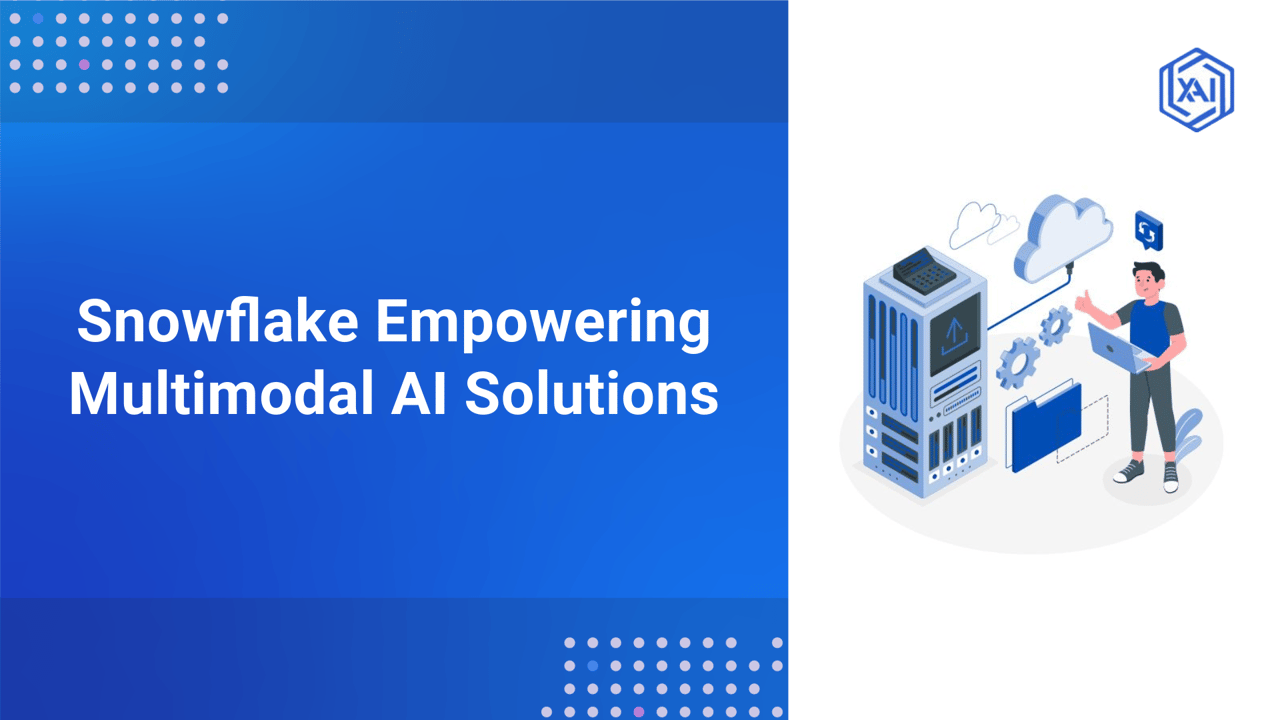
Unlocking the Power of Multimodal AI with Snowflake
AI applications receive unprecedented opportunities through text, image, video, and structured data, which are exploding across the market today. However, organizations face challenges in efficiently handling multiple data types within their databases. As a top cloud data platform, Snowflake provides businesses with an advanced system to manage multimodal data, ensuring high-quality data with Snowflake's data validation for accurate AI-based insights.
The Growing Need for Effective Multimodal Data Management
Current business operations generate enormous quantities of data in various formats. The successful implementation of AI-driven decision systems requires companies to manage diverse customer and operational information, including social media content. Traditional Snowflake Schema-based data management approaches often struggle with processing large volumes of multimodal data, creating inefficiencies in computer vision AI pipelines and other AI-driven workflows.
To overcome these challenges, businesses are integrating Edge AI for computer vision applications, allowing real-time processing closer to data sources. Additionally, AI for real-time data quality monitoring ensures that AI models receive accurate and validated information, enhancing predictive capabilities.
How Snowflake Addresses the Challenges of Managing Multimodal Data
Snowflake’s cloud-native architecture unifies structured and unstructured data, providing a scalable and high-performance environment for AI applications. By breaking data silos and enabling seamless integration, it enhances Generative AI, fostering more advanced and context-aware AI models.
Moreover, data quality checks in Snowflake workflows help maintain data integrity, ensuring that multimodal AI models function optimally. Snowflake’s interoperability with Snowflake Open Catalog & Iceberg Tables further enhances its ability to connect diverse datasets, making AI-driven analytics more efficient. This streamlined data management approach empowers businesses to leverage AI for deeper insights and improved decision-making.
