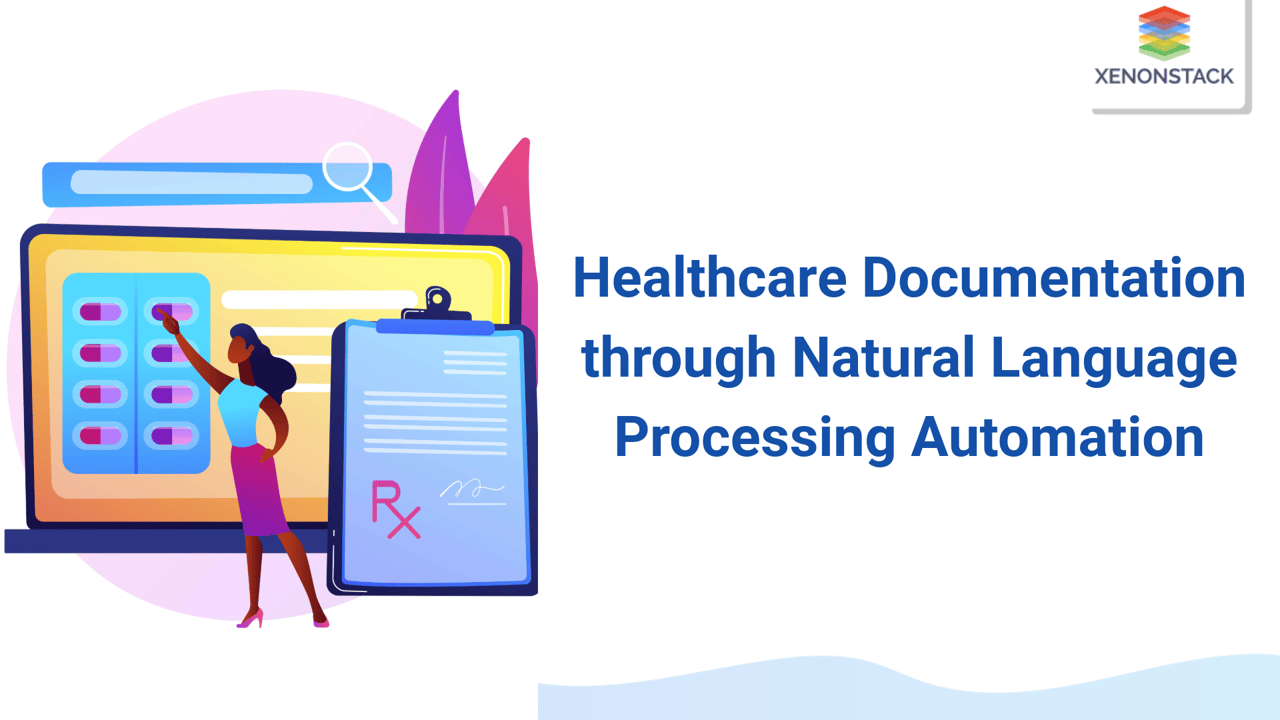
It is common knowledge that the healthcare industry's documentation burden has always been a massive problem. EHRs, clinical notes, discharge summaries, and insurance documentation centre around much of the time doctors, nurses, and administrative staff devote to paperwork. This process becomes time-consuming and counterproductive, promoting burnout and limiting clinicians' time with their patients.
Natural Language Processing (NLP) is an AI field that helps computers read, comprehend, and analyze human language. NLP is revolutionizing healthcare documentation by automating manual workflows, improving data accuracy, and allowing medical professionals to focus on what they do best: caring for patients.
The Challenges of Healthcare Documentation
For several years, documentation within healthcare settings has been cited to cause major problems for caregivers and healthcare systems regarding the time and quality of patient services. Let’s explore the key pain points that make this process so burdensome:
-
Time-Consuming Process
Clinicians commonly use electronic health records, and common reports reveal that a clinician can spend as much as 30-40% of his workday on data entry in EHRs. Such time demands in the managerial area result in decreased time spent on patient contacts and, consequently, decreased quality of patient care. -
Human Errors
Data keyed in manually tends to be either partial or contain full errors; therefore, it is unreliable. This can be very dangerous in areas such as patient diagnosis, treatment, and even prognosis, not to mention the effects on patient results.
