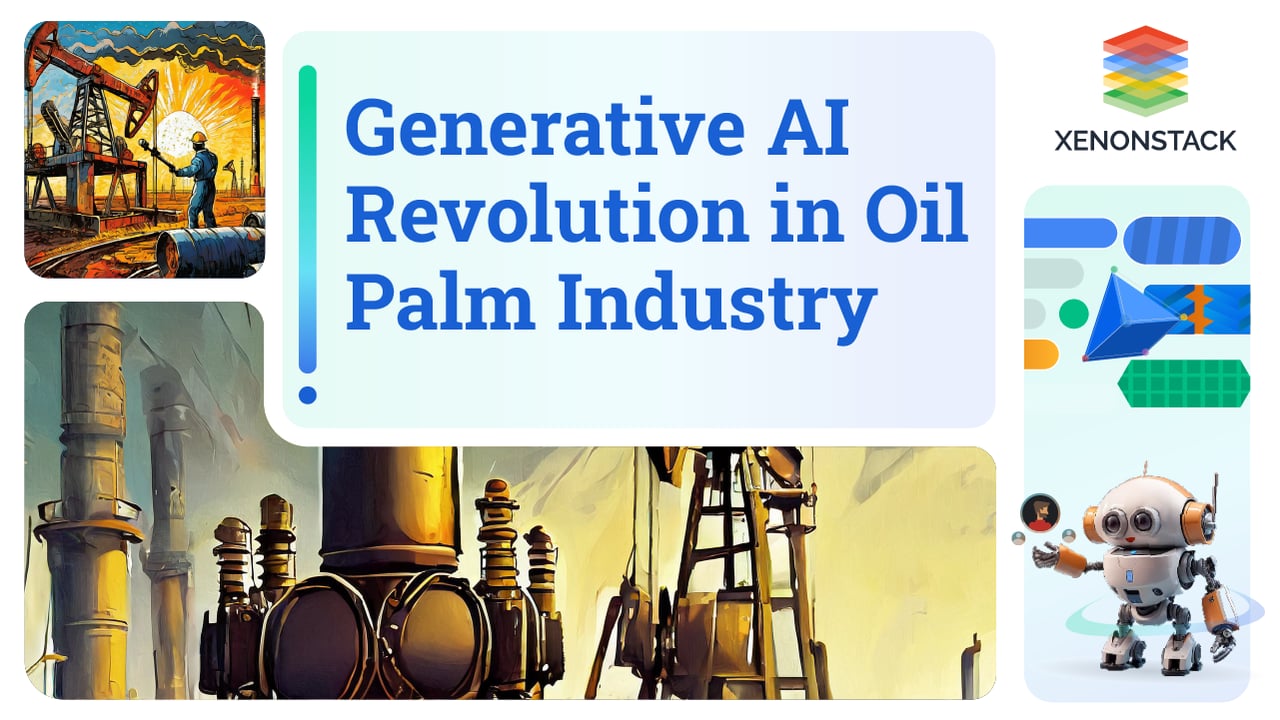
Introduction
In the recent time generative AI has become a revolutionary technology which has impacted all sectors of existence, such as software development, healthcare, transport and navigation and the energy industry is no different. Generative AI has made its impact on the energy industry as well. The integration of Generative AI (Gen AI) into the oil palm industry and broader energy sector has offered a transformative way towards sustainability and innovation.
Generative AI not only provides the basis for innovation but also has a huge role to play when it comes to improving the scale of yields returned from various energy sectors. Generative AI comes with its own baggage of other AI concepts which can be implemented in the “to and fro” of industries and production units such as well such as implementing Predictive Maintenance Strategies, Renewable Energy Integration, Supply Chain Optimisation, Environmental Impact Assessment, Smart Grid Management, Carbon Footprint Reduction and Precision Agriculture Techniques. The below sections describe the use of generative AI in all these aspects.
Energy Industry
The global energy industry is responsible for providing to the basic human needs and it accounts for resources such as electricity, fuel, healthcare and much more. The energy industry in general is responsible for extracting, producing, refining and distribution of global energy resources. Oil palm is one such industry which is involved in the production of products such as soaps, candles, lubricants etc. The industry is not just involved in the production but also supplies raw material to other energy productions as thermal power plants.
