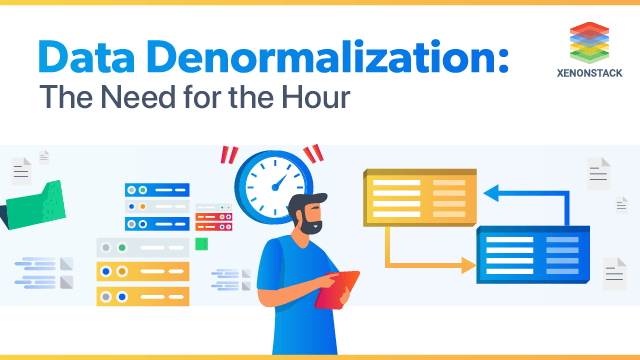
Data Denormalization for Enhanced Database Performance
Data Denormalization is a technique used on a previously-normalized database to increase performance. In computing, denormalization is the process of improving the read performance of a database, at the expense of losing some write performance, by adding redundant copies of data or by grouping it. Some of the techniques used in Data Denormalization are:
1. Pre-Joining Tables
2. Mirrored Tables
3. Table Splitting
4. Storing Derivable Values
Data is a set of characters generated for some purpose. They are individual units of information. Data can be in any form numbers, character, special symbols, sound, and video. The data means " information" and " knowledge". The data is composed of bits, bytes, and characters in computer language. It is processed by the CPU, which uses logical operations to bring new data from the input data. The data describe the quantity and quality also of some objects.
Apache Flink has Dataset API available for batch processing, and the SQL and Table API would work on batch data as well
Normalization Technique
Normalization is a technique that helps in organizing the data in the database. The main purpose of normalizing the data is to remove redundancy from the relation. Normalization divides the table into smaller tables while normalizing the table. Normalization also helps in minimizing the redundancy anomalies such as:
1. Updation anomaly
This anomaly occurs when the duplicated data is updated at one instance and not in the entire instance where the redundant data is present.
2. Deletion anomaly
This anomaly occurs when you remove the records which contain the additional important information which also gets deleted.
3. Insertion anomaly
This anomaly occurs when you cannot add certain attributes in the database without the presence of the other attributes.
Types of Normalization
Below mentioned are certain types of normalization which are commonly used normal forms in the database:1. First normal form (1NF)
2. Second normal form (2NF)
3. Third normal form (3NF)
4. Boyce and Codd normal form (BCNF)
A method/process in which we split a process into different parts and execute them simultaneously using different processors on the same system. Click to explore about our, Golang for Big Data and Parallel Processing Applications
Data Denormalization and Normalization
We all now know about the normalization of the database. It does not mean that the data is not normalized. Denormalization is a technique that is performed on the normalized data. In normalization of data, we store data in separate tables to avoid redundancy due to which we have only one copy of each data in a database. In some ways, it is a good thing to happen. If we update the data at one place, there will be no chance of the duplication of data. But if the no of tables is large, we have to spend much time performing joins on those tables. But with the help of it, we think that some duplicated data is okay and some efforts to perform fewer joins with the efficiency advantages. So that is why the denormalization is not the unnormalized data. Having redundant data can improve the performance in specific ways of database searches for a particular item.
Enhancing Data Denormalization Strategies
Data denormalization is a powerful technique used to optimize database performance, particularly in environments where query speed and efficiency are critical. By strategically reducing the complexity of database structures, organizations can achieve significant improvements in their data retrieval processes. Below are key considerations and strategies that can be integrated into your data denormalization practices.
Database Query Performance
One of the primary goals of data denormalization is to enhance database query performance. By minimizing the number of joins and simplifying relationships between tables, queries can execute more quickly. This is particularly important in high-traffic applications where response times directly impact user experience.
Redundancy Management
While denormalization often introduces some level of redundancy, it is essential to manage this carefully. The trade-off between redundancy and performance must be evaluated to ensure that the benefits of faster queries do not come at the cost of excessive data duplication, which can complicate data maintenance.
