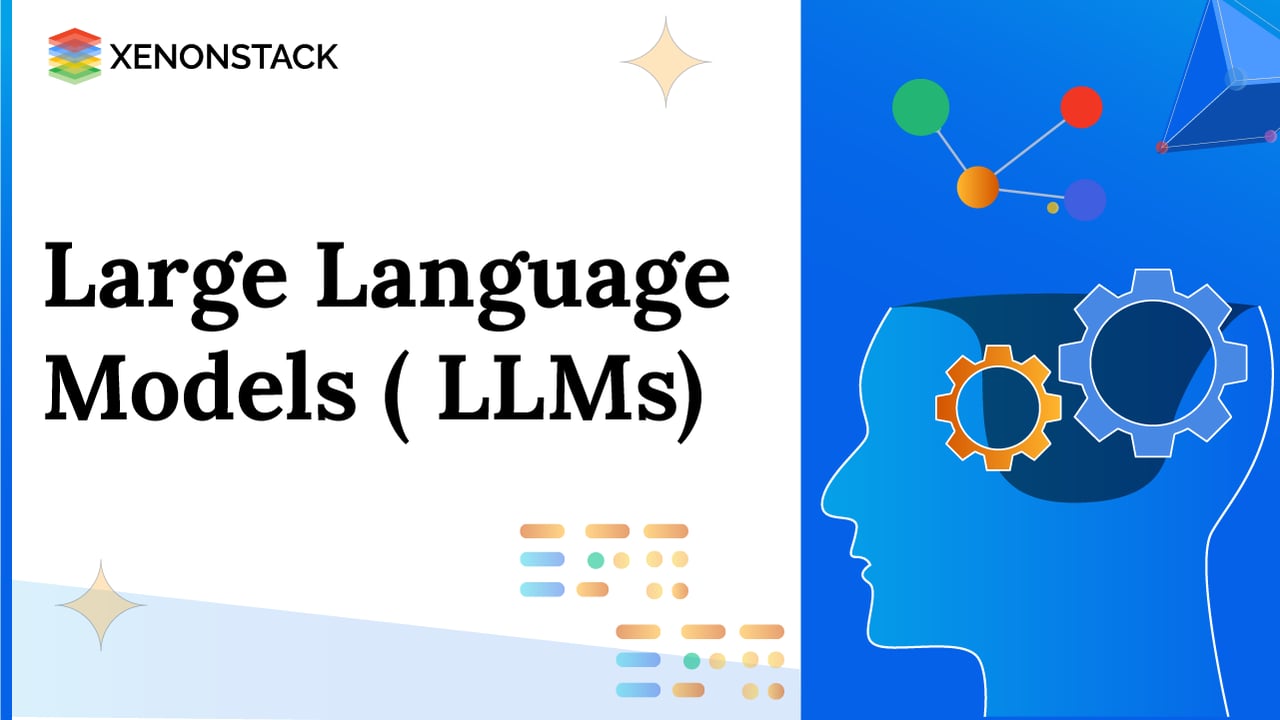
Introduction
Discover the revolutionary power of ChatGPT, an AI marvel that has captivated the world and unleashed an unprecedented wave of creativity. With its astonishing ability to simulate human conversation and decision-making, ChatGPT has propelled AI into the spotlight, unveiling its transformative potential. In an astonishing feat, it has amassed a staggering 100 million monthly active users in just two months, cementing its status as the fastest-growing consumer application in history.
At its core, ChatGPT harnesses the immense capabilities of large language models (LLMs), marking a pivotal moment in AI advancement. These models have cracked the complexity of language, enabling machines to comprehend and generate creative responses autonomously. Through extensive pre-training and adaptability, LLMs prove highly versatile, empowering businesses across industries to revolutionize operations, drive scientific breakthroughs, and shape society. The impact on human productivity and creativity is profound, as Accenture's study reveals that LLMs like GPT-4 have the potential to transform up to 40% of working hours, elevating productivity through augmentation and automation of language tasks, which dominate 62% of employees' time.
Large Language Models
A large language model (LLM) is a generative mathematical model that analyzes the statistical distribution of tokens (words, parts of words, or individual characters) in a vast collection of human-generated text. LLMs, such as the core component of an AI assistant like ChatGPT, have a well-defined function and can provide precise and accurate responses based on the statistical likelihood of specific word sequences.
LLMs have demonstrated positive impacts in various scenarios. They excel in tasks that involve generating coherent and contextually relevant text based on given prompts. For example, when asked about the first person to walk on the moon, an LLM can accurately respond with "Neil Armstrong" based on the statistical distribution of words in the public corpus. Similarly, when prompted with incomplete phrases like "Twinkle twinkle," an LLM can generate the appropriate completion like "little star," reflecting its understanding of common language patterns.
These models offer practical utility and convenience in applications where language generation and comprehension are essential. They can assist users by providing information, suggestions, or creative responses, contributing to improved productivity and efficiency in tasks involving language. LLMs are powerful tools for automating language-related activities, such as generating text for speeches, emails, lectures, or papers, thus saving time and enhancing human capabilities.
