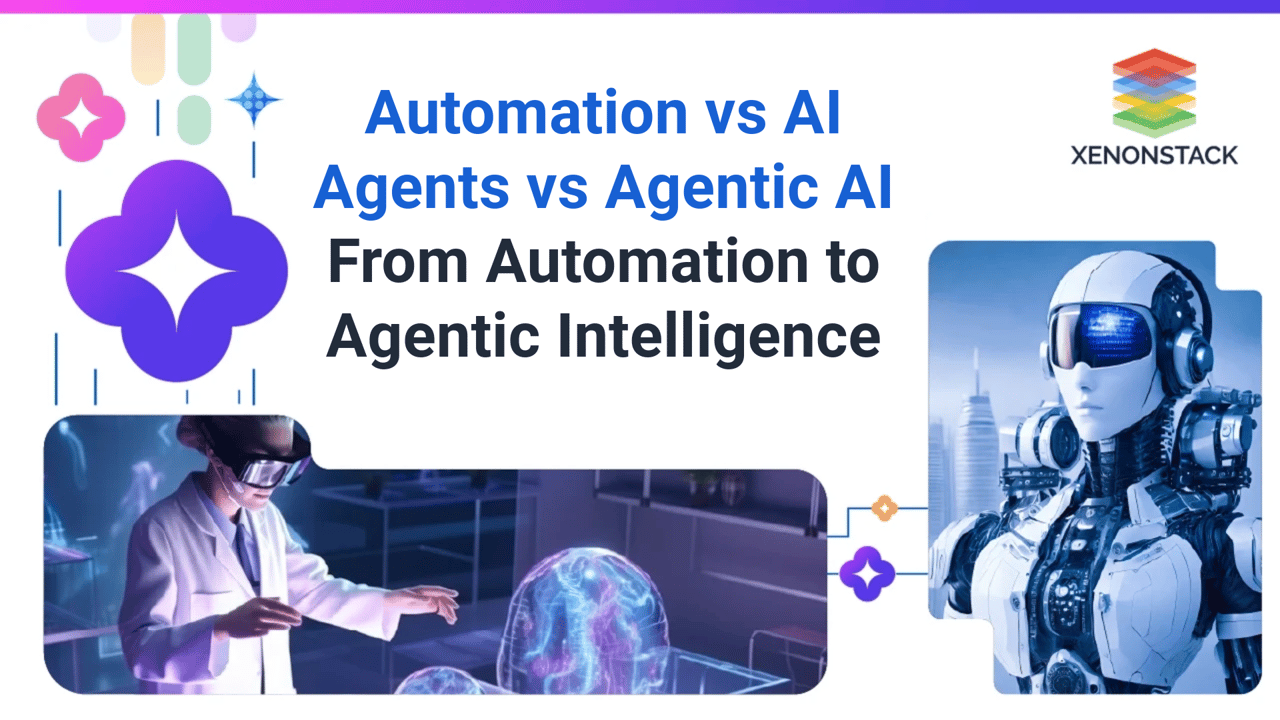
The digital transformation of businesses has led to the adoption of various technologies aimed at streamlining operations and improving efficiency. Among these technologies, automation, Agentic AI, Autonomous Agents, and AI agents are becoming essential tools for optimizing tasks and processes. However, while all three can enhance business outcomes, they serve different purposes and offer unique strengths and weaknesses.
According to Google Trends, interest in this topic is rapidly growing and shows no signs of slowing down. What truly stands out, however, is the intense debate — and, let's face it, some controversy — surrounding the definitions of automation, AI workflows, and AI agents. Clearly, this subject has sparked significant attention, and in some cases, even heated discussions.
In this blog, we will explore the differences between automations, AI Workflows, and AI agents, detailing their capabilities, strengths, weaknesses, and when to use each.
Understanding Agentic AI
Agentic AI refers to AI-driven systems that autonomously execute tasks, adapt to dynamic environments, and make decisions with minimal human intervention. Unlike traditional automation or AI workflows, Agentic AI combines reasoning, decision-making, and learning to handle complex, non-deterministic tasks. These systems operate independently, responding to new variables in real-time while optimizing outcomes based on evolving data.
