Introduction to Data Gaps and Costs
Using any tool without proper attention to fundamental data can lead to significant consequences and increase hidden costs, affecting business operations, decision-making, and efficiency. Therefore, understanding these issues, identifying the sources of data gaps, and applying the right data collection methods are crucial for any company.
Data users often feel frustrated when data is incomplete, inaccurate, or delayed. The difficulty lies in the fact that many applications were developed over the years as point solutions for specific issues. However, defining the necessary data, the time required for its collection, and managing this process is not straightforward. Poor data quality typically stems from three main factors: gathering, archiving, and processing data.
If there are gaps in your end-to-end process, tools, or personnel in data engineering that you have or lack, it means that you will have the wrong data in your pipeline. This is most commonly evident with regard to new Data Integration and Data Transfer scenarios.
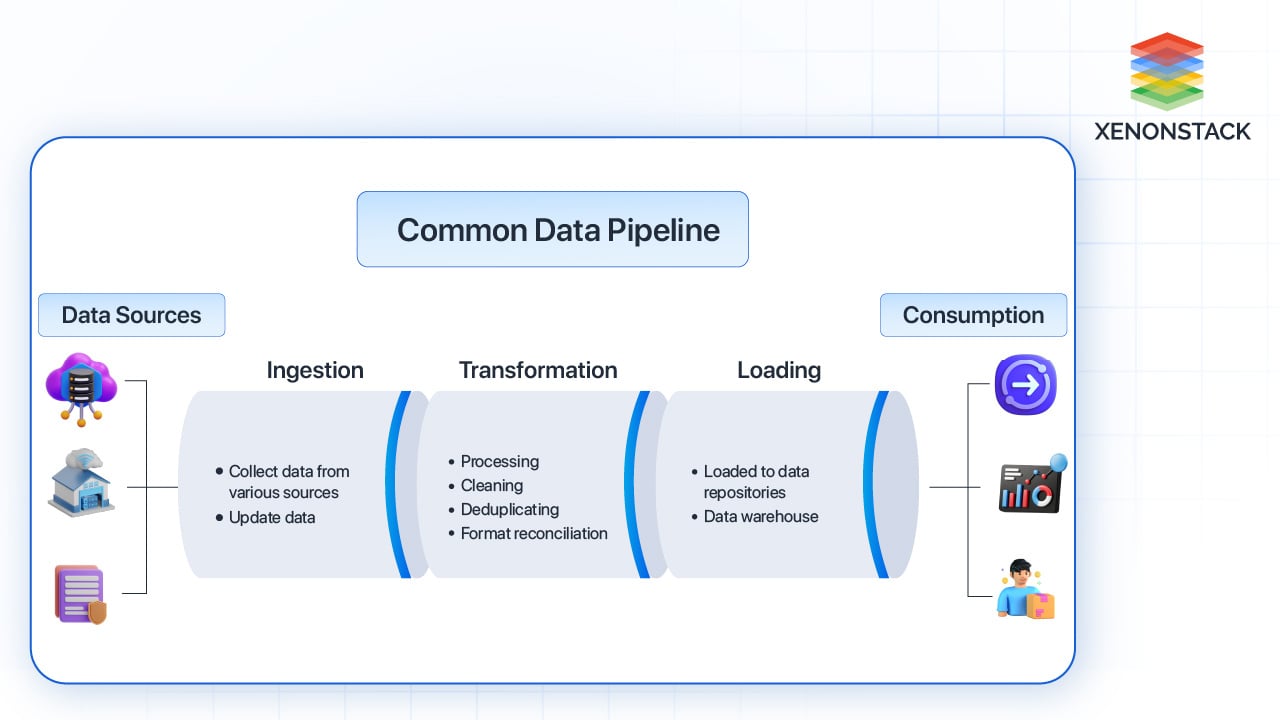
Also, the problems that you meet infallibly face the data scientists that you hire and who spend lots of time on data rectification, cleaning, fixing, and formatting. Not only can they fail to address the basic issues with the data, but they can’t even come up with the insights and forecasts they were hired to deliver in the first place.
