In today’s rapidly advancing technological landscape, 3D vision and depth estimation are revolutionizing how machines interact with the physical world. These technologies, essential for applications such as autonomous vehicles, robotics, and augmented reality (AR), are projected to see exponential growth.
According to a report by MarketsandMarkets, the global 3D sensor market, which heavily relies on depth estimation, is expected to grow from USD 2.8 billion in 2020 to USD 7.9 billion by 2025, at a CAGR of 22.5%.
This surge highlights the critical role 3D vision technologies play in enhancing machine intelligence and automation across industries. In this blog, we’ll explore the mechanics behind 3D vision and depth estimation, key technologies driving this field, real-world use cases, and the challenges and benefits shaping its future.
What is 3D Vision?
3D vision means the ability of machines to process the information coming from the two-dimensional images and interpret them as images that were created in the three-dimensional space. This capability is useful for object recognition, navigation, or scene reconstruction, among others. By doing so, machines can emulate depth perception in that they can distinguish objects, their relative location, as well as the size and shape of objects in a particular environment.
As will be described, the value of 3D vision is in the ways it provides additional information to improve Machine Intelligence’s engagement with the physical environment. Through the above methods and using several technologies, machines can learn from their environments and situations and make better performances on several tasks via processing 3D data information.
The computer vision based technology that detects and analyzes human posture. Taken From Article, Human Pose Estimation for Sport Analytics
How It Works
3D vision requires depth estimation, which is an ability to estimate distances from the viewpoint of an image, surprisingly obtained from 2D pictures. This process helps in the generation of depth, which is the measurement of scenes in relation to one another. Several methodologies are used for in-depth estimation, categorized into hardware-based and software-based techniques:
Hardware-Based Techniques
-
Stereo Vision: Two cameras spaced apart are used to take pictures; the difference in these pictures helps to determine depth.
-
Time-of-Flight (ToF): Calculates the time taken by a light signal emitted towards an object and reflected to first assess the depth.
-
LiDAR uses lasers to measure distances and can create detailed 3D models of the environment.
Software-Based Techniques
-
Single-Image Depth Estimation: This technique uses deep learning algorithms to estimate geometry from a single image, frequently with neural networks derived from numerous data sets.
-
Multi-View Geometry: Takes several pictures from various views to calculate depth information through geometry.
Notable Key Features
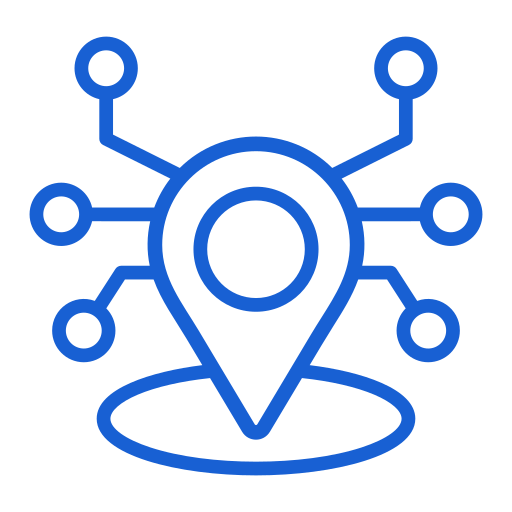
Real-time Depth Mapping
Allows machines to instantly measure and interpret depth, improving obstacle detection and pitch calibration
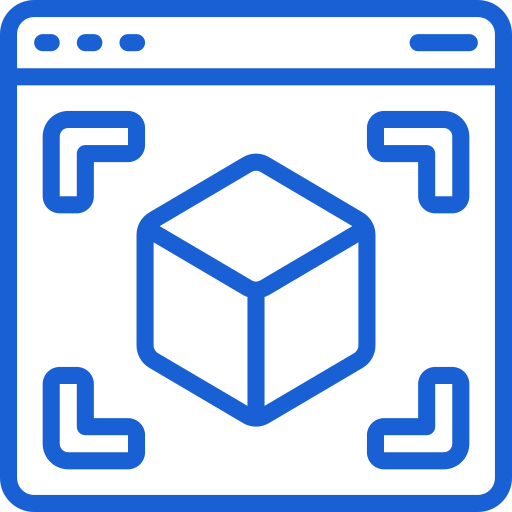
Accurate Object Identification
Enables precise identification and classification of objects in a changing three-dimensional environment
What It Does
3D vision and depth estimation technologies empower machines to perform complex tasks, such as Driving through terrains by themselves. Engaging with objects and other people in a more realistic and/or functional way. Improving Customer Experience of AR and VR Applications.
How It Helps
These technologies Enhance the use of automation across various sectors, particularly the manufacturing and logistics sectors, by providing machines with the ability to perceive depth and spatial relationships. They also improve safety for the more dangerous self-driving cars by improving the ability to see and recognize objects. Finally, they introduce new solutions in the sphere of medical imaging, which would provide better diagnosis and treatment.
Impact in the Real World
The potential use of 3D visions and depth estimation is greatly influential in detail. These technologies are used in:
-
Autonomous Vehicles: Safe navigation and collision avoidance among the entities are made possible by the use of.
-
Robotics: Enhancing end-effector dexterity and force control in robots.
-
Augmented Reality: Overlaid digital information of augmented reality that helps in developing a cozy environment.
