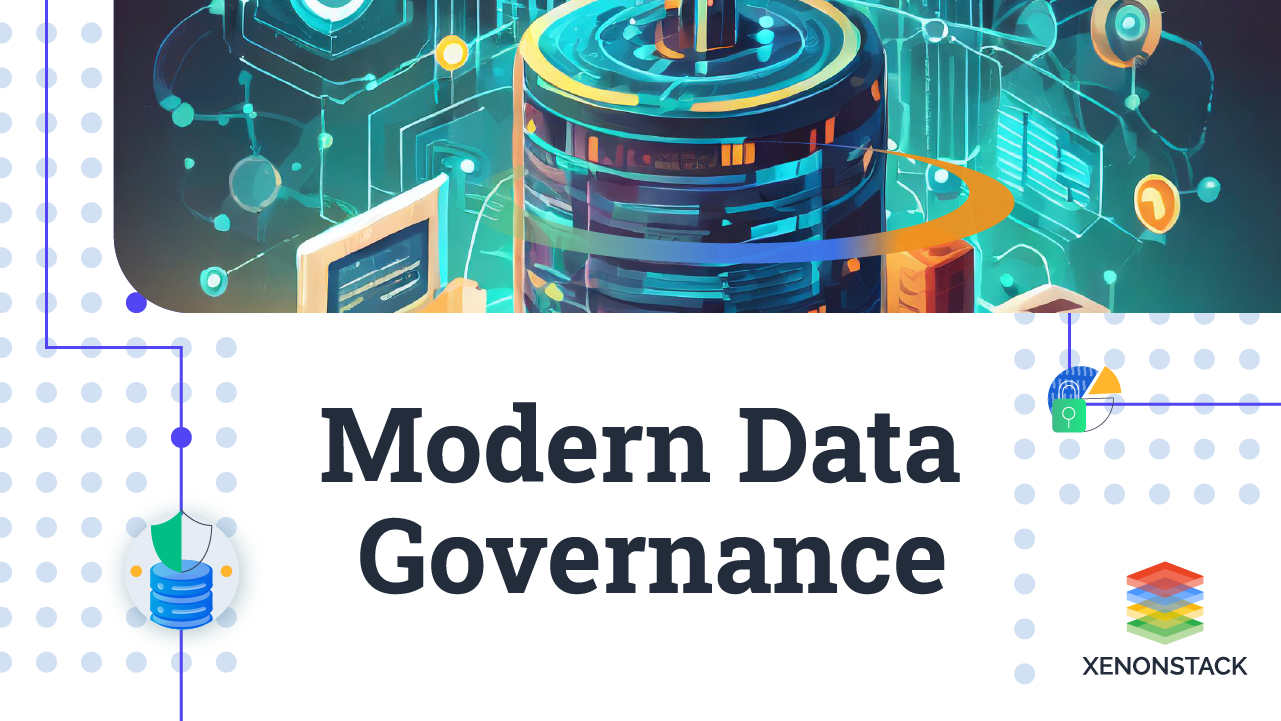
How to adopt Modern Data Governance?
Before beginning with the Governance, the organization needs to find where improvements are required in the system. Firstly, choose a specific dataset and implement it for all the datasets.
After choosing the dataset and the problem, define different teams' roles, responsibilities, and processes. The duties can be understanding data, cleaning the data, data transformation or enrichment, and, in the end, monitoring. There should be one team for each of the processes. Initiating this step on the Big Data platform also helps improve data quality. Any particular dataset and dataset owner will be responsible for the data integrity and provide the technology to ensure the integrity of the assets remains high.
After the integrity and all processes, an organization must change the culture of the organization to be master data-based rather than transaction data-based. Finally, a feedback mechanism helps improve the process. Its users have the right to raise any feedback.
Best Practices of Modern Data-Governance
However, the organizational framework alone is insufficient. Six essential best practices are required to ensure that data governance adds value.
1. Draw the attention of senior management.
Its success necessitates buy-in from business leadership.
