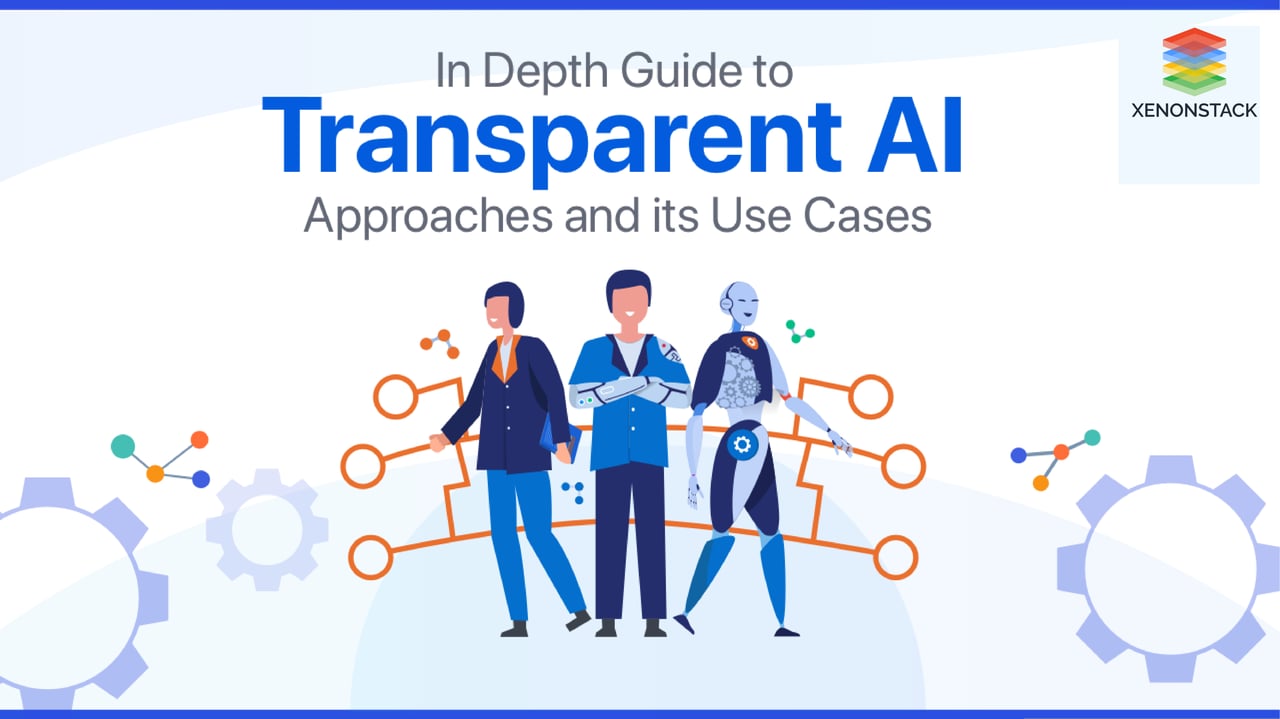
Introduction to Artificial Intelligence
Artificial Intelligence is now being used for decision-making in life and death situations. The technology has been painted both as a villain and a hero where people have taken turns deeming it as Frankenstein’s monster on some occasions and Mother Teresa on other times.
AI is popular attention, investment, and application due to the rapid development in machine learning research and implementation. ML is a key component in making AI work. Machine learning is all about giving machines a lot of data so they can learn and employ intricate algorithms. Machines may learn from their past experiences and data, and their abilities will grow with time. The flow that informs the machine how to learn is known as the machine learning algorithm. The machine learning model is the result of such learning, and it may be applied to new data. The main problem most organizations face is that due to the ambiguity of the systems, the presence of biases is unknown. The sensible response to the challenges caused by opacity is to increase the requirement for clarity.
AI Governance continues to evolve, companies may need to develop basic AI governance capabilities to get the most from their AI projects. Click to explore our, Artificial Intelligence Governance Strategy and Best Practices
What is Transparent AI?
Transparent AI is a solution to the AI black box dilemma when technology needs to explain its conclusion and how it arrived at that decision. It explains how and why AI makes such decisions. After all, how can we agree on a recommendation if we don't know how we arrived at it? We can be certain that this answer is correct and free of bias. AI transparency would help users recognize when an AI decision isn't right. It would make sure that someone was authorizing AI.