-
Data Overload
Another exponentially increasing challenge is clinical data. Doctors are inundated with large amounts of data at once and often lack the proper tools to distil those findings.
-
Physician Burnout
Encompassing administrative tasks increases physicians’ burnout, compromises healthcare quality, reduces team member satisfaction, and increases staff turnover rates.
-
Compliance Issues
Healthcare organizations must meet stringent legal requirements, like the HIPAA Act, to protect patients’ information. Addressing these requirements manually is cumbersome and increases the possibility of non-compliance.
To overcome these challenges, the field known today as Natural Language Processing (NLP) has been developed as a solution. This is because NLP reduces complexity in manual workflows, where clinicians initially had to spend a lot of time on documentation by automatically doing it. Therefore, clinicians will be more efficient and do more to help patients.
How NLP Automates Healthcare Documentation
Natural Language Processing employs artificial intelligence techniques to analyze, understand, and generate information from working notes, laboratory reports, voice and much more. In healthcare documentation, NLP focuses on the following tasks:
-
Speech Recognition
NLP-based speech-to-written text technology solutions involve speech-to-text conversion of doctors' and patients’ discussions, replacing typing. Clinicians can speak to each other and make notes, while NLP tools can write perfectly accurate notes.
-
Text Extraction and Structuring
While NLP integrates much patient documentation from EHRs, it also identifies and extracts relevant information (e.g., what the patient is presenting with, diagnoses, and medications) from unstructured text.
-
Clinical codes and bill automation
NLP is used to code medical procedures, diagnoses and treatments from bills and insurance claims using the ICD-10, CPT or SNOMED codes.
-
Template of Summary Notes of Medical Records
NLP tools extract large volumes of medical records and compile abridged reports with relevant information such as allergy history, previous diagnoses, medication use, and laboratory tests.
-
While NER stands for Named Entity Recognition, it is a process
NLP highlights medical terms such as drugs, symptoms, localization, and laboratory values as part of text-based records.
-
Real-Time Documentation
Sophisticated NLP tools allow for the documentation of clinical notes during the actual encounter, thus allowing timely and correct documentation.
NLP erases the time-consuming work procedures and enhances the quality of documents typical for healthcare.
New Developments in NLP for Health Care Documentation
Pretrained Models Depending on the Transformer Technique (BERT, GPT)
A broad range of new models like BERT (Bidirectional Encoder Representations from Transformers) and GPT (Generative Pre-trained Transformer) have pushed NLP to a new level, optimizing text comprehension and generation.
Voice AI Integration
Voice recognition technologies like Dragon Medical One and Nuance Communications are based on natural language processing, allowing clinicians to multiply their voices with well-documented notes.
Context-Aware NLP
New NLP systems can learn contextual information during medical discussions and correctly identify symptoms, diagnoses, and connections between medical terms.
Multilingual NLP Models
Healthcare is universal, and with the latest models reflecting language capabilities and incorporating more languages, clinicians can write and process medical documents in foreign languages.
Integration with EHR Systems
With the Office 2016 version, NLP tools are fully embedded with common EHR systems like Epic, Cerner or others, meaning less disruption and better integration google nlp services.
AI for Document Summarization
Automated summarization tools help produce concise medical summaries from extensive clinical notes, discharge reports, and analysis findings, reducing physicians' time on these tasks.
Applicable Areas of Healthcare documentation through NLP
- Real-Time Clinical Note Creation
The application of NLP in speech recognition can allow doctors to write notes from direct dictation during a consultation. These tools record the dialogue and encode the data as structures in EHRs.
Example: Nuance Dragon Medical connects the NLP to produce additional clinical notes in real time with a high level of specificity.
Figure 3: Nuance Dragon Medical One
- Automated Discharge Summaries
Making discharge summaries is one task that NLP tools extend so that the analysis of records, reports, and histories does not leave out any important information.Figure 4: Methodology steps for discharge summary notes preprocessing and modelling - Clinical Decision Support
NLP processes clinicians' documentation to determine patterns, call out required patient information, and extrapolate possible diagnoses and treatment plans from databases.