1. The DMO's initial step is to meet with the C-suite to learn about their needs, discuss current data difficulties and constraints, and clarify data governance's function.2. The next step is forming a senior management data governance council. The council will lead the governance strategy toward business needs. They will supervise and approve initiatives to drive improvement in collaboration with the DMO.
3. After that, the DMO and the governing council should work together to identify a set of data domains and choose the business executives who will lead them. These executives lead day-to-day governance efforts, define data items, and establish quality standards.
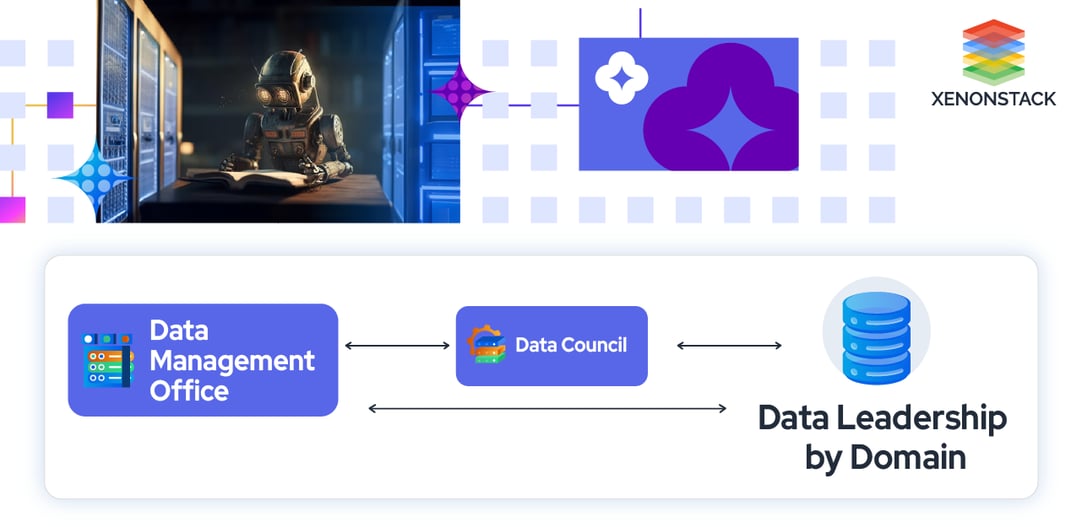
Benefits of having top-down business-leadership buy-in:
- It avoids the usual difficulties of role definition and empowerment.
- On the business side, data stewards will recognize that the project is a top priority for the company and set aside time to handle it.
- It also allows for fast resolution of data ownership disputes.
2. Use primary transformation themes.
Link governance initiatives to ongoing transformation efforts with CEO attention, such as digitization, omnichannel enablement, or enterprise-resource-planning modernization, to guarantee that governance efforts add value. These initiatives are usually reliant on the availability and quality of data.
Senior leadership buy-in is simplified, and the organizational structure is changed when governance is linked to transformation themes. Such approaches push data accountability and governance to product teams, integrating it at production and consumption rather than governance functioning independently.
3. Determine which data assets should be prioritized and where data leadership should be focused.
Many companies take a holistic approach to data governance, examining all data assets simultaneously. However, relative development in any specific area would be modest with such a broad scope, and there is a risk that it will not tie efforts to business demands. Data assets should be prioritized in two ways to be successful: by domains and by data inside each domain.
- By Domains: To build a road map for domain deployment, the data council, with the cooperation of the DMO, should prioritize domains based on transformational initiatives, regulatory requirements, and other inputs. The organization should then swiftly roll out priority domains, starting from 2 to 3, to fully operational each domain in several months.
- By data inside each domain: Prioritize data assets within each domain and domain by specifying a level of criticality (and accompanying care) for each data element. Critical elements, such as a customer's name or address, should be given special attention, including ongoing quality monitoring and transparent flow tracking across the organization. In contrast, elements used less frequently in analytics, reporting, or business operations (such as a customer's academic degree) may benefit from ad hoc quality monitoring without tracking.
