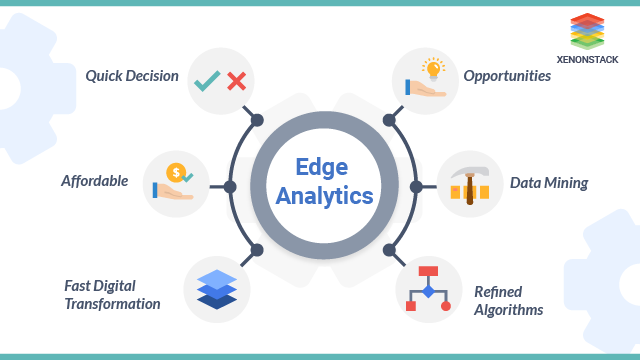
What is Edge Analytics?
Are you wondering about Edge Analytics benefits, tools, or what is it exactly? If so, then let us tell you that it is collecting and analyzing the data at the sensor, device point itself. It is not like waiting for the data sending back to the cloud or on-premise server. In simple terms, we can say that performing the analysis at the point of generating data. The main focus of IoT and data science is speed and decentralization.
Basically, it is the attempt to collect data in decentralized environments, which is a great benefit as well. Its paradigm becomes possible because the internet and web connectivity has improved. Using Edge Computing and analytics has evolved because of the need for fast response times and quick data analytics IoT networks impose. Instead, it in IoT optimizes the process by handling the bulk of analysis on-site, usually in the nearby connected network like switch or device. Then, it transmits only the most critical data back to a central server.
Nowadays, gathering the high volume of information through industries isn’t easy to manage and collect data. Click to explore about our, IoT Edge Analytics and Latest Trends
Its solutions help organizations where data insights are required at the edge, Retail, Manufacturing, Energy, Smart Cities, Transportation, and logistics vertical segments are leading the way in deploying it. There are many use cases of it in IoT like retail customer behavior analysis, remote monitoring and maintenance. For energy operations, fraud detection at financial locations (ATMs and others), and tracking of manufacturing & logistics equipment.
Why is it important?
The following points illustrate the importance of edge analytics:
Saves Time
In centralized systems, all the collected data at internet-connected devices is sent in their raw state for the processing, which is inherently slow. Whether this raw data is relevant or uncleared, processed, and analyzed to extract any value it contains. Now, what happens is that most of the deployed data is useless, and some of the information is inaccurate. In contrast, in a centralized system, all data is given equal status until an analysis is complete. The main aim of the using Edge Analytics system is to filter out the unnecessary information before analysis, and only the relevant data runs through higher-order systems. All of this results in saving both processing and uploading time, which makes the complicated analytical stage performed on the cloud much more efficient and is a great benefit.
Reduce Expenses
Edge analytics in IoT curtail the cost of data storage and management. It also reduces the operational costs, minimizes needed bandwidth, and reduces resources spent on data analysis. All these factors combine to provide significant financial savings. In the majority of industrial IoT, the data is never even analyzed, which results in wasting much information and lost improvements. Though the volume of data starts inhibiting analysis, then using this technology gives a cost-effective way to extract as much use from the data as possible, for the benefit of the business.
Preserves Privacy
It helps to preserve privacy as when sensitive or confidential information is captured by a device (such as GPS data or video streams). This sensitive information is preprocessed on-site and does not get uploaded to the cloud for processing. This extra step means that only privacy-compliant data “leaves” the device for further analysis, and it goes through an anonymizing aggregation in preprocessing. This sensitive content is preserved without losing out on the benefits complex cloud-based analysis can offer.
Reduced Latency of Data Analysis
Using it is more effective to analyze data on the faulty equipment and immediately shut it up rather than waiting for sending data to a central data analytics environment.
Connectivity Issues
It in IoT helps to protect against potential connectivity outages by ensuring that the applications are not disrupted by limited or intermittent network connectivity. It comes useful in remote locations or reducing connectivity costs while using expensive technologies like cellular.
Reduced Bandwidth Usage
Work on the backend servers gets reduced, and analytics capabilities are delivered to remote locations switching from raw transmission to metadata.
Intelligent enterprise Edge Analytics services, specially designed to help clients address the limitations of their edge operations. Check out our Intelligent Enterprise Automation Services
When should edge analytics be considered?
In IoT, it is used for Oil Rigs, Mines, and Factories which operate in low bandwidth, low latency, and richer forms of data such as video analytics.
For example, One car carries 150-300 sensors. The current airbus model has nearly 6,000 sensors and generates 2.5 Tb of data daily.
In wind turbine farms, the nearest IoT sensors generate a large amount of data daily. They also use edge computing and its monitors or detect data outliers, detect trends over time, and analyze and archive different aggregations.
