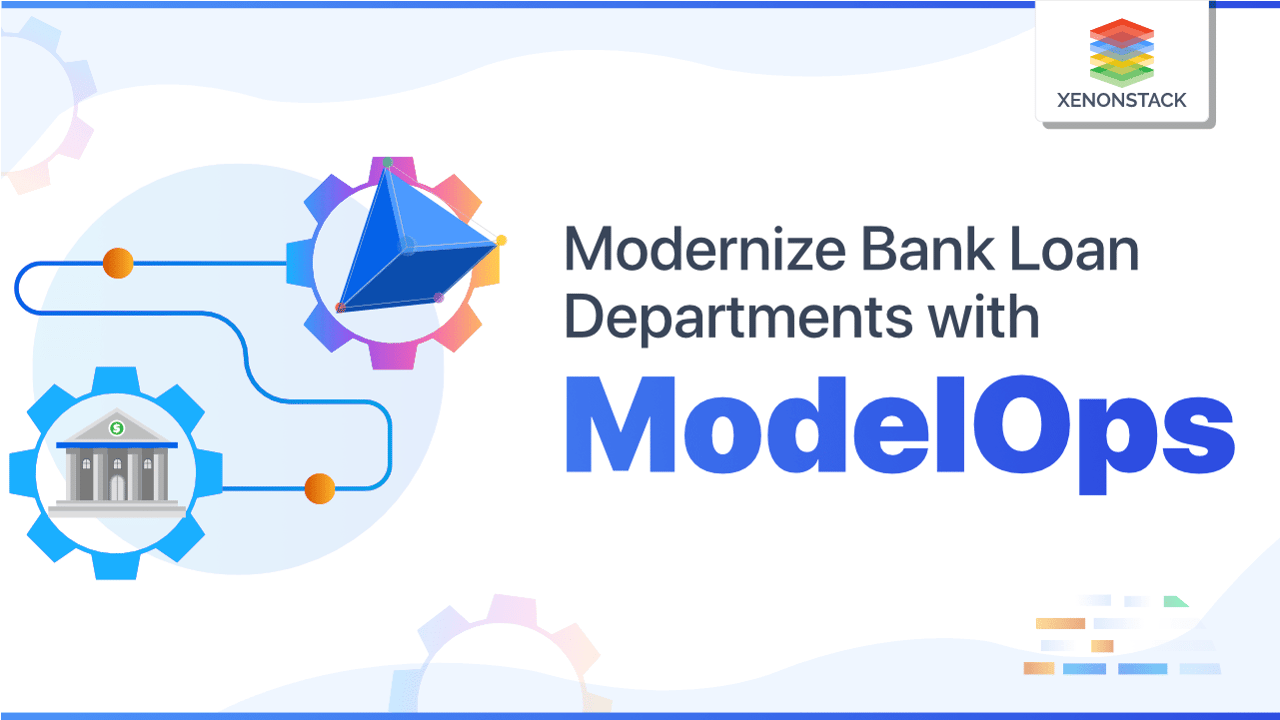
Introduction ModelOps in Lending
Banks provide loans to customers in exchange for future repayment of the loan amount with additional interest. A bank's lending department goes through a long process to determine whether a loan candidate is worth providing a loan to or not. To ensure profitability, it is important that the lending department only provides loans to customers who are less likely to default on their repayments. The information review often involves the candidate's credit history, financial assets, loan amount and duration, interest rate, housing situation, etc.
Explore here about ModelOps Platform for Operationalizing ML
Machine Learning to Aid Lending Decisions
Previously, the decision-making for choosing eligible candidates was done manually, with loan agents reviewing the records and candidate interviews, judging from experience and records to make their decisions. However, with the rise in machine learning and automation, banking loan departments have adapted technical means to analyze the candidate details and make decisions by comparing them to past records.
Using machine learning for analyzing loan candidate details is an effective measure because:
-
ML models perform analyses much faster and are free from human error, negligence, and biases, thus making their predictions more accurate.
-
Machine learning models can utilize various statistical and mathematical tools to learn from customer details and default status records and show relationships between different variables. These relationships can be linear or non-linear.
-
Utilizing the ML approach also solves the problem of dimensionality and scalability since machine learning can use large datasets to make predictions, which other approaches cannot.
-
Inference gained from ML models can be shared widely and is backed by mathematical reasoning.
Classification models have proven to be very effective in classifying potential loan candidates as likely to default or not. Statistical models like Logistic Regression, Decision Trees, Random Forests, and xgboost have been trained and tested on datasets of past loan candidates with 100s of features. These models are then used to classify real-time candidates based on their records.
Once the models are trained and tested, they can be deployed in the bank's loan department to aid decision-making. To ensure that the developed model works effectively and adds value to the decision-making process, it is important to regularly monitor and update the model. And architectures like Model Operations are designed to handle such operations.
Read here about Governing AI initiatives with ModelOps
ModelOps for Lending Models
Bank loan departments often have a highly accurate model, but they cannot operationalize it. Model operations often include collaboration between ML operations, IT operations, and Cloud and Dev operations. From building and deploying the model to regular monitoring and auditing, well-established Model operations are needed to handle the model lifecycle.
If the lending classification model loses its accuracy with new data (also known as model drift), regular monitoring can alert the authorities and developers to take action. In such situations, models are either retrained or discontinued altogether.
There can be instances when the loan approval model has been deployed on the cloud, and some latency issue occurs in the future. In such cases, the data scientist would not be authorized to access the cloud infrastructure. Thus, IT solves this problem.
It can also help scale the models. Since these operations are not isolated to one banking sector or department, data and insights from other departments can be integrated into the model, and the model inferences can be shared with other departments. Integration of Cloud operations aids in sector-wide model governance and monitoring.
Explore here What is ModelOps and its Operationalization?
Setting up ModelOps Infrastructure
To scale their artificial intelligence efforts and apply their developed models to 100s of use cases, bank loan departments must set up an Infrastructure. This often involves building the architecture from scratch and integrating different services that different departments use. It can be confusing and time-consuming without proper experience and guidance in architecture.
Another way of integrating the infrastructure in the model production efforts is to consult third-party providers to partner with the banking department and build the infrastructure according to their needs.
Consulting ModelOps platforms can be an effective way to integrate this technology. The platforms are online platforms that provide end-to-end model governance services on their servers. Users can build their models and personalize their production pipeline by choosing from the various tools available.
Conclusion
Bank loan departments use machine learning models for decision-making when choosing eligible loan candidates since machine learning provides many benefits over manual analysis problems. A collaboration between different departments is needed to operationalize the machine learning models through different branches and departments of the bank. It solves the disconnection between different operational departments. The infrastructure provides an ecosystem to govern a model's life cycle, ensure that the model is monitored and audited regularly, and provide desired value to the customers.
- Discover here What is Multi-Cloud ModelOps?
- Explore here about MLOps Roadmap for Interpretability