Key Snowflake Capabilities for Multimodal Data Management
Handling Structured and Unstructured Data Seamlessly
With its platform Snowflake allows organizations to manage both structured database spreadsheets alongside unstructured image video and document files. A single platform implementation removes the requirement for multiple storage systems thus it enables smooth AI workflow operations alongside consistent data maintenance.
Scalability and Performance for Large-Scale Multimodal Datasets
Snowflake employs a cloud-centered infrastructure that lets its users expand storage and computational power separately to optimize AI workload efficiency. The Snowflake platform delivers efficient infrastructure solutions for processing both terabyte and petabyte data scales that support AI application deployment.
Simplifying Multimodal AI Data Integration with Snowflake
Connecting Diverse Data Sources for Multimodal AI
The implementation of AI models requires datasets from different sources that encompass enterprise databases and IoT sensors as well as external APIs. The numerous connection capabilities of Snowflake allow organizations to integrate data sources easily which yields a complete multimodal AI framework.
Simplifying Data Pipelines and Reducing Complexity
The data ingestion service Snowpipe enables Snowflake to streamline complex data pipeline management. Interoperability with Snowflake Open Catalog & Iceberg Tables allows organizations to seamlessly access and manage data across multiple sources. Snowflake enables faster data processing through automation of data ingestions as well as transformations, which simplifies the complexity of artificial intelligence workflows.
Optimizing AI Querying and Analysis with Snowflake
Leveraging Snowflake's SQL for Multimodal Data Querying
The SQL-based querying capability in Snowflake allows practitioners to work with multimodal datasets through their regular analysis tools. Therapeutic SQL capabilities allow companies to retrieve important insights from established and unstructured information bases beyond the necessity of specialized programming knowledge.
Performing Complex Analytics on Multimodal Datasets
Snowflake delivers advanced analytics functions for business users to perform advanced queries which enable them to connect different data types while generating AI-processed insights. Snowflake optimizes predictive analytics decision-making through its ability to integrate with machine learning platforms.
Enhancing Collaboration Between Data Teams and AI Practitioners
-
Providing a Single Source of Truth for Multimodal Data: A significant obstacle in AI development arises when different teams struggle to maintain consistent datasets. Snowflake’s centralized data repository delivers a unified source of high-quality data with Snowflake's data validation, ensuring accuracy across engineering teams, analysts, and AI developers. This consistency is crucial for building multimodal AI solutions that rely on diverse data sources.
-
Facilitating Seamless Data Sharing and Access Control: Snowflake enables teams to securely share and collaborate on integrating text, image, and video data in Snowflake, ensuring AI models operate on complete and reliable datasets. With data governance with Snowflake's quality management, organizations can enforce strict access controls and protect sensitive information while maintaining operational efficiency.
Seamless AI and ML Integration with Snowflake
Connecting Snowflake with Popular AI Frameworks and Tools
Snowflake enables easy connection to leading AI and machine learning platforms such as TensorFlow and PyTorch and DataRobot. Through its connectivity interface Snowflake enables data teams to access its storage capacity as they run AI models from their chosen environments.
Enabling Smooth Data Flow Between Snowflake and AI Systems
The data exchange capabilities of Snowflake support fluid data transmission among cloud-based AI systems that handle storage functionality as well as processing operations and AI inference functions. The implementation of AI for real-time data quality monitoring ensures that AI applications obtain the most up-to-date information, improving both their operational performance and accuracy measurements.
Real-World Examples of Multimodal AI Applications Powered by Snowflake
Showcasing Successful Multimodal AI ImplementationsMany organizations in different industries utilize Snowflake as their power source for multimodal AI applications. Medical organizations utilize Snowflake to link imaging data with patient data for AI diagnosis purposes while e-commerce companies apply it to process image data and customer behavior information for tailor-made recommendations. Highlighting the Benefits of Using Snowflake for Multimodal AISnowflake combines three essential functionalities to boost AI modeling speed and simplify operations and enhance organizational decision quality. The benefits supported by Snowflake solutions make it an optimal selection for organizations that implement multimodal AI solutions.
Best Practices for Deploying Multimodal AI on Snowflake
As organizations increasingly adopt multimodal AI solutions, effective data management strategies are essential for achieving seamless integration, regulatory compliance, and operational efficiency. Snowflake provides optimized storage approaches and robust security features, but organizations must also develop comprehensive data governance policies to ensure proper data handling, privacy protection, and compliance with industry regulations
Key Strategies for Efficient Multimodal Data Management
-
Data Classification and Organization: Establish a structured approach to categorizing multimodal data (text, images, video, and audio) to streamline AI model training and inference.