- Artificial intelligence is generally thought to be objective. AI isn't flawless either. It is capable of making errors, and it does so on occasion.
- Yes, it is desirable to have AI that is always correct and fair. If AI isn't transparent, we won't identify if a result is the consequence of an error. An AI's false judgment that you want to watch a fishing show, on the other hand, will never harm the rest of your life.
- However, necessary and critical applications use it. It has an impact on people's beliefs, medical treatment, and financial decisions. And all of a sudden, we must understand — and be able to verify — the reasoning behind an AI's output. If AI makes a mistake, it may endanger people's lives.
Why do we need Transparent AI?
The below-highlighted points are why we need Transparent AI:
Unexplained Algorithm
- The fact that some machine learning algorithms are deep is a well-known problem.
Once a decision has been made, or the model has concluded, such as classification or regression. It isn't easy to understand how we reach this solution without transparent AI.
Lack of visibility into Training Datasets
- As previously mentioned, a model's strength primarily determines its training data. In supervised learning approaches, good, tidy, and well-labeled data would result in good, well-performing models.
Lack of visibility into the method of data selection
- Assume you have access to the complete set of training data. Isn't this going to be the height of transparency for the model? That's not the case.
- What if the machine learning engineers only used a subset of that data, or just specific dimensions, columns, or characteristics of that data set?
- What if the data scientists or data analysts used data augmentation techniques to supplement the training data with data that didn't include the training set? Having access to the training data isn't enough to satisfy all transparency concerns.
Limited understanding of bias in training datasets
Models often encounter issues not due to insufficient data or even poorly chosen data but due to inherent bias in the training data. We use the term "bias" in 2 different ways:-
- In machine learning: in the sense of the weights and "biases" set in a neural network.
- Commonly understood the importance of informational "bias" imposed by humans making choices based on their preconceived notions.
The end-to-end governance process is based on Ethics, ML interpretations, and the human-centered and secure AI system. Discover more about Responsible AI in Automotive Industry
What are the challenge of Transparent AI?
For example, to identify cats images, we built a machine learning model. If the model identifies dogs as cats or fails to spot obvious cats in images, we know there’s something wrong with the model.
- There are numerous reasons for a model's poor performance. It's possible that the input data is strewn with errors or hasn't been properly cleansed.
- The model's many variables and configurations, known as hyperparameters, could be misconfigured, resulting in subpar outcomes.
- Perhaps the data scientists and machine learning engineers that trained the model chose a subset of accessible data that was biased in some way resulting in skewed model results.
This is due to the fact that AI is a complete black box before. To resolve the above problems for customers, we need to make AI transparent. It will not only find errors fast but helps to understand the actual working of the model.
What are the best Approaches for Transparent AI?
- Keep Humans in the Loop: AI models developed to work without the presence of humans. In certain instances, though, the human aspect is essential. Humans must revisit decisions to prevent prejudices and errors that often derail AI projects.
- Eliminate Biased Datasets: To create accurate, equal, and nondiscriminatory AI models, you'll need an unbiased dataset. To provide an idea for this, the bank uses AI to resume screening and credit scoring. It has also found its way through some legal systems.
- Ensure Decisions are Explainable: Explainable AI aids in the explanation of why an AI system took a particular decision. It reveals which used aspects of the deep learning model were more often than others to predict.
- Reliably Reproducing Findings: AI models should be consistent when making predictions over time, an essential requirement in research projects.
What are the essential roles of Transparent AI?
- Legal Needs: If the work makes it necessary legal and regulatory explanation, there may be no other option but to include clarity. To achieve this, a company can have to rely on more straightforward but more understandable algorithms.
- Severity: If we use AI for important life missions, the transparency of AI plays a major role. As such tasks are not dependent on AI alone, having a reasoning mechanism improves teamwork with human operators.
- Transparency: is needed if we use AI in life-saving missions. Since such tasks are unlikely to depend mainly on AI. It provides a reasoning mechanism that increases human operators' coordination. The same is true if AI affects someone's existence, such as algorithms used in work applications.
- Exposure: An organization may want to protect the AI model from unauthorized access depending on who has access.
