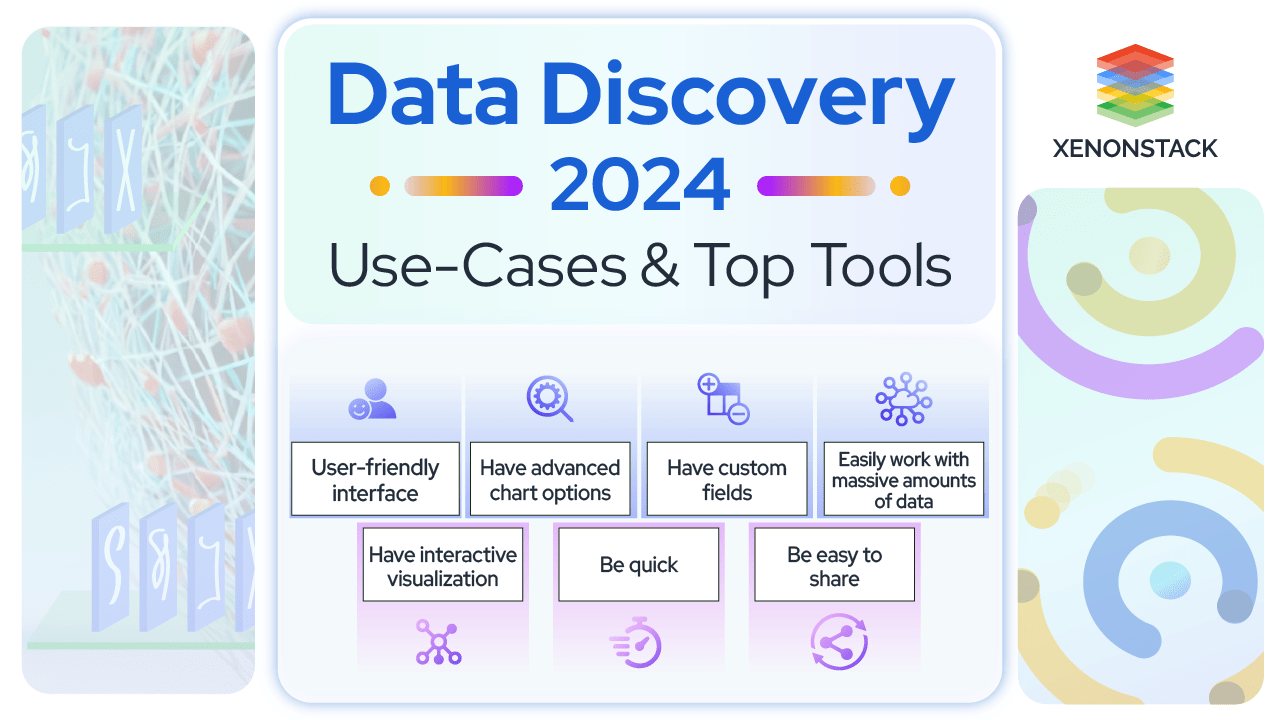
Data discovery is a user-centric data science process that enables individuals to visually navigate, analyze, and uncover insights from organizational data. By leveraging advanced analytics, this approach helps identify trends, patterns, and anomalies, addressing specific business queries and extracting actionable value. It allows businesses to take a step back from isolated data points and instead integrate information from diverse sources, including external data, to build a more comprehensive and holistic view of their operations. Similar to a golfer assessing the terrain before a putt, data discovery facilitates informed decision-making and strategic planning by providing a broader perspective.
Often embedded within business intelligence (BI) software, it presents a comprehensive organizational overview through intuitive dashboards or visualizations, making data analysis more accessible. This empowers users to explore the data landscape, uncover hidden patterns, and address emerging anomalies without the need to build complex models. With a focus on data security, data discovery promotes a deeper understanding of data, contributing to more effective decision-making and improved business outcomes.
Key benefits include:
- User Empowerment: Enables non-technical users to explore data through intuitive interfaces.
- Holistic Data View: Integrates multiple data sources for a comprehensive business perspective.
- Trends & Anomalies: Identifies patterns and outliers to inform decisions.
- Informed Decisions: Supports strategic, data-driven decision-making.
- Actionable Insights: Extract relevant insights to address business needs.
What is the significance of data discovery?