Business Impact of Missing Data
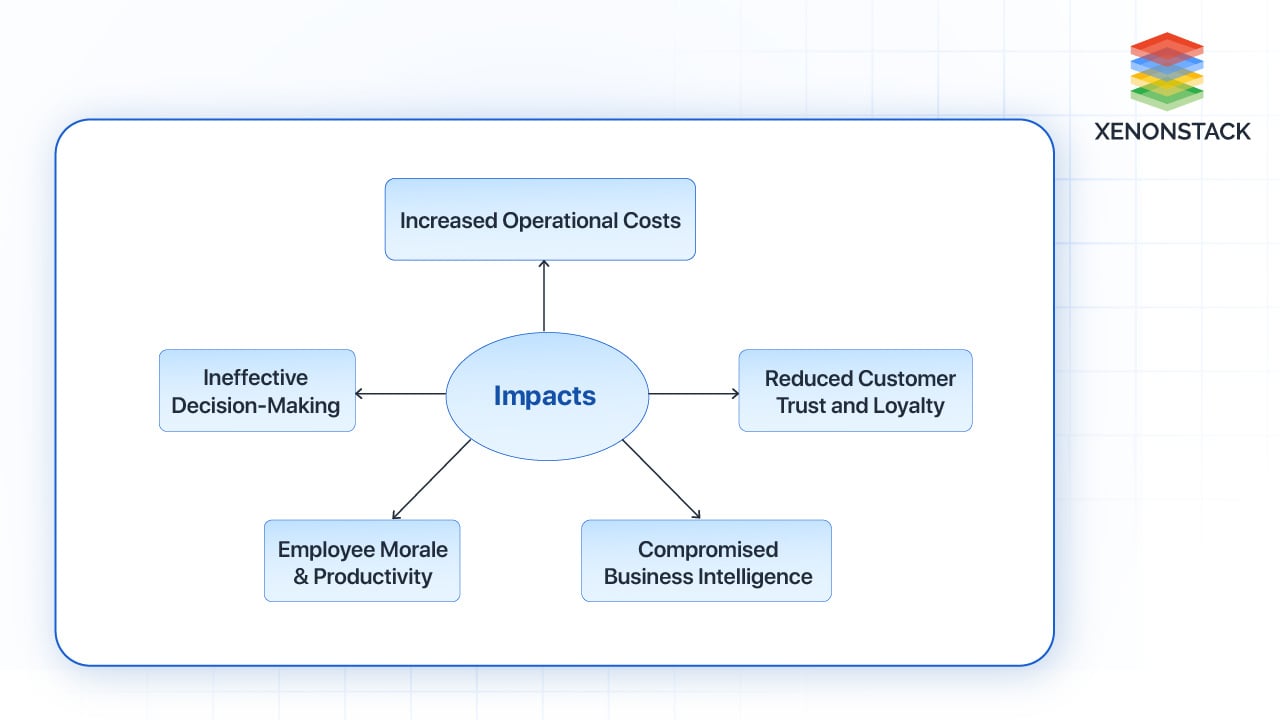
Revenue Loss
Poor records may have an instantaneous and negative effect on an enterprise’s bottom line. Inaccurate client records, improper information about the product, and improper ordering methods can lead to misplaced revenues, decreased client satisfaction, and erosion of brand value, as per Gartner. According to the source, each organization can lose $15 million per year on account of bad data disparity.
Diminished Operational Efficiency
When employees lose their precious time correcting facts errors or searching for correct facts, they significantly diminish their output and standard output. This may lead to choice delays, lost time for time-sensitive work, and improved administrative costs.
Flawed Analytics and Decision-Making
The reliability of facts evaluation and prediction models depends only on the quality of the data on which they base their information. It is critical because incomplete, duplicated, or inauthentic statistics may lead to wrong conclusions and terrible strategic decisions with far-approaching effects for the business enterprise.
Compliance Risks
Stringent facts privacy guidelines, including the General Data Protection Regulation (GDPR) and the California Consumer Privacy Act (CCPA), compel groups to maintain accurate and updated non-public facts. Failure to do so will cost them excessively and damage their reputation.
Missed Opportunities
A lack of effective facts can spare organizations from analyzing market characteristics, identifying customer needs, and utilizing new product or service opportunities; this can provide competitors with data control. Effective practices have been beneficial.
Reputational Damage
Because clients are so fact-conscious, scandals related to records breaches, flawed product statistics, or negative reviews can soon undermine trust and damage a company’s reputation, which should be rebuilt.
Explore the crucial role of Data Quality in driving business success and Learn Best Practices, Solutions, and Architectures to ensure High-quality Data Management. Click here!
Identifying and Resolving Data Gaps
Here are some strategies to address these issues:
- Data Quality Assessment: Regular checks to identify missing, duplicate, or inconsistent data. This may involve using validation scripts or default rules to check the integrity of the data.
-
Implementing Data Management Solutions: Invest in facts and fine control gear that automate the method of correctly storing and coping with statistics. This consists of standardizing statistics structures and definitions to ensure consistency throughout the company.
-
Data Validation Processes: Establish strong record validation approaches throughout facts collection to decrease incomplete records. This can encompass mandatory fields and real-time validation exams.
-
Continuous Monitoring: Track and examine styles of lacking statistics to identify underlying causes. Implement preventive measures to lessen future occurrences, including enhancing data series techniques and team member education.
Data Quality Tools and Techniques
To maintain high-quality data, organizations can leverage a variety of tools:
-
Data Transformation Tools: DBT and Dagster can help standardize and format data for consistent use.
-
Data Catalog Tools: Tools like Amundsen and DataHub enable efficient data discovery and documentation.
-
Data Observability Tools: Datafold helps track data quality, ensuring that data remains complete and accurate.
Additionally, open-source tools like DQOPS can perform data quality checks at both table and column levels. Real-time verification tools integrated into data pipelines ensure continuous quality compliance.
Data quality software development kit that can be incorporated into our data pipelines to conduct real-time data quality verification.
By utilizing these tools and techniques, we can guarantee that our data complies with our data quality standards, resulting in Data completeness.
Best Practices for Data Collection
To improve data quality and minimize the risks associated with incomplete data, organizations should adopt the following best practices:
Address the issue at the source
The quality region to cope with records first-rate problems is on the originating records supply. This manner addresses structures and techniques concerned with facts seized. The task of addressing issues at this layer is the excessive stage of intervention wished at a commercial enterprise manner layer, or if the records are supplied via a third birthday party in which you don't have any control. It is often difficult to get the buying across the business to put in force a facts pleasant application on the supply, although it is the quality location to cope with the issue.
Fix during the ETL(Extract, Transform, Load) process
If you can not repair records high-quality at their source, you could try to repair the issues via your ETL approaches. Many companies regularly take this pragmatic approach. Using described regulations and clever algorithms, it is viable to restore many problems in this way—making sure you've got a clean record set to record from.
Fix at the meta-data layer
Lastly, if you do now not have manage of the ETL techniques and want to research facts set ‘as is,’ then you can use rules and good judgment inside a metadata layer to fix (or mask) your record's great issues. In this way, you could observe some of the policies and common sense you will have carried out in an ETL manner; however, in this case, the underlying data is not updated. Instead, the regulations are implemented at run time to the question, and fixes are applied on the fly.