Materialized Views
Utilizing materialized views can significantly improve query performance by pre-computing and storing complex joins and aggregations. This approach allows for faster access to frequently requested data without the overhead of recalculating results on-the-fly.
Scalability Considerations
As your application grows, maintaining scalability becomes crucial. Denormalized databases can sometimes hinder scalability if not designed with future growth in mind. It’s important to regularly assess database performance and scalability metrics to ensure that your architecture can handle increased loads.
User Experience Optimization
Optimizing for user experience involves creating a seamless interaction with your applications. Fast query responses lead to higher user satisfaction. By implementing denormalization strategies that prioritize speed, organizations can enhance their overall service delivery.
Data Complexity Reduction
Reducing data complexity through denormalization simplifies the data model, making it easier for developers and analysts to work with. This simplification can lead to faster development cycles and easier maintenance.
Transaction Reduction
Denormalization often results in fewer transactions being required for complex queries, which is known as transaction reduction. This reduction not only improves performance but also decreases the load on database systems during peak usage times.
Report Generation Efficiency
For organizations relying heavily on reporting, improving report generation efficiency is vital. Denormalized structures allow for quicker access to aggregated data, enabling faster report generation and more timely insights.
Data Aggregation and Summarization
Finally, effective data aggregation and summarization techniques should be employed in conjunction with denormalization. By summarizing data at various levels, organizations can reduce the amount of information processed during queries while still providing valuable insights. Incorporating these elements into the XenonStack blog will provide readers with a comprehensive understanding of how to leverage denormalization effectively while addressing critical aspects of database management that enhance overall performance and user satisfaction.
What are the advantages of data denormalization?
Denormalization is used by database managers to increase the performance of a database. Some of its advantages are:
1. Minimizing the need for joins
2. Reducing the number of tables
3. Queries to be retrieved can be simpler.
4. Less likely to have bugs
5. Precomputing derived values
6. Reducing the number of relations
7.Reducing the number of foreign keys in relation
8.Data modification at the computing time and rather than at the select time
9. Retrieving data is faster due to fewer joins.
A technique that is used to convert the raw data into a clean data set. Click to explore about our, Data Preprocessing and Data Wrangling in Machine Learning
What are the disadvantages of data denormalization?
Although Data Denormalization can avoid some anomalies that can lead to the mismatch of the result, it may
1. Slow down updates, although maybe speeding up retrievals.
2. Make it more complex in others, although simplifying implementation.
3. Be inconsistent.
Sacrifice flexibility.
It also can
1. Increase the size of relations.
2. Make the update and insert codes harder to write.
Involve Data redundancy which necessitates more storage.
The data can be changed now in many places, so we have to be careful while adjusting the data to avoid data anomalies. We can use triggers, transactions, or procedures to avoid such inconsistencies.
Data Denormalization vs Normalization
For all the benefits that normalizing data brings, just like anything else in information technology, there are tradeoffs and costs. A normalized relational database for even a small business could comprise hundreds of tables. For transactions, like purchases, inventory maintenance, personal data, this should not present many issues if data management is being handled through a front end application. While normalized data is optimized for entity-level transactions, denormalized data is optimized for answering business questions and driving decision making. Denormalized data is data that has been extracted from the large collection of normalized tables and has been organized and/or aggregated into fewer tables without regard to such things as redundancy. It has fewer rules about structure and not like normalization. There are schematic patterns, like Snowflake Schema, but the design is usually more specific to a particular organization’s needs. Reporting and decision support is simplified through a minimum of aggregated tables versus extracting data in real-time through multiple table joins.
Next Steps towards Data Denormalization
Curious about optimizing your database for performance? Learn how data denormalization can simplify complex queries, reduce joins, and boost system speed. Connect with experts to explore the best practices and strategies tailored to your needs!