-
Data Lifecycle Management: Implement automated policies for data retention, archiving, and deletion to maintain data integrity and optimize storage costs.
-
Access Control and Security: Leverage Snowflake’s role-based access control (RBAC) and encryption to safeguard sensitive AI datasets.
-
Data Lineage and Auditability: Maintain transparent tracking of data sources, transformations, and usage to facilitate compliance with regulations such as GDPR and CCPA.
-
Optimization for AI Workflows: Utilize Snowflake’s scalable architecture to ensure efficient storage, retrieval, and processing of large-scale multimodal datasets.
Tips for Integrating Snowflake with AI Workflows
Integrating Snowflake with AI workflows requires careful planning to maximize performance, efficiency, and collaboration. Snowflake’s cloud-native architecture and advanced capabilities provide an ideal foundation for AI-driven analytics.
Best Practices for AI Integration with Snowflake
-
Real-Time Data Ingestion: Use Snowpipe to enable automated and continuous data ingestion from various sources into Snowflake, ensuring AI models are trained on the most up-to-date information.
-
Performance Tuning: Improve query performance using Snowflake’s indexing, materialized views, and query caching to accelerate AI-driven insights.
-
Cross-Team Collaboration: Utilize Snowflake’s data sharing capabilities to enable multiple teams to access, analyze, and collaborate on shared datasets without unnecessary duplication.
-
Seamless AI Tool Integration: Leverage Snowflake’s built-in connectors and APIs to integrate with leading AI/ML platforms, such as Databricks, Amazon SageMaker, and TensorFlow, enabling a streamlined AI pipeline.
-
Compute Resource Optimization: Take advantage of Snowflake’s separate compute and storage architecture to dynamically allocate resources based on AI workload demands, reducing costs and improving efficiency.
Scaling Multimodal AI Initiatives with Snowflake's Cloud-Native Architecture
Accommodating Growing Data Volumes and Complexity
With the rapid expansion of AI-driven use cases, organizations must prepare for increasing data volumes and complexity. Snowflake’s elasticity and scalability enable seamless expansion while maintaining high performance.
-
Elastic Scaling: Snowflake’s architecture automatically scales to accommodate growing datasets and complex AI models without manual intervention.
-
Unified Data Storage: Snowflake consolidates structured, semi-structured, and unstructured data into a single platform, simplifying AI data pipelines.
-
High-Speed Processing: The platform’s high-performance computing ensures that AI applications run efficiently even as datasets grow exponentially.
Ensuring High Performance and Availability for Multimodal AI Applications
-
Automated Workload Management: Snowflake’s multi-cluster architecture dynamically allocates resources to maintain consistent performance across AI workloads.
-
Data Replication and Failover: Built-in replication and failover capabilities enhance system reliability and ensure uninterrupted AI operations.
-
Optimized Query Execution: AI models can benefit from Snowflake’s efficient query execution engine, reducing latency and improving inference speed.
Discover how Multimodal AI Agents are reshaping automation with real-time vision and intelligence in our full blog!
Key Benefits of Using Snowflake for Multimodal AI
Snowflake provides a scalable, secure, and high-performance environment for managing multimodal AI data. Key advantages include:
Seamless AI Integration
Built-in connectors and APIs enable smooth integration with AI platforms.
Scalability & Elasticity
Cloud-native architecture ensures effortless expansion.
Advanced Security & Governance
Industry-leading security measures protect sensitive data.
Optimized Performance
Query optimization and automated workload management enhance AI processing efficiency.
Collaborative Data Sharing
Teams can work on AI projects without duplicating datasets, fostering innovation and agility.
The Future of Multimodal AI and Snowflake’s Ongoing Role
As AI continues to evolve, Snowflake remains at the forefront of innovation, enabling businesses to extract maximum value from their data assets. With continuous enhancements in AI-driven analytics, model training, and real-time processing, Snowflake will play a critical role in shaping the next generation of AI-powered enterprises.
As businesses continue to push the boundaries of AI innovation, Snowflake’s commitment to scalability, security, and cutting-edge analytics positions it as a fundamental enabler of next-generation AI success.
Next Steps for Implementing Multimodal AI with Snowflake
Talk to our experts about implementing Multimodal AI Systems. Learn how industries and different departments leverage AI-Driven Workflows and Intelligent Decision-Making to become Data-Centric and AI-Powered. Utilize AI for Automating and Optimizing Data Processing and AI Workloads, enhancing efficiency, scalability, and responsiveness.