Agentic AI is widely used in scenarios where decision-making must be adaptive, such as AI-driven cybersecurity, autonomous supply chain management, and AI-powered business strategy recommendations.
Tasks Best Handled by Agentic AI
Agentic AI is ideal for tasks that require dynamic decision-making, real-time adaptability, and learning from past interactions. These include:
- Autonomous IT Operations: Continuously monitors systems, detects anomalies, and resolves issues proactively.
- Intelligent Process Optimization: Enhances business operations by analyzing workflows and recommending improvements.
- Decision Intelligence: Supports executives with AI-driven insights, enabling faster, data-backed decisions.
- Personalized AI Assistants: Continuously learn from user interactions to refine recommendations and automate tasks.
- Supply Chain Adaptability: Predicts demand fluctuations and optimizes logistics dynamically.
Strengths of Agentic AI
- Autonomy: Capable of executing complex workflows with minimal human input.
- Adaptability: Learns from new data and adjusts strategies to improve outcomes over time.
- Scalability: Easily expands across various business functions to handle increasingly complex operations.
- Proactive Decision-Making: Anticipates challenges and suggests solutions before issues arise.
Weaknesses of Agentic AI
-
Unpredictability: Since Agentic AI adapts and learns, outcomes may not always be fully predictable.
-
Complex Implementation: Requires advanced AI models, robust data pipelines, and significant computational resources.
- Oversight Challenges: Continuous monitoring is needed to ensure AI decisions align with business goals and ethical considerations.
What is Automation?
Automations refer to systems designed to execute predefined, rule-based tasks automatically without requiring manual intervention. These tasks are typically deterministic, meaning that for every specific input, the output will always be the same.
Examples of automation include systems that automatically schedule appointments, send emails, or generate routine reports. The strength of automation lies in its ability to execute tasks quickly and consistently.
Tasks Best Handled by Automations
Automations shine when handling predefined deterministic tasks. These are tasks that can be clearly defined and do not change over time. Some examples include:
- Scheduling appointments
- Sending recurring emails
- Processing data in batches
- Generating reports based on predefined formats
Strengths of Automations
-
Reliability: Automations provide reliable outcomes by following a fixed set of rules, ensuring that the task is completed consistently.
-
Speed: They execute tasks much faster than humans, which is especially beneficial for tasks that are time-sensitive.
-
Cost-effective: Automating repetitive tasks can save businesses time and reduce operational costs.
Weaknesses of Automations
-
Limited Adaptability: Automations are limited to tasks explicitly programmed into them. If a new task or situation arises, automations will not be able to adapt without significant reprogramming.
-
Inability to Handle Complex Decisions: Automations are ill-equipped to handle tasks that involve dynamic decision-making or creative problem-solving.
What Are AI Workflows?
An AI workflow extends the concept of automation by incorporating artificial intelligence (AI), such as Large Language Models (LLMs) like ChatGPT, into the process. These workflows still follow predefined steps, but they include AI-powered elements that make the process more flexible.
AI workflows can automate several steps in a process and call an AI model via an API for specific tasks that require more than just rule-based logic. For example, an AI workflow might automatically categorize incoming support tickets and then use a language model to generate a response.
Tasks Best Handled by AI Workflows
AI workflows are best for tasks that require some flexibility while maintaining a deterministic framework. Examples include:
-
Customer support ticket routing based on predefined categories and language models.
-
Content generation, where predefined rules guide the structure, but AI creates personalized content.
-
Data analysis where complex rules need to be applied to dynamic inputs.
Strengths of AI Workflows
-
Pattern Recognition: AI workflows excel at identifying patterns in large datasets and can make decisions based on this analysis.
-
Flexibility: These workflows allow for more flexibility than traditional automations, handling tasks with more dynamic input and rules.
-
Complex Rule Handling: AI workflows can manage sophisticated decision-making scenarios that might be difficult for a purely automated system.
Weaknesses of AI Workflows
-
Training Data Dependency: AI workflows often rely on large datasets to train models, and without sufficient data, the AI may not perform well.