Example: NLP systems scan doctor notes and identify possible cases of drug-drug interaction or suggest further tests that the patient needs. -
Medical Coding and Billing Automation
One of NLP’s main uses is to automatically code treatment plans and procedure records using ICD-10 and CPT codes, enhance billing, and accelerate insurance claims. -
Patient-Generated Health Data
NLP analyzes the patients’ generated content, such as chatbot messages and emails to the clinic, to extract data such as symptoms and questions to assist clinicians in sorting through the cases. -
Research and Clinical Trials
Huge volumes of clinical notes are fed into NLP tools to determine patients who fit into clinical trials or to assess real-world evidence for therapeutic studies.
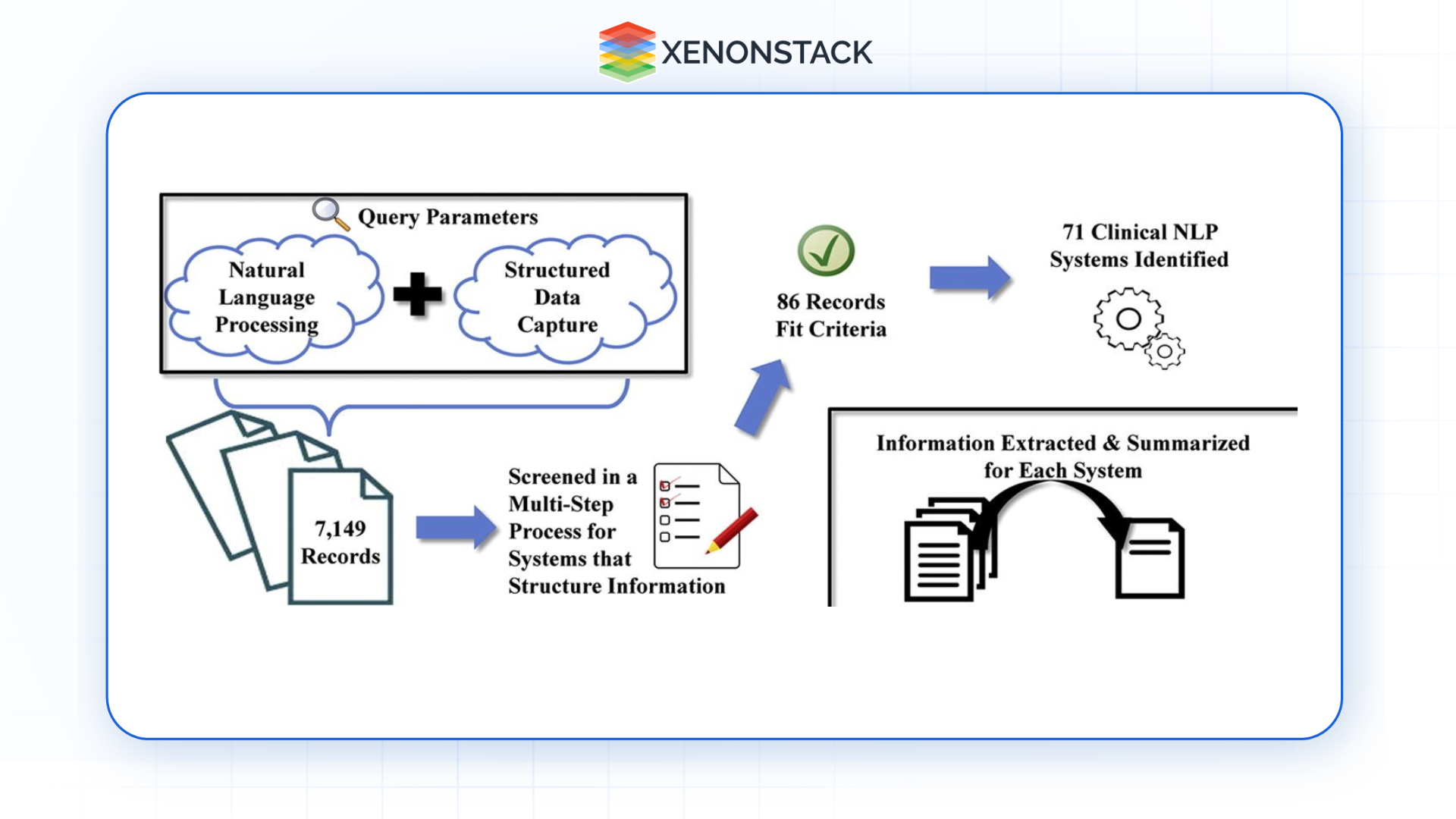
Application of NLP in Documentation of Healthcare
-
Enhanced Efficiency
First, NLP easily cuts on the time and possible monotonous work that the healthcare providers would usually take.