Generative AI is a technology which holds the capability to produce text, images, audio, video animations in response to the prompt it receives, hence the name “Generative AI.”
The energy industry can benefit from the power that generative AI provides, optimization of energy consumption, forecasting sales and demand being a few examples. According to sources a substantial portion of the energy industry is willing to adapt and harness the power of generative AI for its operations.
There are two essentials that energy industry must adopt to harness the capabilities of generative AI. Firstly, developing strong data strategies which involves extracting data from sources such as smart grids and customer behaviour to derive important data driven key points and make decisions based upon those. secondly, implementing AI practices within the organisation involving following the best practices and implementing gen AI in an efficient manner.
Enhancing Yield Efficiency
Generative AI plays a vital role in boosting yield within the energy industry by optimizing processes and accurately predicting outcomes. It is instrumental in developing advanced energy production models like wind farms and solar arrays, leveraging data analysis to determine the best placements for turbines or panels to maximize energy capture. Additionally, generative AI enhances predictive maintenance strategies for energy infrastructure, identifying potential issues pre-emptively to avoid downtime and inefficiencies. Harnessing generative AI techniques enables energy companies to achieve higher yields, reduce operational costs, and improve overall performance in a rapidly evolving industry landscape.
Generative AI can boost yield gained from industries employing its AI driven in-sights. In case of oil palm industry generative AI can play a key role in helping producers select the most optimal crop verities, crop optimization, as well as providing essential in-sights for production techniques for required conditions and needs.
This process would involve providing the generative AI algorithms with the data related to past yields, genetic information for crops, historic climatic data. Once this data is processed by generative AI’s models, it can provide helpful insights which could involve simulations which would guide producers and farmers in optimizing their crop production, improving the agricultural productivity, cope up with the ever changing and evolving market demand and trends and gain better yields.
Predictive Maintenance Strategies
Predictive maintenance is a strategic approach that utilizes past data to forecast future equipment conditions, pre-empting issues before they arise. While this method has a long history dating back a century, it is currently undergoing significant evolution with the integration of generative AI technology.
AI-driven predictive maintenance harnesses machine learning (ML) algorithms and AI frameworks to assess operational conditions, compare performance with established benchmarks, detect early signs of failure, pinpoint subtle decreases in efficiency, and recommend maintenance actions to proactively address requirements before potential failures occur.
Generative AI proves invaluable in the energy and utilities sector by analysing data from critical assets such as turbines and pumps, alongside historical maintenance records and environmental factors, to forecast equipment maintenance needs. This predictive maintenance approach empowers energy and utility companies to optimize maintenance schedules, enhance energy supply reliability, reduce waste, and cut costs effectively.
Different Predictive Maintenance strategies
Figure: Flow diagrams for various predictive maintenance strategies
Brief Description
Figure is describing flow diagrams for various predictive maintenance strategies that uses past data to predict future equipment conditions and employ mitigation strategies for them.
-
Preventive maintenance considers the historical data to predict future equipment failures and deploy maintenance procedures for them.
-
In corrective maintenance approach maintenance procedures are employed when faulty equipment or disruptions are detected. The equipment is then corrected and brought back to a desired state.
-
In risk-based maintenance strategy risk-based evaluations are performed to detect equipment vulnerable to risks and mitigation strategies are developed to cope with them.
- In condition-based maintenance real-time metrics are used to detect if equipment is operating under desired states, if not maintenance strategies are used.
This sub-section describes the several types of predictive maintenance strategies. These strategies comprise of approaches used to forecast failures in equipment.
-
Preventive maintenance
The strategy works on mitigation of failures by developing Maintenace programs, which are based on historical data to derive potential equipment failures and their prevention. This eventually leads to sustainable productivity.
-
Corrective maintenance
This strategy works as to correct faults when detected in equipment, which means when faults or disruptions are detected in equipment those are corrected and the equipment is brought back to a desired state. Corrective maintenance can be both planned (having a corrective Maintenace plan already in hand) or unplanned, although unplanned corrective Maintenace incurs much more costs.
-
Risk-based maintenance
The focus of this maintenance strategy is based on identifying equipment vulnerable to risks and to maintain safety of the employees as well as the assets and resources employed in the company.
