Introduction of Generative Artificial Intelligence
The advancement of artificial intelligence (AI) has resulted in the emergence of a remarkable field known as generative AI. With the ability to replicate human creativity and inventiveness, this innovative area sits at the intersection of art, science, and technology.
What is Generative AI?
Generative AI is a type of AI technology that allows machines to generate new content, data, or outputs that are like human-created content. It uses large datasets to learn the underlying structure and characteristics of the data, enabling it to produce original and contextually relevant outputs. This differs from traditional AI, which focuses on recognizing patterns and making predictions based on existing data. Generative AI can generate various data types, including text, images, sounds, animations, and 3D models, and it can create entirely new data based on the patterns it has learned.
Evolution of Generative AI
Here's a brief history of generative AI
-
1950s - Markov chains were created as an early form of generative AI for generating data sequences.
-
1960s - Joseph Weizenbaum developed ELIZA, the first chatbot.
-
1980s - Neural networks were developed, inspired by the human brain, making it possible to model complex relationships between data.
Explore more about Artificial Neural Network Applications and Algorithm
-
1990s - Generative AI regained interest by developing new algorithms such as Boltzmann machines and restricted Boltzmann machines.
-
2000s - New algorithms like deep belief networks and generative adversarial networks (GANs) were developed. GANs are a type of neural network where two neural networks compete against each other to produce highly realistic and sophisticated outputs.
Explore more about generative adversarial networks
-
2010s - Generative AI gained popularity due to the availability of large datasets and the increasing power of computing hardware. It's now used for various applications, including creating art, generating music, and writing text.
-
2020s - Generative AI constantly evolves, developing new applications daily. It has the potential to revolutionize many industries, and the future of generative AI looks promising.
How Does Generative AI Work?
Generative AI models utilize neural networks to identify patterns and structures within existing data, enabling them to generate unique and original content. One of the significant breakthroughs of generative AI models is the ability to use unsupervised or semi-supervised learning techniques to train them. This has allowed organizations to easily and quickly leverage large amounts of unlabeled data to create foundation models. As the name implies, foundation models serve as a basis for AI systems that can perform multiple tasks.
Types of Generative AI Models and LLM Model Training
Examples of foundation models include GPT-3 and Stable Diffusion, which allow users to harness the power of language. For instance, popular applications like ChatGPT, which uses GPT-3, enable users to generate a paragraph or content based on a text request. GPT-4 generates text by accepting both text and image inputs. On the other hand, stable diffusion allows users to generate photorealistic images by providing text input.
Key Modalities of Generative Artificial Intelligence
Generative AI has expanded its capabilities across six key modalities, demonstrating its capacity to create diverse outputs that were once exclusively associated with human creativity and thought:
1. Text
Outputs written language in an accessible tone and quality tailored to user needs.
Examples: Summarizing documents, crafting customer-facing materials, and explaining complex topics in natural language.
2. Code
Generates computer code in various programming languages, autonomously summarizing, documenting, and annotating for human developers.
Examples: Creating code from natural language descriptions and maintaining code across different platforms.
3. Audio
Produces audio outputs in natural, conversational, and colloquial styles, with the ability to shift rapidly among languages, tones, and complexities.
Examples: Powering call centres with generative AI for customer interactions and providing troubleshooting support for technicians in the field.
4. Image
Responds to textual or visual prompts by creating images with varying degrees of realism, variability, and creativity.
Examples: Simulating product appearances in a customer's home and reconstructing accident scenes for insurance claim assessments.
5. Video
Like imagery, it generates videos based on user prompts, featuring entirely fictitious scenes, people, and objects created by the model.
Examples: Autonomously producing marketing videos to showcase new products and simulating scenarios for safety training.
6. 3D/Specialized
Extrapolates 3D objects from text or two-dimensional inputs, enabling virtual renderings and AI-assisted prototyping in a purely virtual space.
Examples: Creating virtual environments in an omniverse, aiding in AI-assisted prototyping and design.
