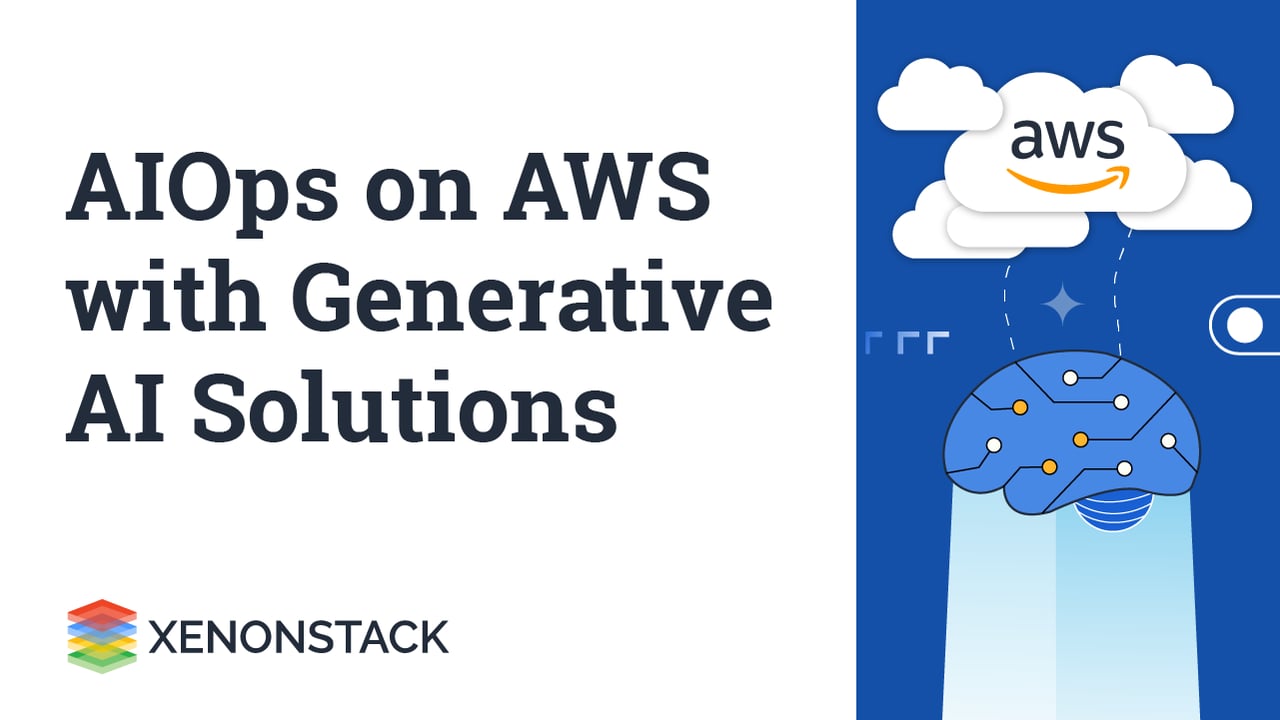
Introduction to AIOps and Anomaly Detection
AIOps (Artificial Intelligence for IT Operations) signifies a new method of IT management in words and practice. It uses data and automation to support operations and make them more efficient, minimize the time needed to solve a problem and improve service quality. AIOps has four key functions, the most important of which is anomaly detection, which involves discovering deviations from the norm in IT systems.
Such changes are useful in identifying any potential problems at the early stage, which an organization is likely to address to avoid becoming a major problem. By integrating AWS Bedrock and Titan solutions, organizations' operations can be optimized, making the AIOps plans concrete, coordinated, and utilized more efficiently and effectively.
Let me begin by asking What is your understanding of AIOps?
AIOps is a term that is used to describe the place of IT operation as well as machine learning, data analytics, as well as automation. It helps organizations work through massive amounts of operational data in real-time, which has an impact on the ability to prevent and respond to events. Thus, resource consumption becomes optimized, system performance increases, and future problems can be forecasted, thereby minimizing the need for the use of adjustment. In other words, AIOps help the IT teams shift to value-creation tasks and are not overwhelmed by operational work.