-
Harder to Debug: Since AI decision-making processes are often less transparent than deterministic automation, troubleshooting errors in AI workflows can be more challenging.
-
Potential for Bias: The effectiveness of AI workflows depends on the quality of the data used for training. Incomplete or biased data can lead to inaccurate results.
Discover how Akira AI Agents power autonomous operations with intelligent decision-making.
- Agent Analyst – Transforms data into actionable insights for smarter business strategies.
- Agent Force – Automates workflows and enhances operational efficiency across teams.
- Agent SRE – Ensures system reliability with proactive monitoring and self-healing capabilities.
What Are AI Agents?
An AI agent is an autonomous program capable of handling non-deterministic tasks. These agents can adapt to changing conditions, learn from their environment, and make decisions without human intervention. AI Agents for Autonomous Operations are designed to operate independently, handling tasks that require decision-making based on unpredictable or evolving variables
AI agents are used in applications like autonomous vehicles, trading algorithms, and predictive maintenance, where the system must operate in real-time and adapt to unforeseen circumstances.
Tasks Best Handled by AI Agents
AI agents are suited for tasks that require non-deterministic decision-making and involve interacting with dynamic environments. These include:
- Autonomous decision-making (e.g., stock market trading algorithms)
- Learning from interactions (e.g., virtual assistants adapting to user preferences)
- Problem-solving in uncertain environments (e.g., AI-driven research or product development)
Strengths of AI Agents
-
Autonomy: AI agents can operate independently, making decisions without human input.
-
Adaptability: These agents are capable of learning from their environment and adjusting their behavior accordingly.
-
Problem-Solving: AI agents excel at tackling complex, adaptive problems in real-time.
Weaknesses of AI Agents
-
Unpredictable Outcomes: Since AI agents are designed to learn and adapt, the outcomes of their actions may not always be predictable, leading to occasional unintended consequences.
-
Complexity: Developing AI agents is often more complex than creating automations or AI workflows. They require sophisticated algorithms and considerable computational resources.
-
Reliability: Because AI agents are autonomous, their decision-making processes can sometimes lead to errors or actions that are difficult to oversee.
Automations vs. Agentic AI vs. AI Agents: Key Differences
Here’s a quick summary of the key differences between automations, Agentic AI, and AI agents
Aspect | Automations | Agentic AI | AI Agents |
---|---|---|---|
Definition | Execute predefined, rule-based tasks automatically. | Automations that call LLMs (e.g., ChatGPT) via API for one or more steps. | Programs designed to perform non-deterministic tasks autonomously. |
Best for | Predefined, deterministic tasks. | Deterministic tasks requiring some flexibility. | Non-deterministic, adaptive tasks. |
Strengths | Reliable, fast, cost-effective. | Pattern recognition, flexibility, and handling complex rules. | Autonomy, adaptability, problem-solving. |
Weaknesses | Limited adaptability, no complex decision-making. | Requires data to train models, harder to debug. | Less reliable, unpredictable outcomes. |
Choosing the Right Solution: AI Agents, Automation, or AI workflows
When choosing between automation, AI workflows, and AI agents, consider these key factors:
Task Complexity:
-
Automation is ideal for simple, repetitive tasks.
-
AI workflows excel in tasks that require flexible rules and pattern recognition.
-
AI agents are essential for tasks that involve adaptive decision-making and complex scenarios.
Data Requirements:
-
Automation needs little data to operate.
-
AI workflows and agents require substantial data for training and ongoing improvement.
Reliability vs. Adaptability:
-
Automation delivers consistent results with speed and reliability.
-
AI workflows and agents prioritize flexibility and adaptability, often at the cost of reliability.
Build Custom Agentic Workflows with Xenonstack
Talk to our experts about building custom Agentic AI workflows with Xenonstack and learn how industries and various departments leverage Agentic Workflows and Decision Intelligence to become more decision-centric. By implementing Agentic AI, organizations can automate and optimize their operations, empowering teams to make real-time, adaptive decisions, while streamlining workflows and boosting efficiency.