Value addition by Generative AI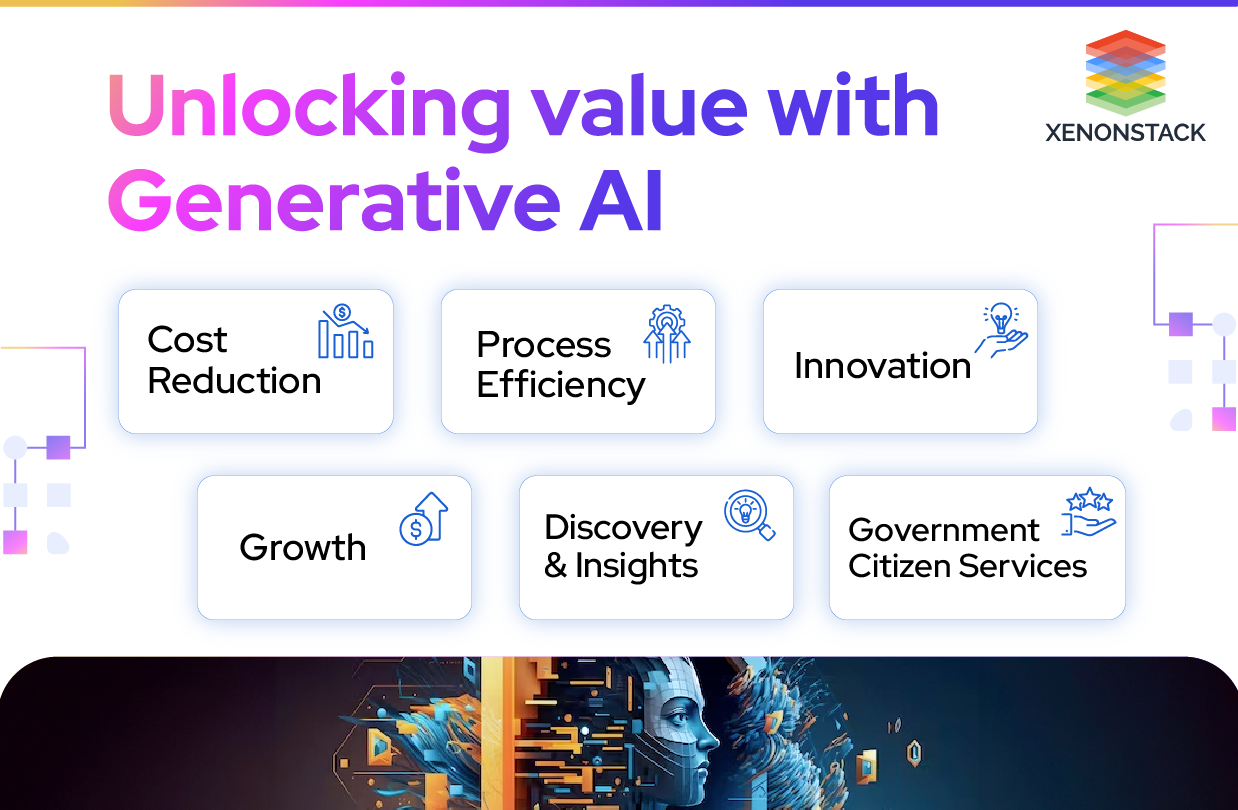
Generative AI holds the potential for value capture across broad categories, contributing to competitive differentiators and operational excellence through six dimensions.
1. Cost Reduction
Achieve cost savings by automating standard tasks and minimizing manual interventions in various processes.
2. Process Efficiency
Enhance overall operational efficiency by automating routine tasks, streamlining workflows, and reducing the need for manual involvement.
3. Growth
Drive revenue growth by leveraging generative AI for hyper-personalized marketing strategies, targeting specific customer segments more effectively.
4. Innovation
Accelerate innovation by expediting the development of new products or services, facilitating quicker iterations, and speeding up the time-to-market.
5. Discovery and Insights
Uncover new ideas, insights, and questions through the creative capabilities of Generative AI, leading to a deeper understanding of various domains and industries.
6. Government Citizen Services
Enhance government services by utilizing Generative AI to improve citizen interactions, streamline processes, and offer innovative solutions for public administration.
Best practices for implementing Gen Artificial Intelligence (AI)
Implementing Generative AI involves several crucial considerations and best practices to ensure effective, ethical, and secure utilization. Here are key practices to keep in mind:
1. Understanding the Technology
Before implementing Generative AI, ensure a deep understanding of the technology in use, such as GANs, VAEs, or other models. Knowing their strengths, limitations, and potential biases is crucial for informed implementation.
2. Data Quality and Diversity
The foundation for training generative models lies in high-quality, diverse datasets. Ensure training data mirrors real-world scenarios the model will encounter. Avoid biased or skewed data, which can result in biased outputs.
3. Ethical Considerations
Be mindful of ethical implications, mainly if AI generates content. Establish guidelines and safeguards to prevent the generation of inappropriate, offensive, or harmful content.
4. User Feedback and Iteration
Implement mechanisms for collecting user feedback, particularly in applications where AI generates creative content. User feedback is valuable for refining the model, reducing biases, and enhancing the overall user experience.
5. Transparency
Maintain transparency about using generative AI, primarily if it interacts with users or generates content that might be mistaken for human-created. Communicate when users interact with AI and manage expectations about its capabilities and limitations.
6. Security Measures
Implement robust security measures, mainly if the AI system deals with sensitive data. This includes securing the training data, model, and any generated content. Regularly update and patch the AI system to protect against potential vulnerabilities.