By leveraging LLMs, individuals can harness the vast knowledge contained within the public corpus of text to enhance their understanding, creativity, and problem-solving abilities. These models act as valuable resources, augmenting human intelligence and facilitating communication in a wide range of domains. Emphasizing their core function of generating statistically likely word sequences allows users and developers to appreciate and utilize the practical benefits of LLMs, avoiding misleading claims about belief, knowledge, understanding, self, or consciousness that may not accurately reflect their capabilities.
Large Language Models: Opportunities and Research Challenges
Large language models (LLMs) have the potential to transform enterprises in a number of ways. They can be used to automate tasks, improve customer service, generate content, and make better decisions.Here are some of the opportunities that LLMs offer to enterprises:
- Automation:
LLMs can be used to automate a variety of tasks, such as customer service, data entry, and content generation. This can free up employees to focus on more strategic tasks. - Customer service:
LLMs can be used to create chatbots that can answer customer questions and resolve issues. This can improve customer satisfaction and reduce the cost of customer service. - Content generation:
LLMs can be used to generate content, such as news articles, blog posts, and social media posts. This can help enterprises to reach a wider audience and to improve their online presence. - Decision-making:
LLMs can be used to analyze data and to make predictions. This can help enterprises to make better decisions about things like product development, marketing, and pricing.
- Bias:
LLMs are trained on massive datasets of text, which can contain biases. This can lead to LLMs generating biased output. - Privacy:
LLMs need to be trained on massive datasets of text, which can contain sensitive information. This raises privacy concerns. - Security:
LLMs can be used to generate text that is malicious or harmful. This raises security concerns.
Despite these challenges, LLMs have the potential to revolutionize the way enterprises operate. As the research challenges are addressed, LLMs will become even more powerful and will be able to be used to solve even more complex problems.
Here are some specific examples of how LLMs are being used by enterprises today:
GPT-3 (and ChatGPT), LaMDA, Character.ai, Megatron-Turing NLG – Text generation useful especially for dialogue with humans, as well as copywriting, translation, and other tasks
Anthropic.ai:
Product focused on optimizing the sales process via chatbots and other LLM-powered tools
Codex (and Copilot), CodeGen :
Code generation tools that provide auto-complete suggestions as well as the creation of entire code blocks
As the technology continues to develop, we can expect to see even more innovative applications of LLMs in the future.
Use Case: Reimagining Evaluation for Conversational Recommendation with Large Language Models
Problem Statement:
The current evaluation protocol for conversational recommendation systems (CRSs) is based on matching the system's recommendations with the ground-truth items or utterances generated by human annotators. However, this approach has several limitations. First, it does not take into account the interactive nature of CRSs, where the user and system engage in a dialogue to reach a recommendation. Second, it does not measure the explainability of the system's recommendations, which is an important factor for user trust.
Solutions of LLMs:
A novel evaluation approach called iEvaLM is introduced, which is based on large language models (LLMs). iEvaLM simulates the interaction between a user and a CRS by using an LLM-based user simulator. The user simulator generates a sequence of utterances, and the CRS responds with a recommendation. The quality of the recommendation is then evaluated based on the user simulator's satisfaction.
Benefits of LLMs:
The benefits of iEvaLM include:
- It takes into account the interactive nature of CRSs.
- It measures the explainability of the system's recommendations.
- It is a more flexible and easy-to-use evaluation framework than the current protocol.
Building a Data-Centric Platform for Generative AI and LLMs at Snowflake
Snowflake is making it easier for businesses to use large language models (LLMs) to gain insights from unstructured data and boost productivity.The company has acquired Applica, a leader in LLM technology, and is integrating its technology into Snowflake. This will allow businesses to run LLMs on their data without having to worry about security or governance.
In addition, Snowflake is developing LLM-powered search experiences and code autocomplete features that will make it easier for developers to use LLMs.
These new capabilities will help businesses to make better use of their data and to become more efficient.
Here are some of the key benefits of using LLMs with Snowflake:
- Increased insights:
LLMs can be used to gain insights from unstructured data that would be difficult or impossible to obtain using traditional methods. - Improved productivity:
LLMs can automate many tasks that are currently done manually, freeing up employees to focus on more strategic work. - Enhanced customer experience:
LLMs can be used to create more personalized and engaging customer experiences.
Future Scope of Large Language Model ( LLMs)
We are entering an era of transformation where access to information, content creation, customer service, and business operations will be fundamentally changed. Generative AI integrated into the digital core of enterprises will optimize tasks, enhance human capabilities, and create new growth opportunities. However, to fully realize the potential of these technologies, it is crucial to reimagine work processes and ensure that people can keep up with technological advancements. Companies must invest in evolving operations and providing training to employees as much as they invest in the technology itself. This is the time for organizations to leverage AI breakthroughs to redefine their performance and reshape their industries. To drive successful reinvention, it is essential for employees at all levels, from the C-suite to the front line, to develop a Technology Quotient (TQ) that demonstrates their understanding of transformative technologies and their ability to harness technology and human ingenuity.
- Read more about Top Generative AI Use Cases and Applications
- Read about Generative AI Solutions is Improving Public Safety in Smart Cities