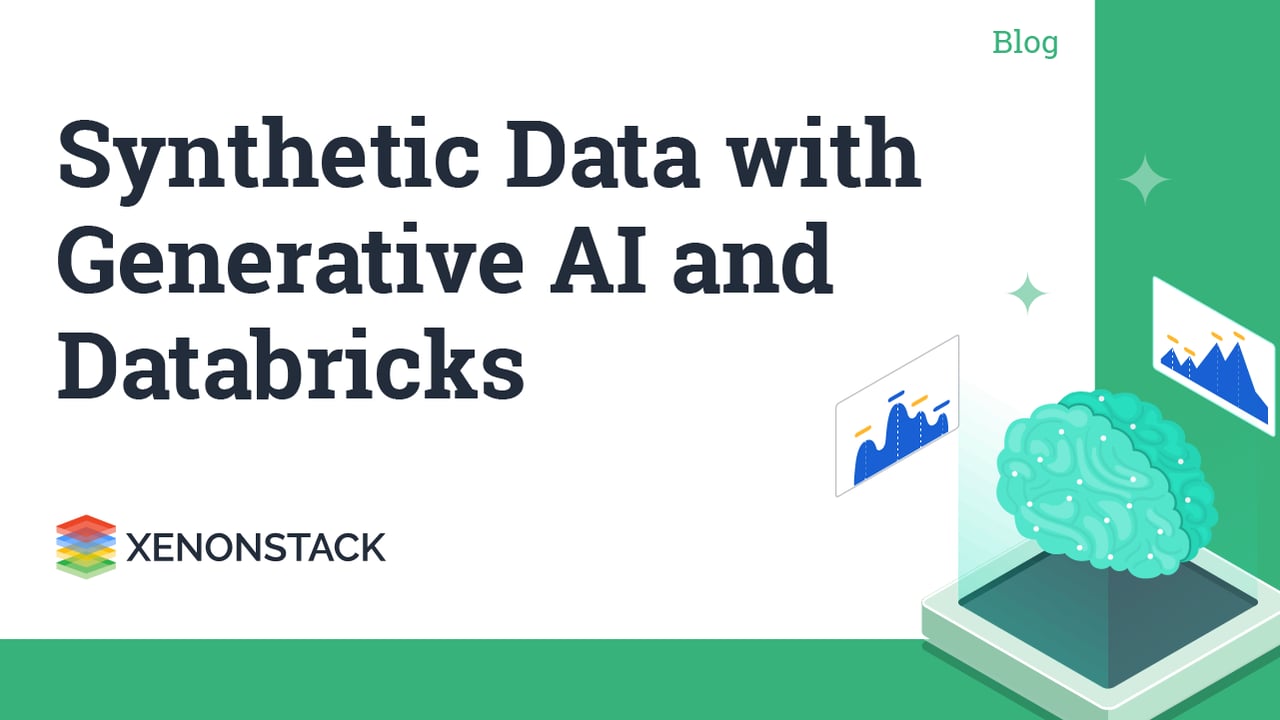
Introduction to Synthetic Data
Synthetic data has become increasingly important in today's data-driven world, providing a powerful solution for generating large and diverse datasets without relying on real-world data. Synthetic data mimics accurate data and can be used for training machine learning models, conducting research, and testing applications.
In today's data-driven world, the demand for large and diverse datasets is more significant than ever. These datasets are essential for training machine learning models, conducting research, and testing applications. However, obtaining real-world data can be challenging due to privacy concerns, data scarcity, or other limitations. This is where Generative AI and platforms like Databricks come to the rescue. Databricks enables organizations to create synthetic data that mimics real-world data for various use cases.
What is Generative AI?
Generative AI is a subfield of artificial intelligence that trains models to generate new data sets. It is commonly used to generate images and text and to generate data for data synthesis. One of the most widely used Generative Artificial Intelligence models is the GAN. A GAN is made up of two neural networks: the generator and the discriminator. In a GAN, the generator generates synthetic data, and the discriminator verifies that the generated data is real. These networks go through a training process where the generator attempts to generate data that is indistinguishable from real data.
Use Cases for Synthetic Data
Privacy Preservation: Synthetic data helps protect sensitive information, like health or financial records, by removing personal details while keeping the overall patterns of the original data.
Testing and Development: Software developers and data scientists can use synthetic data when real data is unavailable or cannot be used due to privacy laws. It allows them to test and develop applications safely.
Model Training: A large and diverse dataset is essential for training machine learning models. Synthetic data can enhance real data or create entirely new datasets for training purposes.
Research and Analysis: Synthetic data is useful for researchers who want to run experiments and simulate scenarios without relying on real-world data, making their work easier and more flexible.
Steps to Generate Synthetic Data
Databricks is an open-source analytics platform that allows data engineers, data scientists, and machine learning experts to collaborate effectively. It offers a wide range of tools and libraries for working with Generative AI and creating synthetic data. Here’s a simple guide to generating synthetic data with Databricks:
1. Data Preparation
Start by importing your real data into Databricks. Make sure to anonymize and preprocess it to remove any sensitive information.
2. Choose a Generative AI Model
Pick a Generative AI model that fits your data type. For instance, you might use Generative Adversarial Networks (GANs) for images or text-based models like OpenAI’s GPT for text data.
3. Model Training
Train your chosen Generative AI model using the preprocessed data. Databricks support GPU acceleration, which can speed up the training process.
4. Data Generation
Once the model is trained, you can use it to generate synthetic data. The quality and variety of this data will depend on how well the model was trained and the amount of data you provided.
5. Data Evaluation
Check the synthetic data against the original data using statistical measures and visualizations to ensure it retains similar characteristics.
6. Data Usage
You can now integrate synthetic data into your projects, research, or applications, keeping in mind data privacy and compliance with regulations.
Benefits of Using Databricks for Synthetic Data Generation
-
Scalability: Databricks allow you to generate large datasets, making it perfect for high-performance Generative AI models.
-
Collaboration: It provides a shared workspace, enabling data scientists and engineers to work together easily on synthetic data projects.
-
Performance: With GPU support, Databricks speeds up the training and generation of data, making the process faster.
-
Integration: The synthetic data you create can easily be used with other data processing and analysis tools available on the platform.
Conclusion
Generative AI and platforms like Databricks are becoming vital in many industries. Synthetic data is a valuable resource for protecting privacy, conducting tests, and training models. By following these steps, organizations can leverage the power of Generative AI and Databricks to create synthetic data that fits their needs while ensuring compliance with data privacy regulations. This approach helps overcome data challenges and speeds up the development of AI and machine learning applications.
-
Benefits of Log Analytics with Generative AI