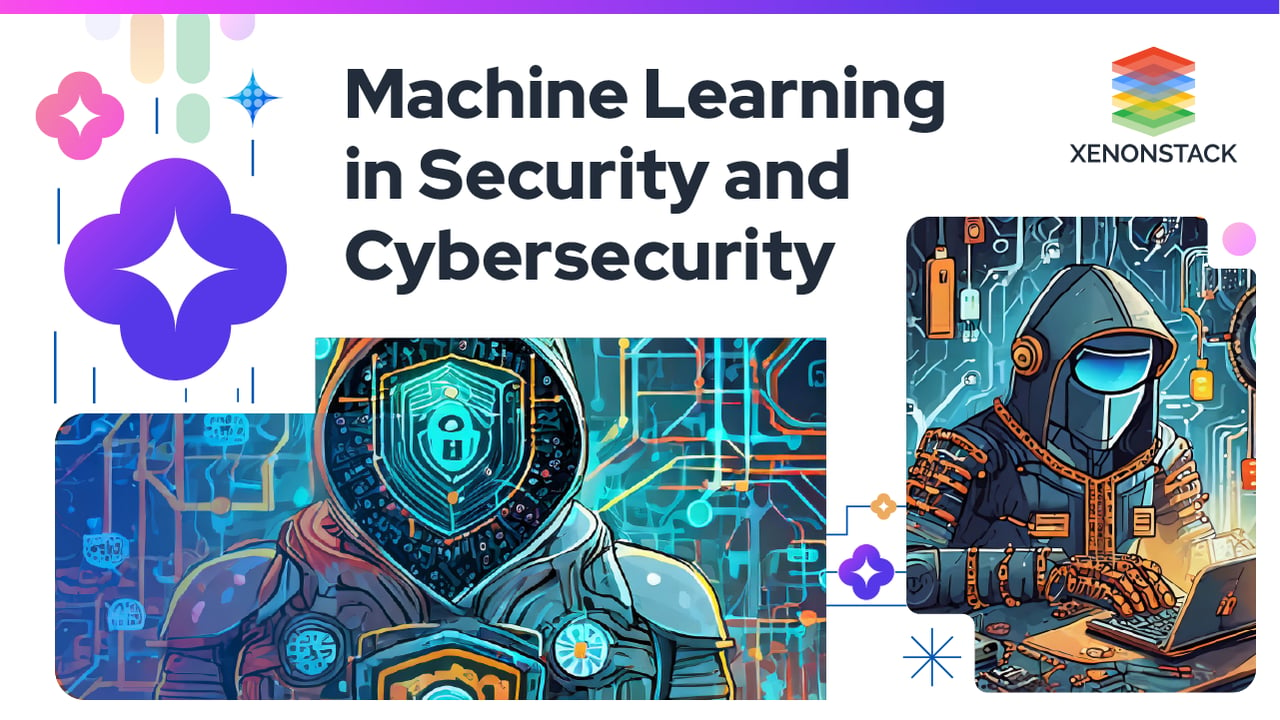
The Top ML-based security tools are
1. bioHAIFCS: A bio-inspired AI framework for securing critical network applications.
2. Cyber Security Tool Kit (CyberSecTK): Python library for cyber-security data preprocessing and feature extraction.
3. Cognito by Vectra: AI solution for threat detection and response across diverse network environments.
Use Cases of ML in Security and Cybersecurity
Machine learning (ML) is transforming cybersecurity by providing effective solutions to combat various threats. Here are some key applications of ML in cybersecurity and security:
1. Abnormal/Malicious Activity Detection
Problem Statement: Abnormal and malicious activities remain a critical challenge in security and cybersecurity.
Solution: ML algorithms can identify and prevent unusual activities to protect from potential attacks proactively. At the forefront of ML applications in security and cybersecurity is the detection of abnormal and malicious activities. ML algorithms are trained to recognize patterns of normal behavior within a network or system. By continuously analyzing activities, these algorithms can quickly identify deviations that may indicate a cyberattack or breach. This real-time detection capability enables organizations to respond swiftly to mitigate potential threats, dramatically reducing the risk of significant damage.
2. SMS Fraud Detection:
Problem Statement: With the increasing prevalence of mobile devices, SMS fraud has become a common attack vector for cybercriminals.