-
Condition-based maintenance
The focus of condition-based maintenance is to check real time metrics such as vibration monitoring, to identify if the equipment falls under the desired zone. Based on these metrics maintenance systems are employed only when required and when the equipment behaves unexpectedly.
Advantages of using Generative AI for Predictive Maintenance
Here are five advantages of leveraging Generative AI for Predictive Maintenance:
1. Extends Equipment Lifespan: AI-based predictive maintenance enables companies to identify and resolve issues proactively, preventing them from escalating into costly problems and prolonging equipment lifespan.
2. Optimizes Energy Efficiency: By closely monitoring equipment performance indicators like energy consumption, AI predictive maintenance swiftly detects deviations from optimal energy usage, leading to significant cost savings and environmental benefits.
3. Minimizes Downtime: Generative AI solutions facilitate strategic maintenance scheduling, predicting equipment failures in advance to avoid unplanned downtime, ensuring continuous operations and minimal productivity losses.
4. Enhances Safety Measures: AI predictive maintenance aids in addressing potential equipment failures proactively, contributing to a safer work environment and reducing hazards.
5. Improves Decision-Making: Leveraging generative AI provides businesses with data-rich insights into equipment performance and behaviour, facilitating better decision-making regarding resource allocation, maintenance strategies, and overall operational efficiency.
Renewable Energy Integration
Renewable energy integration involves incorporating renewable energy sources into established electrical grids. These sources, such as hydropower, geothermal, wind, and solar energy, derive power from self-replenishing natural sources. When appropriately integrated, these sources have the potential to provide ample energy for a sustainable future. However, their characteristics differ significantly from fossil fuels, presenting both advantages and challenges when added to the current power grid infrastructure.
Consider wind turbines as an example of renewable energy technology. Wind power is harnessed by propeller-like blades turning around a rotor, which then spins a generator to produce electricity. The electrical energy is then transformed through a converter to match the voltage requirements of the electricity system. While wind energy offers clean and renewable power domestically, its reliability is hindered by its variability due to fluctuating wind speeds. Despite efforts to build wind farms in optimal locations, this inconsistency underscores the necessity of integrating wind power as part of a broader renewable energy strategy.
How does Renewable Energy Integration work
The stability and efficiency of power grids rely on maintaining a steady energy supply for the seamless generation, transmission, and distribution of electricity to consumers. Integrating renewable energy sources effectively necessitates the deployment of advanced grid optimization technologies such as real-time data analytics, automation, and sophisticated sensors. By harnessing these intelligent technologies, energy distribution can be optimized, grid management efficiency improved, and the transition to a more sustainable energy future accelerated.
Figure: Flow diagram describing the steps employed for renewable energy integration
Brief Description
Figure is describing flow diagram for the steps employed for renewable energy integration which involves the integration of renewable energy sources into existing electrical power grids.
-
Evaluations are performed to detect and assess available renewable energy sources. Along with this the electrical grid infrastructure is analysed for compatibility.
-
Appropriate renewable energy technologies such as wind turbines and solar panels are chosen based on resource assessments and grid compatibility analyses. Interconnection for energy resources and power grids is also planned.
-
Energy storage solutions such as batteries are deployed and smart grid technologies like real-time monitoring systems are implemented.
-
Strategies and tools for real-time balancing of energy supply and demand are developed.
Consider the below steps employed for renewable energy integration
-
Assessing Renewable Resources
Conducting thorough evaluations to identify and assess available renewable energy sources such as wind, solar, hydro, and geothermal in specific areas. This analysis includes studying historical data, climate conditions, and terrain features to determine optimal locations for renewable energy installations.
-
Analysing Grid Compatibility
Evaluating the existing electrical grid infrastructure to understand its capacity, constraints, and suitability for integrating renewable energy sources. This analysis considers factors like voltage levels, grid stability, transmission capabilities, and potential integration points.
-
Selecting Suitable Technologies
Choosing appropriate renewable energy technologies based on resource assessments and grid compatibility analyses. This step involves selecting from options like wind turbines, solar panels, hydroelectric generators, or geothermal systems that align with local resources and grid requirements.