In today’s fast-paced business environment, where data proliferation is a constant, data discovery plays an essential role in unlocking the true potential of the data available. Businesses are inundated with data from diverse sources, including third-party analytics, social media, and internal reports. However, simply accumulating data is not enough; the key lies in the intelligent extraction of hidden patterns and insights. Much like how individual ingredients don't form pancakes until they are properly mixed and cooked, siloed data sources remain disconnected and lack meaningful value without undergoing the data discovery process.
Data discovery is instrumental in transforming unstructured data into actionable intelligence, empowering businesses to make smarter, more agile, and cost-effective decisions. In a competitive advantage landscape, these insights can significantly impact operations, driving cost savings, increasing revenue, discovering new markets, and optimizing business strategies.
Moreover, data discovery provides businesses with the tools necessary to understand, monitor, and manage their data comprehensively. It strengthens data governance, allowing organizations to maintain full visibility over data ownership, storage, access, and transmission. Continuous real-time monitoring of data activities helps mitigate vulnerabilities, ensuring data security and compliance with regulatory standards. The process also aids in data classification, enabling the identification of sensitive data, its context, and the ability to clean, reuse, and scale it across various business functions. This comprehensive approach enhances risk management, regulatory compliance, and the ability to make data-driven decisions at scale.
How does Data Discovery work?
Data discovery is a vital process that enables organizations to uncover valuable insights from their data by identifying, gathering, and analyzing information across multiple sources. It plays a key role in ensuring compliance by helping organizations track sensitive data, manage access, and adhere to privacy regulations like GDPR and HIPAA.
By integrating data from various systems and formats, data discovery facilitates data integration, breaking down silos and providing a unified view of business operations. This integration, combined with powerful visualization tools, allows businesses to easily interpret complex datasets through charts, graphs, and dashboards, enabling them to spot trends and correlations. Furthermore, data discovery lays the groundwork for predictive analytics, allowing organizations to leverage historical data to forecast future outcomes and make informed decisions. Ultimately, this process drives innovation by revealing hidden opportunities and patterns, helping businesses stay competitive and agile in a data-driven world.
1. Iterative Nature of Data Discovery
Data discovery is an iterative process, requiring businesses to continuously repeat the steps to gather, analyze, and distill results over time. This iterative approach ensures ongoing refinement and accuracy of insights.
2. Role of Data Product Manager
The emergence of the Data Product Manager role is integral to the data discovery process, emphasizing the ownership of data. A clear tracking plan/catalog is essential for effective data discovery, aligning with the responsibilities of a Data Product Manager.
3. Purpose-Driven Approach
The first step in data discovery is to clearly define the purpose, aligning with business goals and industry needs. This purpose guides the entire process, helping businesses identify relevant data sources and streamline their approach for more meaningful insights.
The Stages of Data Discovery
Data discovery involves a structured process with six key steps:
1. Ask Right Questions
Business Analysts work with SME to gain Domain Understanding and Then Ask Questions from Data and Define the Problem.
2. Acquire Data
Once business analysts understand different datasets, they will work on collecting data from various data sources with the help of the data engineering team.
3. Data Curation
Business Analysts defined the Data Cleaning Rules and Data Engineering loads the Data in Data Lakehouse so that Business Analysts can start analyzing the cleaner data.
4. Visualize & Analyse
Business Analyst connects their Data Visualization Tools to visualize different Datasets & identify different patterns, trends, and correlations between different Data Sets .
5. Publish Results
Once Business Analysts finish their Analysis, then They publish their Analysis Report and share it with all Stakeholders.
6. Identify Actions
After going through the Report , Stakeholders decide next Actions to improve their Business Processes and Customer Experience.
What are the Top Use Cases of Data Discovery?
The best use cases are listed below:
1. Data Consolidation
Locates and classifies all Personally Identifiable Information (PII) across repositories and file shares, applying business context without manual intervention which helps in facilitating efficient M&A activities or data minimization initiatives by automatically identifying sensitive data repositories, minimizing data footprint, and consolidating disparate datasets for seamless analytics and comprehensive data lineage reporting.
2. Holistic Data Discovery
Discover and automatically classify all PII data, including structured, semi-structured, and unstructured formats which accelerates the identification and tagging of PII data across diverse formats, ensuring an intelligent discovery engine locates and tags data in minutes rather than weeks or months.
3. Fraud Prevention
Utilizing advanced analytics to identify and prevent fraudulent activities across multiple sectors which employs algorithms to analyze vast datasets for patterns indicative of fraudulent behavior, enabling preemptive action to mitigate financial or reputational damage across industries.