4. Use the appropriate level of governance
Organizations and industries have a wide range of data governance programs.
1. Leading companies use a "needs-based" strategy, adopting the appropriate amount of governance sophistication for their business and then altering the severity level by data type.
2. The design of businesses and organizations should be tailored to the level of regulation and data complexity they confront. Organizations with several different businesses across multiple countries have more complicated requirements than those with a single firm; similarly, a high rate of data change or a low level of technology automation increases data complexity.
3. Adjust the level of governance rigor across data sets as you develop the appropriate level of governance for the organization as a whole. Legacy data standards in many organizations impose conservative quality and access limits across the board. While this reduces risk, it can also hinder innovation. Leading companies balance opportunities and threats intelligently and distinguish governance by the data set.
5. Decide on an incremental and focused implementation strategy
Apply iterative concepts to day-to-day governance. If a backlog of known data-quality issues exists, assess and reprioritize daily, working to optimize the benefit to the business as priorities move. Even if the solution isn't flawless, push to enable priority use cases as soon as possible.
This customized approach should be supported and accelerated by data governance, which should focus on resolving availability and quality issues and developing effective master data management.
6. Inspire enthusiasm for data.
Enthusiasm and dedication to data enablement play a crucial role in ensuring the high quality and safety of data. Leading companies understand this and invest in change management strategies to convert skeptics into data supporters. This aspect of the program can be the most challenging, as it requires employees to actively use and share data, while also striving to improve its quality at the source.
To encourage the appropriate behavior, successful companies employ a combination of interventions:
Role modeling by the CEO and other top leaders,- Acknowledgment for high-quality,
- Responsive suppliers,
- Innovative demonstrated use cases,
- Training qualifications and information about data-related job prospects
leveraging data and analytics successes to generate enthusiasm through events, publications, and data art.
Top Modern Data Governance Tools
It is the most important part of organizations across the world. A company’s growth is inevitable with its better. Many different tools built by various organizations provide this support to companies.
Several of its tools are available in the market, and their ranking varies based on different references and parameters.
1. Alation
Founded in 2012, Alation initially provided a data catalog platform to help companies manage their inventory and give access to their data. Alation Data Catalog remains its flagship product, but it released a related tool in September 2021. Alation App software is designed to simplify providing secure access to trusted data across IT systems, including hybrid cloud and multi-cloud computing environments.
2. Collibra
Collibra Data Governance automates key governance and stewardship tasks to keep it up to date as your business evolves. It leverages active metadata to support your organization's data up-to-date across all sources and environments.
3. Ataccama
Using a “self-driving” approach designed to automate as much as possible to improve efficiency and usability, Ataccama ONE automatically calculates data quality, classifies data, and helps prioritize and focus. Security and privacy policies can be automatically applied to all relevant data assets, so data is available to the people who need it when they need it.
4. Erwin
Acquired by Quest Software in December 2020, Erwin is a fully configurable, on-demand Impact Analyst that automatically integrates metadata from disparate data sources into a central data catalog to consolidate critical insights. Provide access through role-based contextual views, including dashboards.
5. Informatica
Informatica Data Governance includes data catalog, privacy, and data quality capabilities in an end-to-end governance solution. The company recently launched a Cloud Data Catalog. It is a comprehensive SaaS offering that combines data cataloging, data quality, and AI governance with integrated metadata-driven intelligence in the cloud.
6. Apache Atlas
Apache Atlas is a tool that makes it easy to process and maintain metadata—designed to share data across multiple tools. Provides platform independent governance. It also includes metadata management and governance capabilities for organizations to catalog their data assets.
Conclusion
Data governance helps enterprises make sure essential data is governed and protected. To learn more data management, we recommend taking the following steps -- Get an Insight into Test Data Management Process and Tools
- Learn more about Data Lineage, Best Practices and Techniques
- Get in Touch for Big Data Infrastructure