- Data set: An organization always aims for a diverse and balanced data set, ideally from as many sources as possible, regardless of the circumstances.
Ethical issues available in the system can only be recognized if data and algorithms are fully understood. Discover about Real-Life Ethical Issues of Artificial Intelligence
Who is responsible for Transparency in AI?
When developing AI systems that directly impact society, researchers and developers should be aware of their responsibility. Governments and individuals should decide how they should handle liability issues. For example, a self-driving car harms a pedestrian, then who will be to blame?
- Hardware builder? (e.g., of the vehicle's sensors to perceive the environment)?
- Software builder that enables the car to decide on a path? Or Authorities that allow the vehicle on the road?
- The owner that personalized the car decision-making settings to meet her preferences?
- The vehicle itself because its behavior is based on its learning?
All these, and more, questions must be informing the regulations that societies put in place towards responsible use of AI systems. AI takes as part of a more extensive set of socio-technical relationships. Education plays an important role here as well, both in terms of
- Ensuring awareness regarding the potential of AI.
- Make people aware that they can influence social growth.
Use Cases of Transparent AI
The below described are the use cases of Transparent AI:
Transparent AI in Healthcare
Using transparent AI in healthcare helps in autonomous monitoring of hospital rooms, Identification of Cardiovascular Abnormalities, Detection of Fractures and Other Musculoskeletal Injuries, Supporting the Diagnosis of Neurological Diseases. There may be chances that the wrong body part is detected as fractured.
Like a human met with a serious accident and his leg is injured badly. Due to the accident, his thigh got fractured, but when we detected with our machine learning model, it shows his knee got fractured. Due to complex algorithms and AI is a complete black box, it is difficult to find fault in our model. But with the help of transparent AI, we can resolve errors and provide the best services. This also helps doctors make a faster decision in an emergency condition and provides the best treatment to their patients. This also increases patient satisfaction levels and helps hospitals to remain in the competition.
Industrial IoT
When it comes to manufacturing, automating a factory for the future to be more efficient and effective will require AI from visual inspection for defects to robotic control for assembly. With Transparent AI, you can deploy AI capabilities at a reduced cost that can also process data at a fast speed.
Autonomous Vehicles
Using Transparent AI in autonomous vehicles or driverless cars, data is immediately processed within the same device, and action is performed within milliseconds. Due to faster decisions, there may be chances that the device failed to detect pedestrians on the road and will meet with an accident. So to find a solution for this problem, it is best to build a machine model as simple as possible. The model should be able to tell its decision and also tell us we took this decision. Because in autonomous vehicles, data is immediately processed, like recognizing vehicles, traffic signs, pedestrians, roads, etc., to operate safely. Through the Transparent AI model, we will be able to achieve goals faster and accurately.
Smart Devices
Nowadays, almost everyone is familiar with face detection, face tracking Google Home, Alexa, and Apple Siri, and they all are using AI. In this, words like Wake, To-Do list, and phrases such as “Alexa” have already been trained with the help of a machine learning model and processed locally on the speaker. Whenever it hears the word “wake”, the word will be sent over the internet to the amazon Alexa voice service that helps in phrasing voice into command it understands. After processing, it will show you the desired output. But there might be cases where it will be confused between A.M and P.M until we don’t train it properly; it can give wrong and biased output. But if we make it transparent, we will be able to find errors and know why it took this decision.
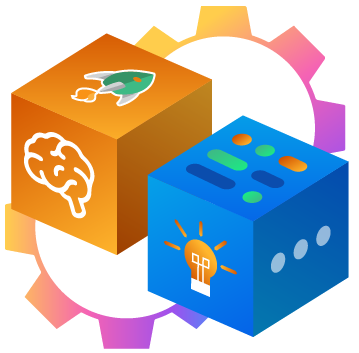
Conclusion
As discussed above, AI is present everywhere, from waking up a person using a smart device to reaching home at night through a driverless car. So to trust AI, organizations need to train models so that customers can trust easily. Sometimes it is challenging to trust third-party sources or models without having transparency into how those models operate. So to achieve long terms goals and increase customer trust, each organization needs to improve model transparency and give good clarity on what their model is going to do.
- Explore about Ethics of Artificial Intelligence
- Click to know the Role of Edge AI in Automotive Industry