4. Compliance Monitoring
Regulatory bodies, such as FINRA, require companies to adhere to specific compliance standards, especially concerning employees handling confidential customer data which helps in detecting potential violations or misuse of customer information through continuous monitoring, ensuring adherence to regulations and mitigating risks of fines or loss of business.
5. Insider Threat Detection
Network-based approach to discovery and mapping of "unknown unknown" sensitive data sets which enables fully autonomous data discovery without targeted scans, providing comprehensive visibility into sensitive data without limitations.
6. Third-Party Risk
Identify vendor exposure and unapproved sharing of sensitive data with third parties which monitors shared data with third-party vendors in real time, automatically detecting and notifying when sensitive data is shared, ensuring compliance with data policy rules.
7. Interactive Data Discovery for Customer Churn in Marketing
Utilizing interactive data discovery tools to delve into vast amounts of historical data across any industry, identifying key trends, relationships, and factors that drive business outcomes which facilitates a deeper understanding of data-driven insights, enabling businesses to make informed decisions swiftly. When combined with predictive analytics, it supports proactive strategies for improving customer engagement, operational efficiency, or product development.
8. Better Customer Modeling Reduces Risk in Financial Services
Risk analysts use data discovery to understand customers and predict mortgage defaults which provides precise insights by analyzing data from multiple sources, allowing for more complete customer profiles and accurate risk assessment. Adheres to data governance policies to ensure reliability and protect personally identifiable information.
9. Self-Service Analytics in Engineering for Proactive Maintenance
Leveraging self-service analytics platforms for predictive maintenance and operational efficiency across various sectors like manufacturing, utilities, and transport which empowers professionals to autonomously analyze and interpret operational data, identify potential issues before they escalate, and optimize processes for efficiency. Facilitates the creation of predictive models for maintenance, reducing downtime and extending asset lifespan. Enhances scalability and rapid response capabilities, crucial for maintaining high operational standards.
Why is data discovery creating so much buzz?
Data discovery is creating significant buzz due to several compelling reasons:
1. Unprecedented Data Growth
The modern business landscape is witnessing an unprecedented growth in data creation, acquisition, and utilization. The sheer volume and variety of data sources, including third-party analytics, internal reports, and global trends, have fueled the need for effective data discovery.
2. Informed Decision-Making
Data discovery empowers organizations to move beyond merely possessing data to intelligently extracting patterns, themes, and trends within it. This process enables businesses to make informed, data-driven decisions, leading to enhanced operational efficiency and strategic planning.
3. Iterative and Agile Approach
The iterative nature of data discovery allows businesses to continuously refine and improve their insights over time. This agile approach ensures that organizations can adapt to evolving data landscapes and extract maximum value from their data assets.
4. Business Intelligence Integration
Integration with Business Intelligence (BI) software enhances the accessibility of data discovery tools. The seamless incorporation of data discovery into BI platforms provides users with intuitive dashboards, fostering broader utilization across different functions within an organization.
5. Role in Digital Transformation
Data discovery plays a pivotal role in digital transformation initiatives. As businesses strive to become more data-centric, the ability to uncover hidden insights becomes crucial for gaining a competitive edge, reducing costs, and identifying new market opportunities.
6. Importance of Data Ownership
The emergence of roles like Data Product Manager highlights the importance of data ownership in the data discovery process. Clear tracking plans and catalogs are essential for successful data discovery, contributing to the rising significance of these roles in organizations.
7. Holistic Understanding of Data
Businesses recognize the need for a holistic understanding of their data landscape, encompassing aspects such as data storage, access, transmission, and continuous monitoring. Data discovery provides the tools and knowledge to achieve this comprehensive understanding.
8. Benefits Across Multiple Business Processes
The benefits of data discovery extend across various business functions, including risk management, regulatory compliance, data visibility, classification, and the identification and tracking of sensitive data. This broad impact contributes to the widespread adoption and excitement surrounding data discovery initiatives.
In summary, the buzz around data discovery stems from its ability to address contemporary challenges in handling vast and diverse datasets, facilitating better decision-making, and supporting organizations in their journey towards digital transformation.
Leveraging Generative AI for Data Discovery
The advent of Generative AI is reshaping the landscape of data discovery, providing businesses with the tools to navigate the vast seas of data more efficiently and uncover insights that were previously beyond reach. This transformative technology is not just enhancing data analysis capabilities but is revolutionizing the way enterprises leverage data for decision-making, innovation, and strategic advantage.