-
Planning Interconnection
Developing plans for seamlessly connecting renewable energy systems to the grid while ensuring compatibility with existing power generation and distribution systems. This includes designing interconnection points, establishing energy flow protocols, and adhering to regulatory standards and grid codes.
-
Implementing Energy Storage
Deploying energy storage solutions such as batteries, pumped hydro storage, or thermal storage systems to manage intermittency issues associated with renewable energy sources. Energy storage helps store surplus energy during peak generation periods and release it during high demand or low renewable energy production times.
-
Integrating Smart Grid Technologies
Implementing smart grid technologies like advanced metering infrastructure (AMI), real-time monitoring systems, smart sensors, and automation controls to optimize energy management, improve grid resilience, and enable responsive demand-side measures.
-
Managing Grid Balancing
Developing strategies and tools for real-time balancing of energy supply and demand, considering the variability of renewable energy generation. This involves utilizing predictive analytics, energy forecasting models, and grid optimization algorithms to optimize generation schedules, maintain grid stability, and reduce energy wastage.
Supply Chain Optimization
Problem statement
Business leaders face challenges in optimizing supply chain operations due to complexities in demand forecasting, production scheduling, and risk management. Traditional methods often fall short in handling unforeseen disruptions, leading to inefficiencies, increased costs, and customer dissatisfaction.
Solution
Integration of AI technologies, specifically Generative AI (Gen AI), into core components of supply chain operations offers a transformative solution. Gen AI tools leverage advanced algorithms and machine learning capabilities to streamline interactions, improve demand forecasting accuracy, optimize inventory levels, enhance production scheduling, and mitigate supply chain risks.
Leveraging Generative AI in supply chain management not only enhances operational efficiency but also strengthens resilience, reduces costs, and drives sustainable practices, positioning organizations to thrive in an increasingly dynamic business environment.
Impact
1. Improved Planning and Forecasting
Gen AI simplifies interactions and aids in developing real-time demand models, leading to optimal inventory levels, production schedules, and distribution plans that align with customer demand fluctuations and market trends.
2. Enhanced Production Efficiency
AI-driven production planning considers various factors such as customer fluctuations, production capacities, resource availability, and order priorities to reduce bottlenecks, optimize resource allocation, and improve production effectiveness.
3. Risk Management and Resilience
Gen AI tools can anticipate and manage supply chain risks by running scenarios, suggesting multiple courses of action during disruptions, and enhancing overall risk management capabilities.
4. Cost Reduction and Sustainability
By optimizing supply chain processes, AI integration leads to reduced operational costs, improved resource utilization, minimized wastage, and enhanced sustainability practices, ultimately contributing to long-term cost structures and resilience.
Environmental Impact Assessment
Environmental Impact Assessment (EIA) is increasingly benefiting from the integration of generative AI technology across various industries. Leveraging gen AI allows organizations to conduct more comprehensive and precise assessments of their activities' environmental impacts.
Gen AI integration is revolutionizing the process of conducting environmental impact assessments in the energy sector. It empowers energy companies to analyse and understand the environmental consequences of different energy sources and infrastructure projects with unparalleled accuracy and detail. For instance, gen AI can simulate and evaluate the environmental impact of developing and operating new renewable energy facilities such as solar farms or wind turbine arrays. This simulation considers several factors such as changes in land use, impacts on wildlife habitats, water usage, and carbon emissions throughout the project's lifecycle. This comprehensive analysis enables informed decision-making to optimize energy production processes while minimizing adverse environmental impacts. Gen AI also aids in emissions forecasting, optimizing energy distribution networks, and identifying opportunities for integrating renewable energy sources to promote sustainability in the energy sector.
Within the manufacturing sector, gen AI plays a pivotal role in assessing and mitigating environmental impacts associated with production processes and supply chains. Gen AI algorithms analyse extensive datasets related to material sourcing, production methods, energy consumption, waste generation, and emissions to identify areas for improvement. For example, gen AI can optimize manufacturing workflows to reduce energy consumption, predict maintenance requirements for machinery to minimize waste generation, and suggest sustainable alternatives for raw materials or packaging materials. Through thorough environmental impact assessments facilitated by gen AI, manufacturing firms can significantly reduce their ecological footprint, comply with environmental regulations, and meet consumer demands for sustainable products and practices.
