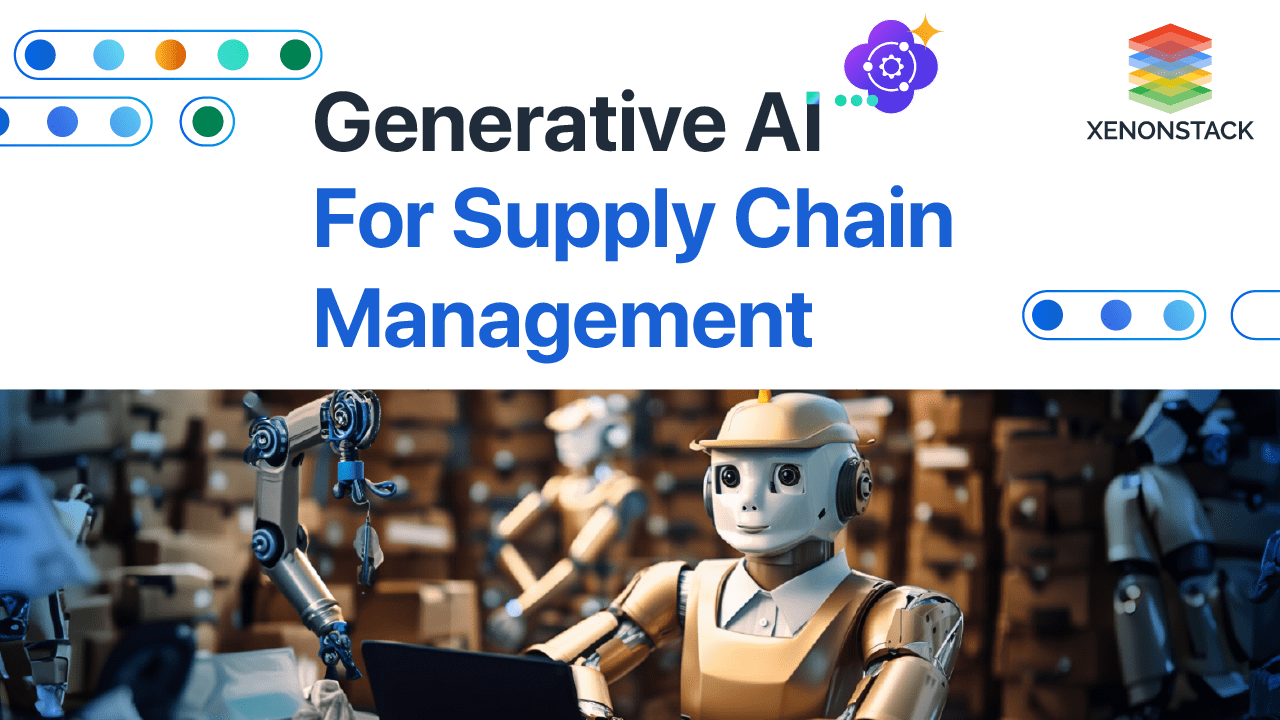
Sustainable Supply Chain Strategies
One way to improve the sustainability and profitability of supply chains is to adopt the following strategies:
-
Green Procurement Policies: Choose suppliers that meet environmental and social standards, such as using renewable energy, recycling materials, and respecting human rights. This can enhance the reputation and performance of the supply chain.
-
Lean Principles: Eliminate non-value-added activities and resources that increase costs and environmental impacts. Focus on quality and efficiency to reduce overproduction, defects, and excess inventory.
-
Circular Economy Concepts: Design products and processes for reuse, repair, and remanufacturing. Extend product life cycles, reduce waste, and save resources by avoiding disposal. This has the potential to unlock fresh avenues for growth and establish a strong competitive edge.
-
Leveraging Digital Technologies: Employ digital technologies to inform decision-making through data, enable real-time visibility, and promote collaboration within the supply chain. Improve efficiency, quality, and responsiveness through technologies that enhance supply chain operations.
- Stakeholder Engagement: Engage with customers, employees, regulators, and communities. Seek feedback, insights, and support for sustainability and cost-reduction initiatives. This can increase customer loyalty, team member satisfaction, regulatory compliance, and social impact. Generative AI for Energy Sector.
Generative AI Tools for Supply Chain Visibility
By leveraging generative AI capabilities, organizations can enhance visibility, optimize operations, and foster stronger collaboration across their supply chain networks. This leads to improved efficiency, responsiveness, and better customer service.Here are some ways generative AI tools can enhance supply chain visibility and collaboration:Demand Forecasting:
Generative AI models can analyze historical sales data, market trends, and external factors to generate more accurate demand forecasts.
This improved demand visibility helps supply chain teams better plan production, inventory, and logistics.
Predictive Maintenance:
AI-powered predictive maintenance models can analyze sensor data from supply chain equipment to anticipate failures before they occur.
This enables proactive maintenance scheduling and reduces unplanned downtime.
Supply and Logistics Optimization:
Generative AI can simulate different supply chain scenarios to optimize routing, scheduling, and resource allocation.
This leads to more efficient transportation, warehousing, and delivery processes.
Anomaly Detection:
AI models can identify unusual supply chain data patterns indicating supplier delays, quality problems, or compliance violations.
Prompt detection of these anomalies allows for faster intervention and mitigation.
Automated Documentation:
Generative AI can automate the creation of supply chain documentation like purchase orders, invoices, and shipping manifests.
This streamlines administrative tasks and reduces errors.
Collaborative Planning:
AI-powered chatbots and virtual assistants can facilitate real-time collaboration between supply chain partners.
They can help coordinate tasks, share updates, and resolve issues across organizational boundaries.
Natural Language Interfaces:
Generative AI models can provide intuitive, conversational interfaces for supply chain professionals to query data, generate reports, and get recommendations. This improves the accessibility and usability of supply chain management systems.
Benefits of adopting Generative AI
The adoption of sustainable and cost-effective practices in supply chain management, facilitated by technologies like Generative AI, can yield various benefits, including:
-
Improved Demand Forecasting and Inventory Optimization: Generative AI can analyze large datasets to improve demand forecasting accuracy. Optimizing inventory levels ensures products are readily available, minimizing waste.
-
Enhanced Customer Service and Satisfaction: Generative AI-enabled supply chains ensure timely deliveries, improving product availability and customer satisfaction.
-
Reduced Operational Costs and Waste: Eliminate non-value-added activities and reduce waste through lean and sustainable practices powered by generative AI insights to contribute to cost reduction.
-
Increased Productivity and Efficiency: Enhancing supply chain efficiency with automation and data-driven decisions. This results in enhanced productivity, timely processes, and reduced delays.
-
Better Risk Management and Compliance: Generative AI can analyze risks and provide insights to manage potential disruptions. Ensuring compliance with environmental and social standards mitigates risks and enhances the brand's reputation.
Conclusion
Generative AI can revolutionize supply chains by simplifying inventory management, production timelines, and shipping routes. Analyzing transactional data detects patterns, irregularities, and potential instances of fraud within the supply chain. This technology ensures comprehensive oversight across all supply chain facets, including forecasting demands, managing sales orders, tracking inventory, and assessing supplier effectiveness. Furthermore, it enhances logistical processes, optimizes sourcing, and strengthens inventory control.