Gen AI Transforming Enterprise Data Analytics
Generative AI, with its capability to generate, simulate, and predict data, is radically changing enterprise data analytics. Platforms like SparkBeyond's Discovery leverage Generative AI to automate the generation and testing of hypotheses at an unprecedented scale, bypassing human cognitive limits and biases. This not only accelerates the process of data exploration but also opens new avenues for discovering nuanced insights and relationships within data.
Gen AI Enhancing Data Management Across the Board
Generative AI's impact extends across all facets of data management, from integration and transformation to discovery and quality assurance. It aids in inferring data schemas, parsing unstructured data, and automating data cleansing and transformation processes. Moreover, it enhances data discovery through advanced profiling, clustering, and visualization techniques, making it easier for businesses to identify patterns, trends, and outliers.
Gen AI powered Data Discovery using Dremio
Dremio
Dremio has taken a significant leap forward in the realm of data analytics with its introduction of Generative AI-powered data discovery features, announced at AWS re:Invent. This innovative approach not only accelerates the process of data contextualization and description for analytics but also simplifies it, marking a notable advancement in how data is handled and analyzed.
Key Features and Capabilities:
- AI-Powered Data Discovery: Dremio now incorporates AI to streamline the discovery, contextualization, and description of data, making it significantly easier for users to navigate and understand their datasets.
- GenAI-Powered Documentation and Labeling: Expanding on its text-to-SQL capabilities, Dremio introduces features that automate data documentation and labeling. This not only provides comprehensive business context for analytics but also significantly reduces the manual labor involved in these processes.
- Enhanced Analytics Engine for Apache Iceberg: Alongside its GenAI capabilities, Dremio continues to improve its performance as an analytics engine, offering superior functionality for Apache Iceberg, further solidifying its position as a leader in the analytics space.
What is Governed Data Discovery?
Governed Data Discovery refers to a structured and controlled approach to exploring and analyzing data within an organization. It combines the flexibility of self-service analytics with established governance policies and controls. In this framework, users are empowered to discover insights independently, but within predefined boundaries set by data governance rules. Governed Data Discovery ensures compliance with regulatory requirements, maintains data integrity, and establishes clear guidelines for data access and usage. This approach strikes a balance between fostering data exploration and mitigating risks associated with unauthorized or unregulated data practices, promoting responsible and secure data-driven decision-making within the organization.
What are the best data discovery tools for 2024?
An increasing number of data discovery tools are available, generally included as part of a business intelligence solution, and cover at least one of the three main data discovery categories: data preparation, visual analysis, and guided advanced analytics.
1. JMP Statistical Discovery:
- Empowers organizations to simplify data analysis and decision-making.
- Notable for its ad hoc analysis capabilities and user-driven customization of dashboards.
2. Looker:
- A Business Intelligence (BI) platform that transforms raw data into dashboards, reports, and graphs.
- Offers holistic views of corporate performance with configurable access to key performance indicators (KPIs) and metrics.
3. Microsoft Power BI:
-
Comprehensive suite for BI, reporting, and data visualization.
-
Includes Power BI Service, Power BI Desktop, Power BI Pro, Power BI Premium, Power BI Embedded, Power Bi Mobile, and Power BI Report Server.
4. Phocas:
-
Business intelligence software facilitates data aggregation and transformation.
-
Simplifies data management with powerful analytics and visualization tools.
5. Qlik Sense:
-
Enterprise-grade analytical solution for data discovery, visualization, and predictive analytics.
-
Provides interactive analytical apps and customizable visualizations.
6.Spirion:
-
Specializes in discovering and classifying sensitive data across various formats.
-
Aids in network-based discovery of "unknown unknowns" sensitive data sets.
7. Tableau:
-
Leading BI solution offering data discovery, analysis, and visualization tools.
-
Enables users to create interactive dashboards for comprehensive data exploration.
8. TIBCO Spotfire:
-
Enterprise-grade analytical solution with data discovery, visualization, and predictive analytics.
-
Accelerates data analysis, providing AI-driven insights for informed decision-making.
9. Atlan:
-
Facilitates data discovery and collaboration for teams.
-
Focuses on simplifying data workflows and ensuring data accessibility.
10. Domo:
-
BI software-as-a-service platform for aggregating and transforming raw data into dashboards.
-
Offers configurable access to a variety of KPIs and metrics for real-time data insights.
Each tool brings unique features and capabilities, catering to diverse business intelligence needs.
What are the Attributes of Data Discovery Tools?