7. Interpretability and Explainability
Work towards making generative AI models interpretable and explainable. This is particularly important in critical applications where understanding why a particular decision was made is crucial for user trust.
8. Compliance with Regulations
Ensure compliance with relevant data protection, privacy regulations, and industry-specific standards. Adhering to these regulations is crucial for legal and ethical use.
9. Continuous Monitoring
Implement systems for continuous monitoring of the AI system's performance. This includes vigilance for biases, unexpected behaviors, and any drift in data distribution that could impact the model's effectiveness over time.
10. Scalability and Performance
Design the system to be scalable and performant. As the user base grows, ensure the AI system can handle increased demand without sacrificing performance, maintaining a reliable user experience.
Positive Impact: Empowering Innovation and Transformation
Generative AI algorithms have the potential to revolutionize various fields and bring about significant positive changes. Some examples of the impact of generative AI are listed below.
1. Medical Imaging Enhancement
Generative AI aids radiologists in detecting abnormalities and structures, improving accuracy in medical image analysis.
2. Personalized Medical Treatments
Generative AI personalizes medical treatments, optimizing therapeutic outcomes for individuals based on their unique characteristics.
3. Language Translation without Barriers
Generative AI-powered language translation eliminates language barriers, facilitating effective communication across different languages.
4. Tailored Learning Experiences
Generative AI tailors learning experiences to individual preferences, optimizing engagement and comprehension in educational settings.
5. Enhanced Content Creation and Marketing
Generative AI improves content creation and marketing campaigns, amplifying engagement and customer satisfaction.
6. Revolutionizing Drug Discovery
Generative AI predicts molecular structures and identifies potential drug candidates, revolutionizing medical treatment.
7. Efficient Chatbot Customer Service
Chatbots powered by generative AI offer efficient and personalized customer service, enhancing the user experience.
8. Optimized Supply Chain Operations
Generative AI optimizes supply chain operations, improving efficiency and reducing operational costs.
9. Urban Planning and Infrastructure Optimization
Generative AI aids urban planners in designing and optimizing city layouts, transportation networks, and infrastructure development.
10. Natural Language Interfaces for Coding
Generative AI-powered natural language interfaces enable developers to interact with code using plain language, speeding up the coding process and automating documentation.
Negative impact: Confronting Ethical Challenges
Generative AI, while holding immense potential for positive advancements, also raises several ethical and societal concerns. As with any powerful technology, it can be misused or have unintended consequences. Here are some negative use cases and potential impacts of Generative AI:
1. Misinformation and Fake Content
Generative AI can be exploited to create convincing fake news, images, and videos, leading to the spread of misinformation and erosion of trust in media.
2. Privacy Violations and Deepfakes
Hyper-realistic deepfakes generated by Generative AI pose risks of privacy violations, identity theft, and reputation damage, impacting individuals and society.
3. Automated Cyberattacks
Cybercriminals can leverage Generative AI to automate and evolve sophisticated cyberattacks, making it challenging for traditional security measures to detect and defend against them.
4. Job Displacement
Integrating Generative AI in creative tasks raises concerns about job displacement in content creation, design, and coding, potentially devaluing human creativity.
5. Bias and Discrimination
Generative AI trained on biased data may perpetuate societal biases, leading to AI-generated content reinforcing stereotypes and unequal representation.
6. Intellectual Property Challenges
Determining ownership and copyright of AI-generated works becomes complex, leading to legal disputes over intellectual property rights.
7. Erosion of Authenticity
The proliferation of AI-generated content raises questions about the authenticity of creative works, impacting the cultural and artistic landscape.
8. Overdependence and Creativity Stagnation
Overreliance on generative AI may lead to a stagnation of human creativity and innovation, as humans might miss out on exploring new and unconventional ideas.
9. Ethical Dilemmas in Entertainment
AI-generated content blurs the line between factual and fabricated experiences, raising ethical concerns in areas like virtual influencers or digitally resurrecting deceased individuals.
10. Loss of Skill Development
Dependence on AI-generated solutions might result in declining human skills and expertise in creative domains, as individuals may need more motivation to develop their abilities.
Conclusion of Generative Artificial Intelligence
Generative AI showcases its remarkable capabilities, pushing the boundaries of creativity and innovation across healthcare, art, education, and more. Its positive use cases promise transformative advancements that can enhance human lives. However, this progress necessitates responsible development and ethical considerations. Addressing challenges related to misinformation, job displacement, privacy, bias, and ownership is crucial. Proactive measures can help harness the potential of generative AI while ensuring alignment with ethical principles and societal well-being. As stewards of this technology, we can shape a future where the positive impacts of Generative AI are maximized and negative implications are mitigated through thoughtful and ethical practices.