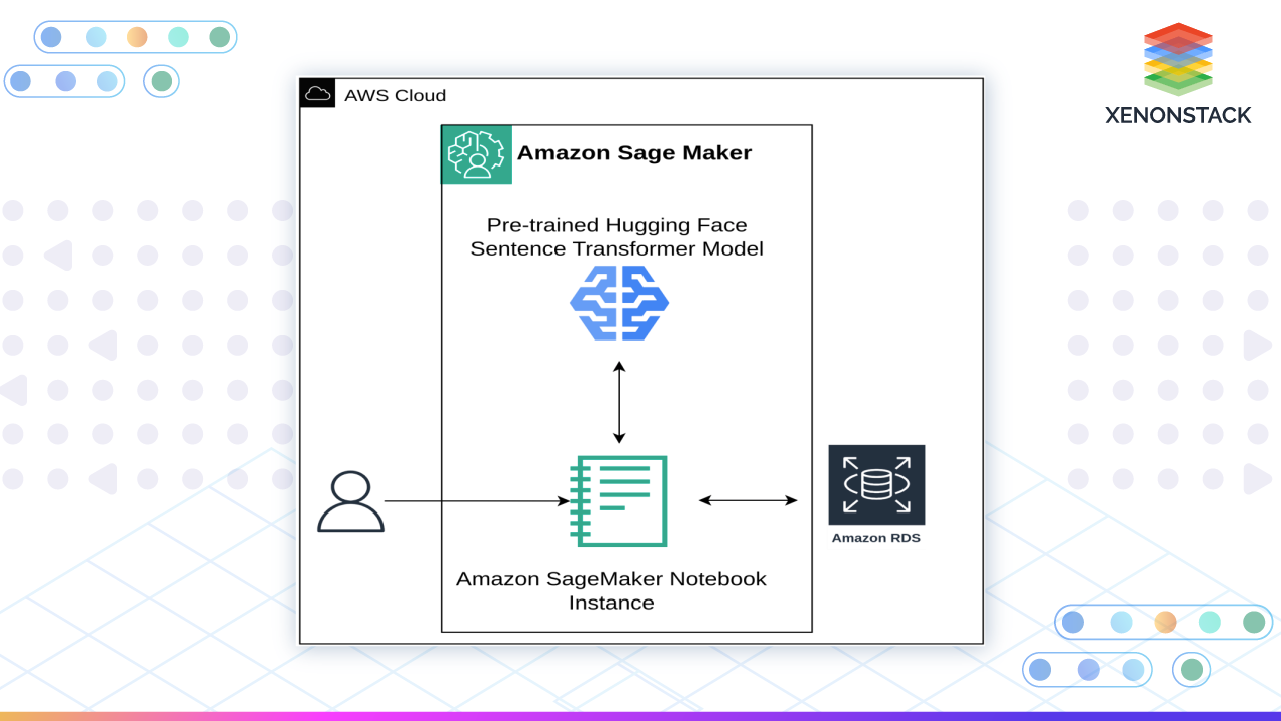
Integrating Generative AI with Amazon RDS involves harnessing the capabilities of Generative AI models, such as Generative Adversarial Networks (GANs) or Variational Autoencoders (VAEs), to produce synthetic data and store it in Amazon Relational Database Service (Amazon RDS). This integration offers numerous advantages, including scalability, security, and simplified management.
Generative AI models can generate new data samples that replicate the patterns found in the training data. This makes them valuable for data augmentation, privacy preservation, and testing tasks. By integrating Generative AI with Amazon RDS, organizations can generate synthetic data and securely store it in a managed relational database in the cloud. Amazon RDS streamlines database management tasks by providing features like automatic backups, scaling, and security enhancements. This integration ensures the generated data is stored securely and efficiently accessible for various applications. It also allows organizations to scale their data generation efforts as needed without the burden of infrastructure management.
Real-world Use Cases and Success Stories
Numerous e-commerce retailers have leveraged Generative AI and Amazon RDS for PostgreSQL to enhance their recommendation systems and drive business growth.
-
Healthcare Innovation: Healthcare organizations use Generative AI and Amazon RDS to generate synthetic medical images to train diagnostic algorithms. This approach helps augment limited datasets, improve the accuracy of medical imaging analysis, and accelerate the development of innovative healthcare solutions.
-
Financial Services: Banks and financial institutions utilize Generative AI and Amazon RDS to generate synthetic financial data for risk assessment, fraud detection, and algorithmic trading. These organizations can enhance their models' robustness and mitigate potential risks by creating realistic but synthetic financial transactions.
-
Content Generation: Media and entertainment companies leverage Generative AI and Amazon RDS to create synthetic content, such as artwork, music, and video clips. This enables them to produce a vast amount of high-quality content quickly and cost-effectively, catering to diverse audience preferences and driving engagement.
-
Manufacturing and Design: Manufacturers utilize Generative AI and Amazon RDS to generate synthetic product designs and simulations, optimizing production processes and reducing time to market. Manufacturers can identify and implement improvements by simulating various design iterations more efficiently, enhancing product quality and innovation.
Addressing Challenges in Deployment
Leveraging the advanced features of Generative AI and Amazon RDS can enhance recommendation systems, but implementing these technologies may pose particular difficulties. E-commerce businesses must prioritize data protection, algorithm efficiency, and smooth integration of recommendation systems into their current setup. Collaborating with seasoned tech partners and adhering to industry standards can help retailers tackle these obstacles and fully leverage the capabilities of Generative AI and Amazon RDS for PostgreSQL.
Future Trends in E-commerce Recommendations
Looking ahead, the future of e-commerce recommendations lies in continuous innovation and adaptation to evolving consumer preferences and technological advancements. As Generative AI algorithms become more sophisticated and scalable, retailers can expect further improvements in recommendation accuracy and personalization. Additionally, advancements in cloud computing and big data analytics will enable retailers to analyze more extensive datasets and derive more profound insights into customer behaviour, further enhancing the effectiveness of recommendation systems.
Best Practices for Implementation
To successfully implement Generative AI and Amazon RDS for PostgreSQL in e-commerce recommendation systems, retailers should follow best practices such as:
-
Ensuring data privacy and security compliance
-
Optimizing algorithm performance and scalability
-
Conducting thorough testing and validation
-
Monitoring and analyzing system performance metrics
-
Continuously iterating and improving recommendation algorithms based on user feedback and data insights.
Conclusion
In conclusion, combining Generative AI and Amazon RDS for PostgreSQL offers e-commerce retailers a powerful solution for transforming their recommendation systems and delivering personalized, engaging customer experiences. By overcoming the challenges of traditional recommendation systems and harnessing the capabilities of advanced machine learning and database technologies, retailers can drive sales, increase customer loyalty, and stay ahead of the competition in today's highly competitive e-commerce landscape.
To maximize the benefits of these technologies, retailers should partner with experienced technology providers, invest in ongoing training and development, and remain agile and adaptable to changing market dynamics and consumer trends. By adopting an effective strategy and implementation approach, e-commerce businesses can discover fresh avenues for growth and innovation in the modern digital landscape.
Next Steps in Retail Recommendation with Gen AI
Talk to our experts about implementing advanced AI systems and how industries and departments utilize Decision Intelligence to deliver personalized retail recommendations. Leverage GenAI and Amazon RDS for PostgreSQL to optimize data-driven insights, enhance customer experiences, and drive operational efficiency.