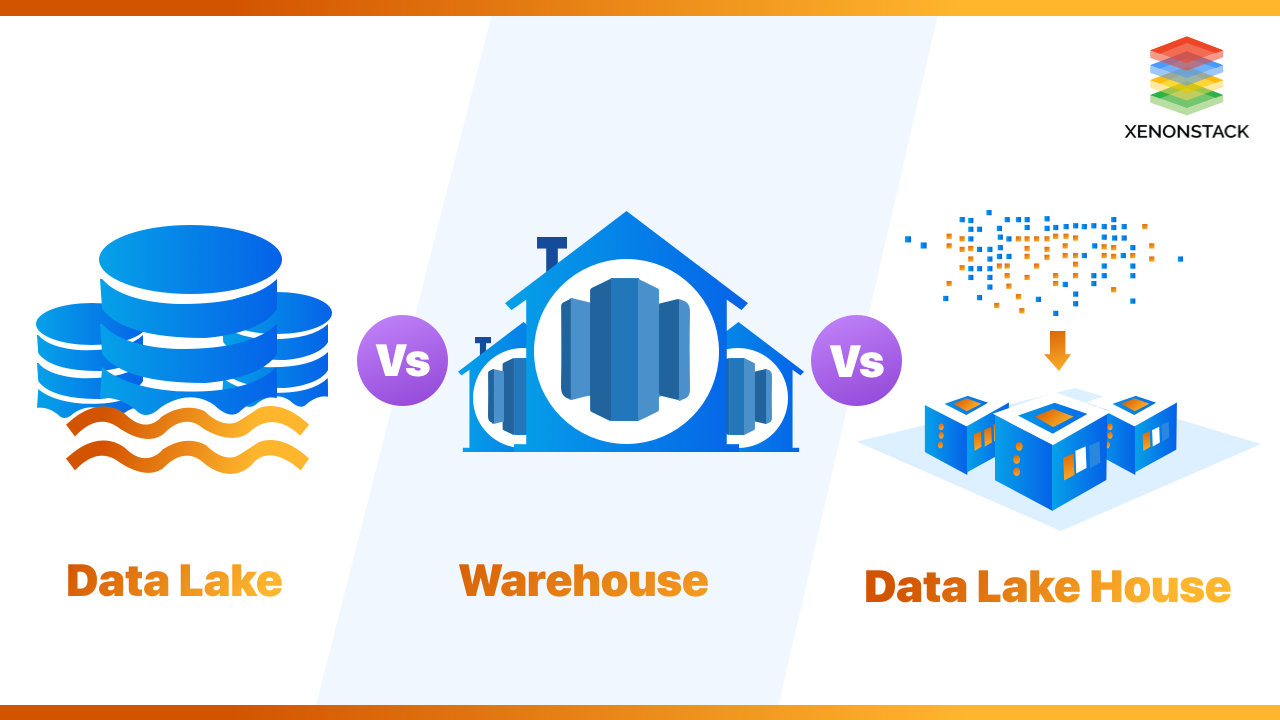
Introduction
In the ever-shifting era of technologies where each day a new term emerges and evolves, data being generated is also increasing, and businesses are investing in technologies to capture data and capitalize on it as fast as possible. But a question arises what benefits does real-time data bring if it takes an eternity to use it. The quandary the stack faces is at roots on what to use data warehouse or lake.
While warehouse is inefficient to store your streaming information, using a data lake is also less compelling as you can’t query the model and data while it is fresh enough. What cloud architecture do we opt for? Shall we settle with the limitations of the warehouse, or we accept the lake, or should we ponder over newer concepts data lakehouse?
What is Data Warehouse?
Structured data is integrated into the traditional enterprise warehouse from external sources using ETLs. Enterprise warehouses were built for BI and reporting purposes. But with the increase in demand to ingest more data, of different types, from various sources, with different velocities, the traditional data warehouses have fallen short.
Store and Transform your Data into Modern Warehouse with Xenonstack
Remember the time when changing the operating system required formatting hard drives. If you ever wanted to use a different operating system, you would need a separate hard drive explicitly formatted for the operating system, as with warehouses. The warehouse link you with a single vendor to process your data either because your storage and analytics are lumped together, or processing requires data in a specific format only, on the contrary, it makes the information availability rapid, valuable, organized, and pretty straightforward, thus empowering business intelligence and reporting.
What are the Pros and Cons?
Pros | Cons |
Easy data discovery and query | Cannot leverage other vendor capabilities |
Straight forward data preparation with clean data | Not a very cost-effective way to store and analyze unstructured or streaming data. |
What is Data Lake?