-
Improved Accuracy
The application of NLP tools greatly reduces the number of mistakes made when documenting episodes, entering data, coding, or billing.
-
Time Savings
Using speech-to-text and summarization to write notes in real-time freed up as much of clinicians’ time as it takes to write notes traditionally.
-
Better Clinical Insights
NLP can highlight certain elements in patients’ histograms, recognize patterns, and guide therapy.
-
Cost Reduction
Due to enhanced documentation, the use of NLP lowers the general working costs that accompany manual operations and compliance issues.
-
Improved Patient Outcomes
Adequate documentation speed accelerates diagnoses and treatments hence the quality of patient care delivered to the patients enhances.
-
Reduced Physician Burnout
It reduces the competitor's burden on clinicians and enhances their job satisfaction by avoiding administrative tasks.
Challenges in Implementing NLP for Healthcare Documentation
-
Data Privacy and Security
Dealing with medical information involves HIPAA-related questions and many other private acts.
-
Compatibility with Other Systems
NLP tool integration with current EHR systems can be challenging and require a large number of resources.
-
Accuracy of NLP Models
Due to medical data interpretation, safety, and trust, NLP must be nearly one hundred per cent % accurate.
-
Unstructured Data Variability
The heterogeneity of clinician language and documentation styles and the use of acronyms and different document templates complicate the interpretation of NLP results.
-
Adoption Resistance
Some physicians and other healthcare stakeholders using AI-based solutions have concerns about their dependability and possible alterations to a knowledge workflow system.
NLP in Healthcare: What’s Next?
The future of NLP in healthcare is promising, with continued advancements set to revolutionize the industry:
-
AI Integrated Virtual Helpers
Integrating the virtual assistant into health care through NLP will be normal since the assistants are used for real-time patient documentation, diagnosis support, and communication with the patient.
-
Improved Voice AI
The accuracy of voice recognition will improve. The voice recognition system will identify different accents and fully automate clinical speech-making notes.
-
Multimodal AI Integration
Patient documentation and monitoring will be all-encompassing, embedded in patients’ records and supported by data obtained through NLP, medical imaging, and wearable devices.
-
Personalized Medicine
NLP will work together with genetic and lifestyle data, assisting in developing individual treatment strategies.
-
Global Accessibility
Applying natural language processing tools, written documents will be translated into interpreted information that clinicians can understand regardless of location.
Integrating NLP technology into healthcare documentation is a leap to a new level and approach to minimizing margin errors while simultaneously delivering optimal patient care services. From speaking note-taking and medical coding to automated medical coding, NLP Tools usher clinicians in new ways to serve their patients with optimally integrated toolsets.
Considering the recent developments such as transformers, speech AI and real-time data analysis, the use of NLP in the contemporary healthcare sector will expand further. Healthcare organizations should, therefore, embrace the use of NLP-powered tools that deliver efficiency, low cost, and value in health outcomes. The future of creating, implementing and maintaining health records is automated and intelligent and encompasses the patient.
Next Steps with Automating Healthcare Documentation
Consult our experts about implementing advanced AI systems and how industries and departments use Decision Intelligence to become decision-centric. Leverage AI to automate and optimize healthcare documentation with Natural Language Processing, improving accuracy, efficiency, and responsiveness.