An XML-based predictive model interchanges format but predictive Model Markup Language ( PMML ) provides a way to describe and exchange the analytic applications produced by data mining and machine learning algorithms.
In peer-to-peer nodes, communication may be a real possibility over time: IoT sensors are currently deployed through silos. In Edge network offers peer-to-peer communication by the Edge devices if sufficient processing capability.
The most significant promise of it may be its ability to offer energy providers insights about assets at a given time, thereby enhancing the ability to perform predictive maintenance. Maintaining and buying equipment is a significant investment for any company. Repairing or replacing energy-producing assets malfunction can exponentially increase operating expenditures.
In Edge computing, apart from intelligent sensors and connected IIoT devices to collect data, it needs hardware and software platforms to store data, prepare and train the data by the algorithms, and process the algorithms.
The descriptive analytics segment holds the largest market share, owing to the streaming need for descriptive data to enhance predictive analytical models and identify new edge analytics deployment across numerous places ( construction, industrial, and public places ) that are looking forward to driving the market for it.
The predictive analytics segment is to grow at the highest rate during the forecast period due to the high demand for preventive measures to be taken to avoid dangerous and harmful situations, which affects the growth of the industry.
How to deliver it?
It includes creating the analytics model, deploying the model, and executing the model at the edge. In each of these areas, some decisions need to be taken regarding the collection of data. For example: preparing data, selecting the algorithms, training the algorithms continuously, deploying/redeploying the models, and much more. The processing or storage capacity at the edge also plays an important role. Some of the merging deployment models consist of decentralized and peer-to-peer deployment models with pros and cons for each.
How is it different than regular analytics?
It have similar capabilities as regular analytics, except for the various situations where the analysis is performed. The main difference is that its applications need to work on edge devices with more memory, processing power, or communication impediment.
A technology-driven approach that uses Internet-connected machinery to observe the production process. Click to explore about our, IoT Solutions for Smart Manufacturing
What are its benefits?
It has received more attention with the Internet of Things (IoT) spread. For all businesses, streaming data from disparate IoT sources creates a massive amount of data which tough to manage. The analytics algorithm filters all data created at the network's edge and parameters to decide whether it is worth migrating to the cloud or data store.
Analyzing data generated decreases latency in the decision-making process as better. If an IoT component of a system suffers a failure, the algorithm interprets that data and automatically shuts it down. This saves lots of time transporting data to a centralized store, reducing or avoiding equipment downtime.
Its algorithms are applied to sensors and devices that relieve the hassle of management and analytics systems, anyway. The number of connected devices and the network size allow the system to enhance quickly and easily, no matter how much the data grows.
Limitations of Edge Analytics
It is a relatively new and trending technology. Not all hardware is capable of storing data or performing complex processing.
Businesses also consider whether or not it makes sense to invest in it, best suited for scenarios that need to minimize speed, security, or efficiency. With new technology and architectures, some engineering obstacles remain to deploying an edge application successfully.
End-to-End Solutions for empowering real-time monitoring and processing of data. Click to explore about our, Digital Twin Consulting Services
What are the best Edge analytics tools?
Some of its best tools are:
- AWS IoT Greengrass
- Cisco SmartAdvisor
- Dell Statistica
- Microsoft Azure IoT Edge
- Inter IoT Developer Kit
- Oracle Edge Analytics (OEA)
- IBM Watson IoT Edge Analytics
What are its latest Use cases?
The latest use cases of Edge analytics are defined below:
Retail customer behavior analysis
Retailers can collect data from various sensors, including car parking lot sensors, shopping cart tags, multiple store cameras, and CCTV. By applying analytics to the collection from these types of devices, retailers can offer personalized solutions with the help of behavioral targeting analytics.
Remote monitoring and maintenance for various industries
Today's industries, such as energy and manufacturing, need instant response when machines fail to work or require maintenance. Without centralized data, analytics organizations can identify the failure faster and take action before it can arise within the system.
Smart Surveillance
Businesses can benefit from real-time intruder detection edge services for security. From security cameras, by collecting raw images, we can detect and track any auspicious activity through it.
Conclusion
As far as Edge Analytics is an exciting area. Every organization in the Industrial Of Things (IIOT) is increasing yearly investments. Many famous companies are aggressively investing in this technology in specific segments such as retail, manufacturing, energy, and logistics that deliver it quantifiable business benefits by reducing the latency of decisions, solving the bandwidth problem, and potentially reducing expenses.
- Click to explore about Edge Computing vs Cloud Computing
- Discover here about IoT Strategy and Consulting Solutions