It enable all kinds of data. It helps to store information at one location in an open format that is ready to be read. For example, you could integrate semi-structured click stream data on the fly and provide real-time insights without incorporating that data into a relational database structure. The lake offers great potential, but on the other, we need to be wary about the amount of data we put in and avoid situations like swamps.
It also brings us to one of its major issue: the ingested open formatted data still needs to be queried and prepared. The analytics team often waits before the complex pipeline has been set to drive value out of the data. In addition, any issue would require the engineers to tweak the code to get the desired result, which makes the process cumbersome.
What are the Pros and Cons?
Pros | Cons |
Can handle both structured and semi-structured data.
|
Take time for data to be queryable.
|
Optimum for streaming and complex data processing.
|
Requires building complex pipeline.
|
Cost-effective solutions for any data type.
|
Takes time to ensure data quality and reliability.
|
What is the difference between Data Warehouse and Data Lake?
Data in your Warehouse is rigid and normalized. It is well structured, making it easily readable, whereas data in the Lake is raw, loosely bounded, and decoupled. Hence, while moving from warehouse to it, we lose rigidity and atomicity (no partial success), Consistency, Isolation, Durability.
- Warehouse tends towards schema-on-write whereas it tends towards on schema-on-read
- Itcan store both structured and unstructured data, whereas structure is required for a warehouse.
- The data warehouse is tightly coupled, whereas Lakes have decoupled compute and storage.
- Lakes are easy to change and scale in comparison with a warehouse.
- Data retention in the warehouse is less due to storage expense.
What is Data Lakehouse?
It attempts to satisfy the desire to bring in the best of both data warehouse and lake, alluding to giving reliability and structure present in it with scalability and agility. A lakehouse provides a one-size-fits-all approach. It is not merely an integration of a warehouse with a data lake but a combination of it, warehouse, and purpose-built store enabling easy, unified governance and movement.
A Lakehouse is a new, open system design architecture that combines the agility, cost-efficiency, and scale of it with warehouses' data management and ACID transactions, enabling BI and ML on all enterprise data.
What are the Pros and Cons?
Pros | Cons |
Atomicity, Consistency, Isolation, Durability remain intact | Relatively new and is far away to stand as a mature storage system |
BI tools can be empowered hence critical decision making is possible | Need out of a box approach or else is costly to maintain |
All data resides in one platform also implying fewer hostname to maintain | It May take time to setup |
Data duplicity gets reduced | No one for all tool is yet present to utilize full potential |
Doesn’t binds to a single platform and can leverage different tech | |
Cost-effective | |
Easy to maintain and problem fixing takes less time | |
Make it easier to build a pipeline |
How does it works?
The lakehouse has dual layered architecture in which a warehouse layer resides over a lake enforcing schema on write and providing quality and control, thus empowering the BI and reporting. It is a hybrid approach and proved an amalgamation between structured and unstructured data.
What are the use cases of Data Lakehouse?
- Analysis of Clickstream Data - as the data collected from the web can be integrated into it, some of the data could be stored in the warehouse for daily reported while others for analysis.
- Creating a Larger Dataset - by copying data from sales of product from warehouses to lakes to provide the best product recommendation
- Other Situations - for moving data from purpose-built store to another for more effortless movement taking into account the data gravity
Difference between Data Lake, Data Warehouse and Data Lakehouse?
The Lakehouse is an upgraded version of it that taps its advantages, such as openness and cost-effectiveness, while mitigating its weaknesses. It increases the reliability and structure of the data lake by infusing the best warehouse.
Parameters | Data Lake | Data Warehouse | Data Lake house |
Purpose of Data | For ML and AI workloads ( Purpose of the data is not yet determined) | For Analytics or Business Intelligence ( The data is currently in use) | Can be used for ML/AI workload and Analytics/BI needs |
Type of Data | Unstructured | Structured | Unstructured and Structured |
Users | Data scientists and engineers | Business professionals | Business professionals and data teams |
Data Quality | Raw Data, Low Quality and Not Reliable | Highly curated data, reliable | Raw and curated data, high quality with in-built data governance |
ACID Compliance | Non-ACID compliance: updates and deletes are complex operations | ACID-compliant : guarantee the highest levels of integrity | ACID-compliant to ensure consistency as many sources concurrently read/write data |
Storage | Cost-effective, rapid and flexible | Costly and time-consuming | Cost-effective, rapid and flexible |
Schema | Schema on read | Schema on write | Schema enforcement |
Conclusion
To conclude, selecting the right solution of the stack will always depend on how you want to access your data while taking into consideration the velocity of the data and the gravity of data, and other factors like scalability and flexibility of your solution, The amount of effort you want to commit the future scope of your data and the actual value you want to drive through.
- Discover more about Data Lake Services for Real-Time Analytics
- Click to explore about Data Warehouse vs Data Lake vs Data Mesh