Solution: ML models are now being trained to distinguish between legitimate and fraudulent SMS messages. By analyzing message content, sender information, and sending patterns, these models can alert users to potential scams before they fall victim to phishing attempts or malicious links. This application of ML not only protects individual users but also helps maintain the integrity of communication networks.
3. Human Error Prevention:
Problem Statement: The challenge lies in mitigating human errors in cybersecurity processes, particularly in the identification and prevention of security threats within large datasets.
Solution: ML platforms are crucial in preventing human errors by efficiently filtering out malicious activities from large datasets. These platforms can automatically analyze and detect potential security threats, reducing the need for manual analysis and minimizing the risk of human errors. This proactive approach enhances the accuracy and effectiveness of threat detection processes, ultimately strengthening overall cybersecurity defenses.
4. Anti-Virus and Malware Detection:
Problem Statement: Traditional anti-virus and malware detection software relies on signature-based methods that often fail to catch new or evolving threats.
Solution: ML enhances these tools by employing anomaly detection and behavior tracking algorithms. These sophisticated ML models can analyze the characteristics and actions of software, identifying malicious programs based on their behavior rather than relying on known signatures. This approach significantly improves the detection rates of zero-day threats, offering a dynamic defense mechanism against malware.
5. Email Monitoring:
Problem Statement: Email remains a primary attack vector for cyber threats, with phishing attempts becoming increasingly sophisticated.
Solution: ML models, particularly those utilizing Natural Language Processing (NLP) algorithms, are now employed to scrutinize email content, sender information, and other metadata to identify phishing attempts. By learning from vast datasets of known phishing emails and legitimate communications, ML models can accurately flag suspicious emails, protecting users from potential fraud and information theft.
6. Bot Detection:
Problem Statement: Not all bots are created equally; some perform legitimate functions, while others are designed for malicious purposes. It is essential to differentiate between 'beneficial' and 'malicious' bots to uphold the security and operational integrity of online services.
Solution: ML algorithms analyze behavioral patterns, such as the frequency of requests, the nature of the interactions, and the speed of actions, to identify and block malicious bots. This ensures that services remain available to legitimate users and bots while preventing spam, data scraping, and DDoS attacks.
7. Network Threat Detection:
Problem Statement: As networks become more complex, identifying threats amidst the vast amount of legitimate traffic is increasingly challenging.
Solution: Machine learning models are highly proficient in scrutinizing network data traffic to pinpoint potentially malicious patterns indicative of cyber threats. Through ongoing data flow monitoring, these models can detect irregularities like abnormal data transfers or sudden spikes in traffic directed towards particular destinations. This capability empowers IT teams to swiftly investigate and address potential threats.
Benefits of Machine Learning in Security
The various benefits of Machine Learning based Security are:
1. Continuous Improvement: AI/ML technology evolves over time by learning from business network behaviors and identifying web patterns, making it challenging for hackers to breach the network.
2. Scalability: AI/ML can efficiently handle large volumes of data, enabling Next-Generation Firewall (NGFW) systems to scan numerous files daily without causing disruptions to network users.
3. Enhanced Detection and Response: Implementing AI/ML software in firewalls and anti-malware solutions accelerates threat identification and response times, reducing the reliance on human intervention and enhancing overall effectiveness.
4. Comprehensive Security: AI/ML solutions provide security at both macro and micro levels, creating barriers against malware infiltration and enabling IT professionals to focus on addressing complex threats, thereby enhancing the overall security posture.
What are the best practices Machine Learning in Security ?
1. Secure Data: Safeguard the data utilized in your machine learning models by implementing appropriate access controls and encryption measures to mitigate the risk of unauthorized access or data breaches.
2. Auditing: Consistently audit and assess your ML systems to verify their proper functionality and absence of vulnerabilities.
3. Monitoring: Continuously monitor the status of your ML systems to detect any potential issues or anomalies in real-time.
4. Testing: It is crucial to conduct unit and integration testing to ensure proper software functionality and to detect any potential issues.
5. Patching: Keep your ML system up to date by applying patches and updates to address any known vulnerabilities or bugs.