Foundations of Anomaly Detection
Anomaly detection is a helpful method that serves to search for a record that differs from other records. Understanding different types of anomalies is essential for effective detection:
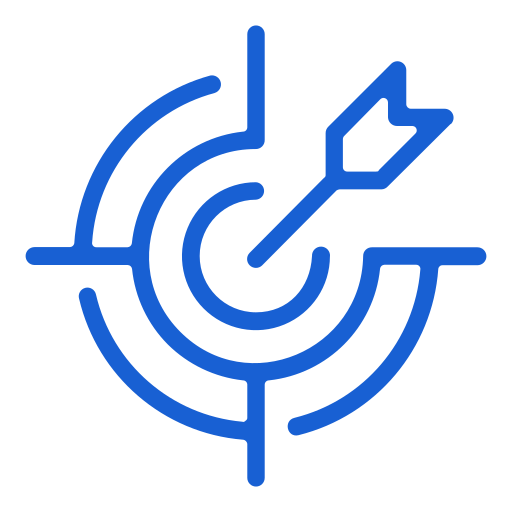
Point Anomalies
These are single values that are different from the other values of the data set. For instance, if the CPU utilization for a server starts at 30 % and goes to 100 %, then the 100 % utilization is a point anomaly
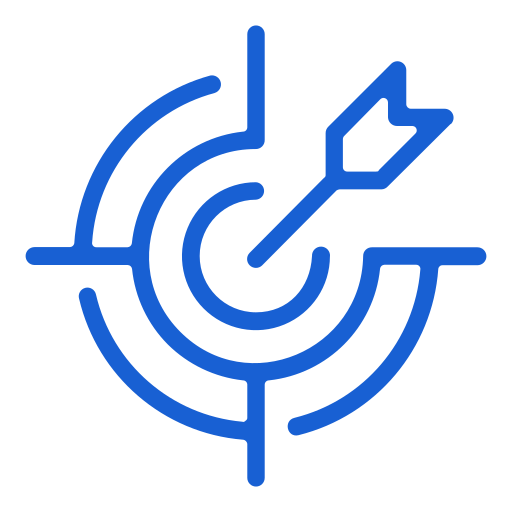
Contextual Anomalies
These are used when a data point is considered as out of range in a certain sense. For instance, congestion level on the networks can be normal during business time but might be abnormal at nighttime
Being aware of these differences enables anomaly detection systems to adapt to different situations and data kinds. While anomaly detection is a useful tool, conventional approaches frequently face serious obstacles. One of the main problems is the imbalance between normal and anomalous data. Anomalies are infrequent in many real-world circumstances, making it challenging to train models successfully.
The purpose of Generative AI is to create content, as opposed to other forms of AI, which might be used for different purposes, such as analyzing data or helping to control a self-driving car
Key Technologies in AIOps Anomaly Detection
The efficacy of AIOps in anomaly detection relies on advanced technologies:-
Machine Learning and Deep Learning: These use algorithms that can be trained and optimized from data. The models examine data in enterprises' databases to analyze normalcy, which they use to detect cases of anomalous behavior effectively.
-
Unsupervised Learning Techniques: This technique is very important when working with data that is unlabeled, untidy, or unstructured. In learn mode, the opportunity is given to select systems to discover occlusions on their own without the acquisition of specific directions for doing so, which is ideal for unpredictable settings where routines can transform.
-
Time Series Analysis: Using research data accumulated over time helps identify a trend, season, and cycle of events. This is important for detecting deviations that result from temporal factors, such as a higher traffic flow during rush hours.
-
Natural Language Processing (NLP): Log data and user feedback, which are mostly unstructured, are examples of parameters for which NLP is applied. Because AIOps deals with meaning, it can better locate problems and such based on the context that it can glean from textual data.
Enhancing Anomaly Detection with AIOps
A multifaceted strategy enhances AIOps anomaly detection:
.png?width=512&height=512&name=artificial-intelligence%20(2).png)
Featured Engineering
This process involves selecting and redesigning data aspects to enhance model efficiency. Organizations should focus on features relevant to anomaly detection models
.png?width=512&height=512&name=artificial-intelligence%20(1).png)
Hyperparameter Tuning
Choosing the appropriate model and adjusting parameters based on data characteristics can improve detection rates and reduce false positives
Real-time Anomaly Detection
Importance of Real-time Detection
-
Due to the high-speed incident activities that are faced by IT organizations, it is imperative to identify incidents in real-time. They produce not just large amounts of data but data that change frequently, and their analysis in real-time provides a way for organizations to react promptly to new developments. This means that through early detection one can avoid a small problem developing into a serious problem that may lead to major outages and loss of availability of service.
AIOps Solutions
-
Stream Processing: Once the data is in, this allows an organization to process it in real time, as it can detect an anomaly practically at a glance.