Smart Grid Management
Known by various names such as power grid, power distribution grid, electrical grid, or national grid, this interconnected electrical network is responsible for generating and distributing electricity across expansive regions. It encompasses energy utility companies and suppliers tasked with delivering electricity to residential and commercial properties.
Furthermore, the power grid encompasses the infrastructure necessary for power generation and distribution.
The utilization of AI is revolutionizing the electricity production and consumption landscape. When AI techniques are integrated into smart grid applications, we can attain an energy system that is not only more reliable, resilient, efficient but also sustainable, benefiting both utility companies and consumers.
AI plays a transformative role across various critical aspects of smart grid management. Firstly, in demand forecasting, AI leverages historical data, weather information, and consumer behaviour patterns to accurately predict electricity demand across different timescales and locations, aiding utility companies in effective supply-demand balance and future planning. Secondly, AI contributes to renewable energy integration by managing the variability and uncertainty of renewable sources like wind and solar. It forecasts their output based on weather conditions, adjusts other generators' output to compensate for fluctuations, and coordinates energy storage devices to ensure a smooth supply. Additionally, AI enhances grid security by detecting and preventing cyberattacks through network monitoring and identifying physical faults using sensors and self-healing techniques. Moreover, AI optimizes grid performance and efficiency by finding the optimal configuration of assets and minimizing losses, congestion, and voltage deviations. Lastly, AI enhances customer engagement by providing personalized energy consumption feedback, suggesting optimal usage schedules, and encouraging participation in demand response programs, promoting energy efficiency and cost savings for consumers.
Carbon Footprint Reduction
Figure: Carbon footprint reduction
Brief Description
Figure is describing carbon footprint, its causes and ways carbon footprint can be reduced.
-
Carbon footprint is a measure of the amount of carbon di-oxide produced due to human activities.
-
Carbon footprint accounts for gaseous emissions which are a by-product of human resource utilisation such as burning fuels for transportation, electricity etc.
-
Carbon footprint can be reduced by following environment healthy ways such as recycling, reusing and proper waste disposal.
Problem statement
Industries across diverse sectors are under increasing pressure to diminish their carbon footprints and adopt sustainable practices to mitigate environmental impacts. However, conventional methods struggle to effectively analyse complex datasets related to energy consumption, emissions, and operational processes, leading to challenges in pinpointing areas for improvement and implementing targeted strategies.
Solution
Harnessing the capabilities of Generative AI (Gen AI) presents a promising solution to tackle the challenges of reducing carbon footprints across industries. Gen AI excels in analysing extensive datasets using advanced algorithms and machine learning capabilities, enabling precise identification of areas ready for improvement and optimization in energy usage, resource utilization, and emissions reduction.
Impact
1. Energy Efficiency Enhancement
Gen AI analyses energy consumption patterns and operational processes to devise innovative strategies for reducing energy waste and optimizing resource utilization across supply chains and production cycles. This includes optimizing transportation routes, implementing energy-efficient protocols in manufacturing, and integrating renewable energy sources where viable.
2. Emissions Mitigation
By optimizing processes and resource usage, Gen AI assists industries in reducing carbon emissions across their operations. This involves identifying emission reduction opportunities in manufacturing, logistics, and energy consumption, contributing to overall environmental sustainability targets.
3. Predictive Maintenance and Downtime Reduction
Gen AI enables predictive maintenance for infrastructure and machinery, reducing downtime, extending equipment lifespan, and minimizing energy inefficiencies linked to equipment failures.
4. Operational Efficiency and Cost Reduction
Integrating Gen AI into carbon footprint reduction efforts not only delivers significant environmental benefits but also drives operational efficiency and cost savings for businesses. By streamlining processes, optimizing resources, and reducing waste, businesses can achieve environmental sustainability alongside economic competitiveness.
Precision Agriculture Techniques
Precision agriculture, commonly known as "smart farming," is a data-centric strategy that adjusts agricultural practices according to the unique requirements of specific plants or regions within a field. In contrast to traditional farming methods that treat entire fields uniformly, this approach utilizes AI-powered tools to analyse various data points such as crop health, weather patterns, and soil conditions in real-time. This provides farmers with the capability to make informed decisions, enhance productivity, and manage resources efficiently.