6. User Authentication and Encryption: Authenticate users who access your ML models and encrypt authorized user sessions to protect against unauthorized access or data interception.
7. Protect Against Attacks: Implement measures to defend against malicious code, data theft, and insider threats that could compromise the security of your ML system.
8. Choose Reliable Companies and Technologies: Select reputable ML service providers and ensure they have robust security measures in place. Keep yourself informed about the most recent security technologies and optimal procedures.
9. Transparent Auditing: Use ML services that offer transparent auditing processes to track service improvements and address emerging threats.
10. Regular Review and Updates: Regularly review and update firewall rules, software versions, and security patches to stay protected against potential vulnerabilities.
For example, when employing a web server for your website, it's crucial to integrate filters that can intercept requests originating from sources other than your server and those that diverge from typical traffic patterns, like spam. Stay informed about security vulnerabilities in the open-source projects you utilize and promptly apply patches to address any identified issues. This proactive approach helps prevent exploitation by attackers who may gain access through various means like phishing attacks.
Adhering to these recommended guidelines simplifies the process of fortifying the security of machine learning systems, effectively reducing the potential risks linked to data breaches and cyber-attacks.
Challenges for adopting Machine Learning in Security and Cybersecurity
While machine learning (ML) holds immense potential to revolutionize cybersecurity and security, navigating its deployment and ensuring its effectiveness are not without challenges. The road to integrating ML into security protocols is fraught with hurdles, from data-related issues to the inherent complexities of ML systems. Shedding light on the obstacles that professionals face in harnessing the full power of ML for cybersecurity and security.
- Insufficient Training Data: ML algorithms require a significant amount of diverse training data to perform effectively. Inadequate data can lead to biased predictions and hinder model accuracy.
- Data Quality Concerns: The quality of training data greatly impacts the performance of ML models. Errors, outliers, or noise in the data can compromise model accuracy, emphasizing the importance of high-quality training data.
- Complexity of Machine Learning: The dynamic nature of machine learning presents a complex and evolving process for practitioners. Tasks such as data analysis, preprocessing, and complex computations contribute to the challenges faced in ML implementation.
- AI/ML Vulnerabilities: Security risks associated with AI and ML technologies extend to various applications, with adversarial attacks targeting machine learning systems posing a significant threat. Unintentional information leakage and data breaches due to employee negligence are common vulnerabilities.
- Adversarial Attacks on ML Systems: ML systems are vulnerable to adversarial attacks that manipulate model behavior, particularly targeting visual input systems and analytics. Hackers exploit vulnerabilities in machine learning algorithms, posing a growing security threat.
- Data Poisoning and Model Integrity: Relying on publicly available datasets for training can introduce the risk of tainted data. Deliberate data poisoning during training can compromise model integrity, allowing malicious actors to exploit vulnerabilities in AI systems.
Future of Machine Learning in Security and Cybersecurity
It is crucial to recognize that as technology, particularly advancements in AI and ML, continues to progress rapidly, its impact is shaped by the intentions and actions of those who control and utilize it.
Scope in Security with ML
The realm of machine learning within security systems holds considerable promise. ML algorithms play a pivotal role in enhancing threat detection capabilities by scrutinizing extensive data sets to discern patterns and anomalies that may signify potential security breaches. The prospective trajectory of ML in security systems hinges on its capacity to adjust and develop countermeasures against sophisticated cyber threats, automate incident response procedures, enhance user authentication protocols, and streamline security orchestration. This evolution could render security systems more intelligent, adaptable, and proficient in safeguarding sensitive data and digital assets.
Scope in Cybersecurity with ML
The ethical use of technology ultimately depends on the discretion and intentions of those who control it. The Cyber Security Industry is facing a shortage of skilled professionals, and technology can play a crucial role in easing this burden and advancing to prevent cyber-attacks effectively. Despite the benefits, hackers are also leveraging advanced technology for complex attacks. Botnets utilizing sophisticated algorithms can identify network vulnerabilities and exploit them. Deep Neural Network (DNN) algorithms must be able to differentiate between benign and malicious DNNs to enhance AI's effectiveness in cybersecurity. It is essential for cybersecurity measures to anticipate and counteract potential threats, when adversaries attempt to weaponize AI against them.
What's Next?