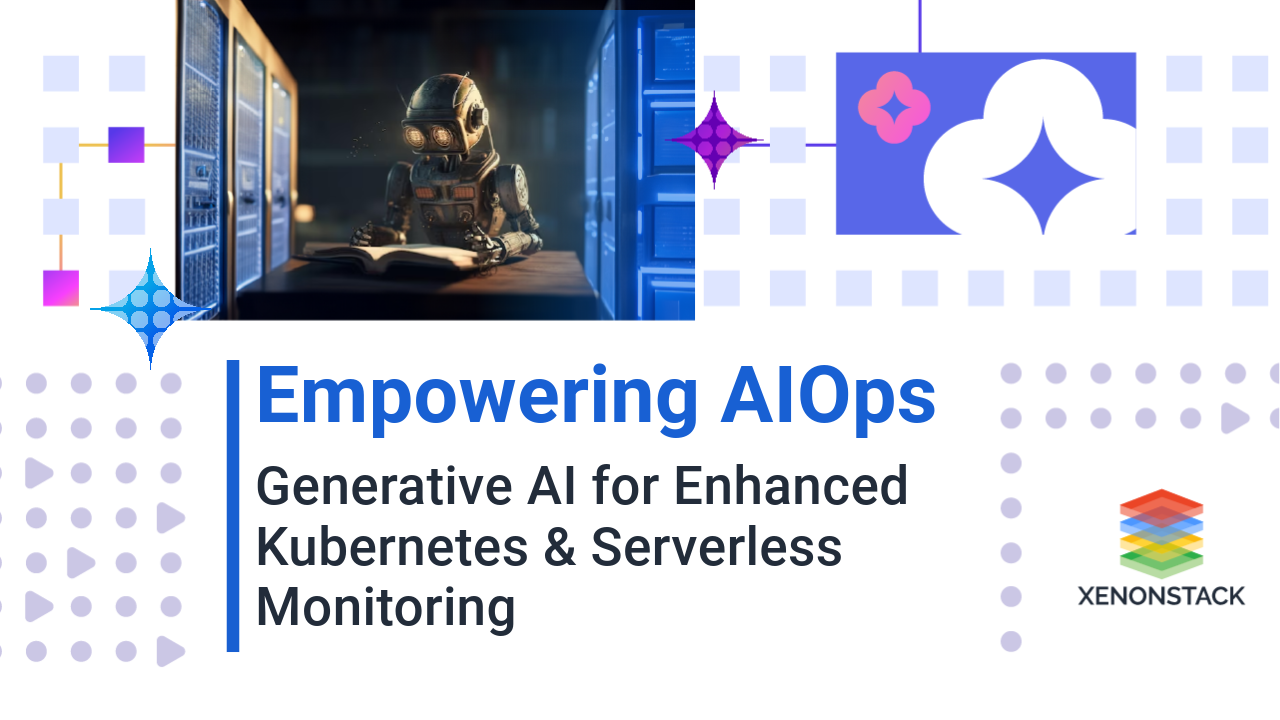
Components of a Generative AI Stack for AIOps
- Data Collection and Aggregation
To effectively monitor and analyze cloud-native environments, the AIOps platform must collect data from a wide variety of sources:
-
Kubernetes Metrics: CPU usage, memory consumption, and network traffic from the cluster.
-
Serverless Telemetry: Function invocation times, API requests, and error logs from serverless platforms.
- Generative AI Models for Monitoring
These AI models form the core of the system’s intelligence:
-
Anomaly Detection: Models that continuously monitor system health and identify outliers.
-
Predictive Analytics: Generative models that forecast system behavior, generating possible future scenarios.
-
Remediation Generators: Models that propose fixes for issues, such as scaling up resources or altering configurations.
- Automation and Orchestration Layer
An automation engine applies the insights from Generative AI to trigger corrective actions. For instance, if the AI identifies a pattern suggesting a pod failure, the orchestration layer can automate pod restarts, scaling, or network adjustments.
- Visualization and Feedback Loop
Dashboards powered by AI offer real-time visual insights into system health, and the feedback loop continuously updates the Generative AI models with new operational data, ensuring the system learns from every incident and gets smarter over time.
Kubernetes simplifies Continuous Integration and Continuous Deployment ensuring data consistency. It focuses on building and delivering software. Click to explore about, AIOps for Monitoring Kubernetes
Use Cases of AIOps with Generative AI in Cloud-Native Monitoring
- Predictive Scaling in Kubernetes
-
Challenge: High-frequency load fluctuations can stress a Kubernetes cluster, resulting in downtimes.
-
Solution: Types of AI can predict traffic loads and learn from past traffic data to extend predictions for the future. As these predictions are made, AIOps can be used to scale up Kubernetes pods before resources become constrained so the system stays alive.
- Serverless Function Optimization
-
Challenge: Observations reveal that Serverless functions experience heightened execution time, which results from inefficient code or improper API adoption.
-
Solution: The AI is provided with invocation logs, and it identifies that several code paths are suboptimal. It makes suggestions regarding increasing function execution and API usage. Besides, it can suggest the right resource profiles to improve performance with the least amount spent on resources.
- Security Monitoring and Remediation
-
Challenge: Implementing security event and threat detection in real-time, deployed on the distributed serverless and Kubernetes environment.
-
Solution: The generative AI models alert system identifies patterns of behaviors that are out of norms, thus raising suspicion of security threats. The system can handle threats by creating firewall rules or signaling other protections to minimize exposure to threats.
Benefits of Generative AI in AIOps Monitoring
The integration of Generative AI within AIOps offers several distinct advantages:
Final Thoughts
AIOps, together with Generative AI, offer a unique solution to the ever-evolving challenges of monitoring in cloud-native contexts such as Kubernetes and serverless. Using features that range beyond simple monitoring, like predictive scaling, automated root cause analysis, and self-healing, Generative AI underpinned AIOps allow organizations to do more with less, be cheaper, and keep systems availability high.
As businesses sustain a new generation of more dynamic and distributed architectures, the combination of AIOps with a Generative AI stack will be essential for achieving and sustaining operational superiority, reducing disruptions, and ensuring that systems are ready to address future needs.
Discover here about AIOps on AWS with Generative AI Explore here AIOps Solution for Telecom Industry