-
Event Correlation: For instance, with AIOps, one can relate disparate events to find complicated patterns. This capability is important in determining whether certain trends, such as hacking scares or system collapse, exist in a bigger context.
-
In-memory Computing: Utilizing in-memory databases helps to lessen latency, gain faster access to databases, and lengthen database processing time
Network Anomalies Detection
In a network, AIOps are essential in monitoring traffic deviations that frequently point toward issues or even threats. A network activity log allows organizations to note down some alterations, such as high traffic, strange access, or unusual data flow at the initial stages. It is also commendable since it provides an added proactive way of preserving the integrity and security of the networks.
Application Performance Monitoring
By identifying and recommending the areas that are latently causing issues, AIOps improves the application performance monitoring process. Through constant evaluation of application metrics, AIOps can illustrate where performance is fading, giving IT departments the chance to proactively fix any problems. This, in turn, enhances the reliability of the applications and the ultimate satisfaction of the user.
Security and Threat Detection
AIOps enhance security by quickly detecting vulnerability and mitigating risks using the concept of anomaly detection. The essence of configuration management is that organizations can identify anomalous behaviors of users, flows through the networks, and system logs. This capability increases an organization's preparedness to counteract threats, consequently escalating organizational protection from cyber threats.
Benefits of Implementing AIOps on AWS with Bedrock and Titan
Integrating AIOps with AWS Bedrock and Titan offers substantial benefits:
-
Proactive Issue Resolution: Detects threats to performance before they materialize into an obstacle.
-
Cost Optimization: Enables organizations to optimally manage the AWS resources.
-
Efficient Resource Management: Examines the patterns for present-time resources management.
-
Improved Security: Scans the traffic in the network for intrusion.
-
Predictive Maintenance: In order to prevent frequent incidences of hardware failures this is done in advance.
Overcoming Challenges in AIOps Anomaly Detection
Some realistic problems are that real-world data contain errors and data are always imbalanced between different classes. Solutions include:
-
Data Quality: High-quality data is essential for identifying the right anomalies. Data cleaning and normalization are just some examples of methods for improving data quality.
-
Class Imbalance: This is due to class imbalance, in which normal behavior occurs frequently compared to anomalies in many datasets. To deal with it, one can use oversampling, undersampling, and certain special algorithms.
-
Seasonality: It can often be difficult to separate between cyclical fluctuations that could be normal for birds at certain times of the year and actual specific abnormalities. The general system patterns can be discerned from interacting time series data with more sophisticated techniques to detect genuine exceptions in usual patterns.
Ethical and Privacy Considerations
As data usage grows for AIOps decisions, ethical issues such as data privacy and bias in the models used become crucial for organizations. Things like GDPR and HIPAA have to be respected. Organizations should also be clear on the processes they follow and honest about how the data is being utilized.
AIOps on AWS with Bedrock and Titan
Data gathering and /or consolidation
For AIOps to be applied, data must be unified and centralized to geometric storage solutions in AWS. This includes using different monitoring tools, which can be combined to gather real-time information from the IT infrastructure.
Data Preprocessing
Data cleaning is crucial for making the right conclusions and achieving the goal. Preliminary operations like cleaning, normalization, and transformation of the sheer amount of data can be useful in enabling the data to undergo an efficient process for detecting outliers.
Pattern Recognition
The use of machine learning algorithms for pattern recognition is highly important. It covers determining the baseline from previous behaviors, or the lack of it, and identifying behavioral anomalies.
Predictive Analytics
Amazon AWS SageMaker helps organizations create models that predict and thus prevent upcoming problems utilizing past experience and trends.
Synthetic Data Generation
2 make use of Generative AI to produce synthetic data for improving testing needs.
Collaborative Monitoring and evaluation for sustainability
AIOps is a continuous process, but it must be noted that more frequent performance checks and updates are generally provided. This guarantees that the AIOps strategy is constantly relevant, given that the efficacy of the system needs to be evaluated more often while tweaking algorithms on the platform.
Next Big Things in AIOps and Anomaly Detection
In the future, the development of AIOps technologies will continue to expand the symbiotic relationship between DevOps and SecOps. Also, improvements will be made to the level 2 work concerning autonomous incident management, which will improve the system's resiliency and case response time.
So, AIOps on AWS using Bedrock and Titan is indeed a great solution for current IT operations. With these innovations, organizations are able to enhance IT efficiency and reliability as well as proactively manage in the midst of the techno-logicalities of current-day IT organizations.
Discover more about AIOps for Telecom Industry How are Integrate AIOps in DevOps