Machine learning and data analytics, examples of AI technology, are fundamental to the success of modern agriculture. These algorithms process vast amounts of data collected from sensors, satellites, and drones to identify patterns and connections. This data-driven knowledge equips farmers to take precise actions by understanding the specific needs of their farms.
Gen AI can further enhance these capabilities by generating insights from complex datasets, optimizing algorithms for more accurate predictions, and automating decision-making processes. For instance, AI-driven drones equipped with various sensors can capture detailed field images, which are then analysed by Gen AI to detect signs of pest infestations, nutrient deficiencies, or disease outbreaks with greater accuracy and efficiency. Early detection enables farmers to apply targeted remedies in affected areas, reducing reliance on pesticides and fertilizers and minimizing environmental impacts.
One of the key advantages of precision agriculture is its ability to efficiently allocate resources. AI systems analyse data from sensors embedded in the soil to assess temperature, nutrient levels, and moisture content. By leveraging this data, farmers can accurately determine the optimal amount of water and fertilizer required, leading to reduced waste and cost savings.
Figure: Flow diagrams for various precision agriculture techniques
Brief Description
Figure is describing flow diagrams for various precision agriculture techniques which focus on optimizing agricultural practices.
-
Variable Rate Technology involves adjusting inputs such as fertilizers based on agricultural data analysis results.
-
Remote Sensing utilises satellite imagery, drones etc. to gather data on crop health, soil moisture etc. For making informed decisions on irrigation, fertilisation and resource allocation.
Precision irrigation entails the deployment of irrigation systems that distribute water precisely at specific locations and times, guided by data analysis of moisture levels, weather forecasts, and other factors.
- GPS guidance systems involves use of GPS technology to precisely navigate farm machinery such as tractors and planters, ensuring accurate agricultural practices.
Consider the below precision agricultural techniques which focus on optimizing agricultural practices.
-
Variable Rate Technology (VRT)
VRT involves adjusting inputs such as fertilizers, pesticides, and water based on specific needs identified through data analysis. This allows for targeted application rates, optimizing resource use and reducing waste.
-
Remote Sensing
Utilizing satellite imagery, drones, and sensors to gather data on crop health, soil moisture, nutrient levels, and pest infestations across large agricultural areas. This data is then analysed to make informed decisions regarding irrigation, fertilization, and pest control.
-
Precision Irrigation
Implementing irrigation systems that deliver water precisely where and when it is needed based on soil moisture levels, weather conditions, and crop water requirements. This prevents overwatering, reduces water wastage, and promotes healthier crop growth.
-
GPS Guidance Systems
Using GPS technology to precisely navigate farm machinery such as tractors and planters, ensuring accurate planting, spraying, and harvesting operations. This minimizes overlaps, reduces fuel consumption, and improves overall efficiency.
Conclusion
The integration of Generative AI (Gen AI) into the oil palm industry and broader energy sector offers a transformative pathway towards sustainability and innovation. Gen AI optimizes yield efficiency by leveraging data analytics and simulations to guide producers in crop selection, optimization, and production techniques, thus enhancing agricultural productivity and meeting market demands. Furthermore, Gen AI-driven predictive maintenance strategies enable proactive equipment maintenance, extending lifespan, optimizing energy usage, minimizing downtime, enhancing safety measures, and improving decision-making processes.
Renewable energy integration benefits from Gen AI through grid optimization, managing variability, and forecasting output based on weather conditions, while supply chain optimization optimizes inventory levels, production schedules, and distribution plans, reducing bottlenecks and enhancing efficiency. Environmental impact assessment and smart grid management benefit from Gen AI's data-driven insights, aiding in emissions forecasting, energy distribution optimization, and grid security. Finally, Gen AI contributes to carbon footprint reduction by optimizing energy consumption, resource utilization, and promoting renewable energy integration, while precision agriculture techniques benefit from AI-driven insights for efficient resource allocation, pest detection, and environmental impact minimization, collectively driving sustainable energy solutions and operational excellence across industries.
-
Learn about the Role of Generative AI in Generate Synthetic Data