Challenges Faced Ahead
Despite their potential, 3D visions and depth estimation face several challenges:
-
Computational Complexity: The use of 3D information implies a high computational load, which makes real-time execution challenging.
-
Environmental Factors: Depth estimation depends on lighting conditions, occlusions, and textureless surfaces. The cases shown below are examples.
-
Integration with Existing Systems: Adhering to present-day technologies and frameworks may also be difficult.
Benefits of Technology
-
Enhanced Perception: When machines are applied to enhance their efficiency and reliability, the environment is recognized as being better understood.
-
Improved Accuracy: Moreover, estimating depth improves the size and analysis of the results.
-
Automation: These technologies make it possible to automate some difficult tasks in different fields of endeavor.
Why This Is Important
This makes the perception and understanding of 3D space very important for AI and robotics. As we move further into the age of industrial automation, the utilization of intelligent systems, 3D visions, and depth estimation will remain a major influence on the technology being developed.
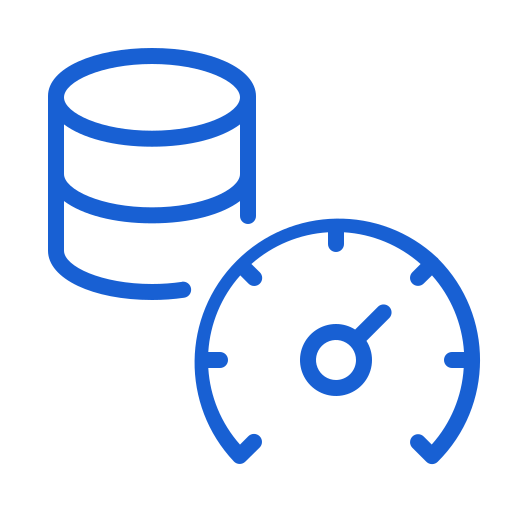
Data Limitations
Creating accurate depth maps may, however, be a challenge, especially if high-quality training set data is not available
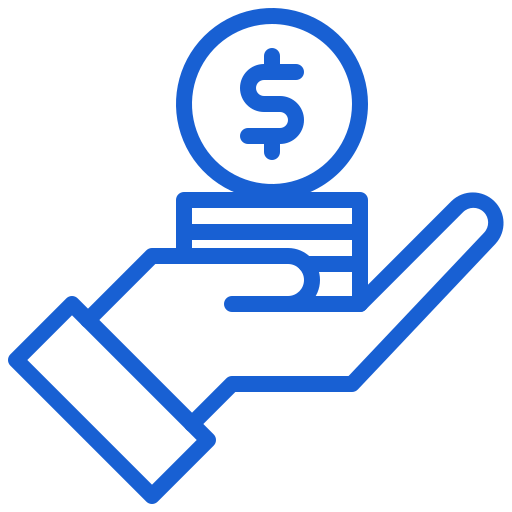
Financial Constraint
Parallel I/O hardware solutions are costly and can pose a problem for organizations that are not so financially endowed
Use Case: Autonomous Vehicles
Problem Statement
Self-driving cars derive their operations from detecting the surrounding environment in as much detail as possible. However, getting the right image may be made difficult by challenges such as dynamic objects, changing light conditions, and hilly terrains.
To meet safety concerns in various road conditions and be dependable on the road. Including depth estimation technologies on current vehicle systems. Full consideration of the challenging questions regarding regulation and standards for the vehicle’s autonomous operation.
Solution
Hardware sensor approaches such as LiDAR and stereo vision combined with software sensor approaches like deep learning algorithms can give autonomous vehicles a more powerful perception. This integration enables instant reception of obstacles in space and their identification.
Architecture Diagram for 3D Vision and Depth Estimation
Key Components of the Solution
Input Sources:
-
Cameras: Capture 2D images for depth estimation.
-
LiDAR Sensors: Provide accurate distance measurements.
Data Processing Layer:
Preprocessing Module-
Image enhancement and noise reduction.
-
Calibration of camera and LiDAR data.
-
Hardware-based Techniques: Stereo Vision, Time-of-Flight, LiDAR.
-
Software-based Techniques: Single-Image Depth Estimation (Deep Learning), Multi-View Geometry.
Fusion Layer:
-
Data Fusion Engine: Combines data from cameras and LiDAR sensors to create comprehensive depth maps and 3D representations.
Analytics and Machine Learning:
-
Object Recognition Module: Utilizes trained models (e.g., CNNs) for detecting and classifying objects.
-
Scene Reconstruction Module: Rebuilds the environment in 3D based on the processed depth data.
-
Deep Learning Algorithms: For computation of results and decision making of special occurrences in our day-to-day life.
Output:
-
Depth Maps: Visual representations of distances in the scene.
-
3D Models: Generated models for use in applications such as AR/VR, robotics, and autonomous navigation.
Final Thoughts
Seeing three-dimensionally and measuring depth are fundamental to the future sensors and perception for use in many industries. As these technologies progress, they will progress to upgrading automation, advancing safety, and changing the way we interface with our reality. Substantial investigations into questions related to this area have been conducted, and this research indicates that consistent advancements in this domain hold great potential for the future of technology that will revolutionize the capability of machines to perceive three dimensions and estimate depth, making these features indispensable.
Learn more about Augmented Reality (AR) and Virtual Reality (VR) Explore more about Vision Transformers (ViTs)