1. User-Friendly Interface
Modern data discovery tools should prioritize a user-friendly interface, moving beyond the realm of professional analysts or IT experts. Democratization of data allows individuals across various roles to leverage information and tools effectively.
2. Efficiency and Speed
In a competitive landscape, the efficiency of data discovery tools is crucial. The chosen tools must operate swiftly, ensuring that businesses can gain a competitive edge in a fast-paced industry.
3. Handling Large Data Sets
As data volumes continue to grow, the ability of a tool to efficiently work with large amounts of data is paramount. Look for tools that facilitate data integration from diverse sources, accommodating varying data sizes seamlessly.
4. Interactive Visualizations
A robust data discovery tool should provide powerful and interactive data visualizations. This includes a dashboard creator with a variety of predefined templates, fostering a comprehensive understanding of key metrics at a glance.
5. Advanced Graph Options
Beyond basic visualizations, effective software must offer advanced chart options for in-depth analysis. Features like combining multiple chart types, creating secondary axes, utilizing trend indicators, conditional formatting, and enabling comparison periods enhance analytical capabilities.
6. Custom Fields Functionality
Professional data discovery tools should incorporate custom fields, allowing users to add calculated fields and merge multiple category aspects. This feature, often implemented through drag-and-drop functions, enhances the flexibility and depth of data analysis.
7. Ease of Sharing
Collaboration is a cornerstone of effective data management. An ideal data discovery tool facilitates easy sharing, enabling stakeholders across departments to comprehend data patterns. The tool should provide different access levels based on roles and data requirements, promoting seamless collaboration.
Explore further about Augmented Data Management Solutions
How to Perform Smart Data Discovery?
Different ways for performing Smart Data discovery are mentioned below:
1. Define your business goals
The first step in performing intelligent data discovery is defining your business goals to ensure that your process. Smart Data discoveries is focused on accurate information. You can start a conversation with your key stakeholders and team members to define the primary strategic goals for the business and the metrics you will need to track the progress and measure the success.
2. Determine your problem areas
After defining your goals, it's time to recognize your problem areas or the roadblocks keeping you from becoming a more competent, intelligent business entity. While every organization is unique, and one size does not fit all, several problem areas frequently cross over from one to the next.
3. Combine various data sources to gain a deeper insight
Collecting data from various sources allows you to gain insights to propel your business forward. Data comes from various structured and unstructured sources. Integrating your data with the data of others will provide you with a greater understanding of what you need to do to accomplish your goals.
4. Clean the data extracted
Before visualizing your data, please clean it up and extract insights from it. It will also make it easier for BI reporting tools to interact with your data and perform intelligent data discovery. The ultimate goal of the data cleaning stage is to avoid the risks of working with inaccurate data, which can harm your business.
5. Choose the right data discovery tool
What precisely is an intelligent data discovery model? Fundamentally, this type of model is a strategic approach to data use. Entity-relationship diagrams, data mapping specifications, data matrices, and data flow diagrams are examples of discovery models in terms of approach. Selecting the perfect report tools to do the job is also an important consideration.
6. Automate the process
With such a large amount of data, preparing it can be time-consuming. As a result, BI solutions with automation technologies offer the benefits of more advanced data classification methods such as catalog-based search or patterns search. Another significant advantage of data discovery automation is real-time decision-making.
7. Make the data available
When we speak of ease of access, we mean using user-friendly tools that anyone in the organization can use without any tech expertise. The marketing team, for example, should be able to easily access IT metrics to support their e-commerce strategies. You will lay the foundation for the much-desired data-driven culture and empower everyone else in your organization to play an active role.
How does Data Discovery Give Security?
Data discovery enhances security by providing comprehensive visibility into sensitive information, ensuring swift identification and classification of data. Through tools like Spirion and Datapine, organizations can locate, tag, and monitor personal or confidential data, reducing the risk of unauthorized access or data breaches. Governing data discovery with solutions such as Dundas BI and Logikcull ensures adherence to compliance standards, protecting against regulatory violations. The ability to discover unknown data sets with solutions like Tibco Spotfire further fortifies security measures, enabling proactive risk management. In essence, data discovery acts as a powerful safeguard, enabling organizations to proactively manage and protect their valuable data assets.
Discover the role of Master Data Management in supply chain efficiency
Next Steps towards Data Discovery
The next steps towards data discovery involve identifying key data sources, cleaning and organizing datasets, applying advanced analytics techniques, and leveraging modern tools to extract actionable insights. Collaboration across teams is essential to unlock the full potential of data.