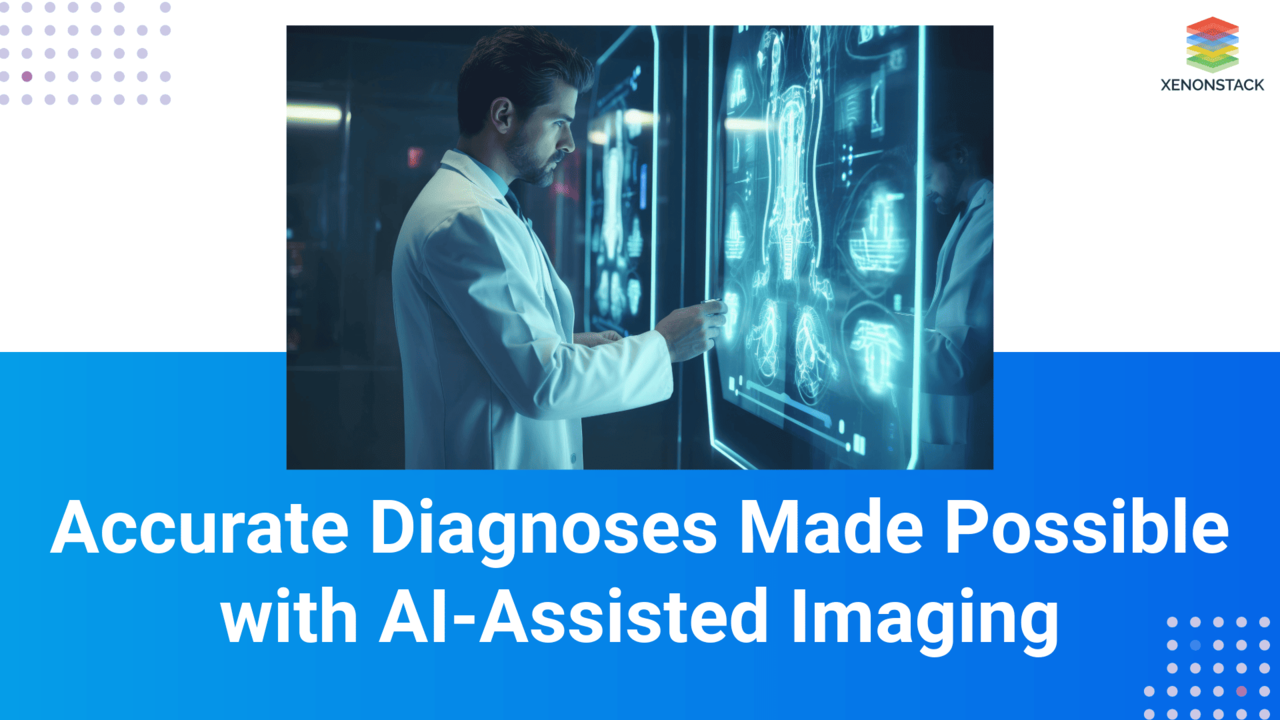
Artificial Intelligence (AI) has cemented its place in the medical world as its role in healthcare is changing the face of medical imaging. In this context, AI is making radiologists, physicians, and other caretakers more capable of handling many diagnostic challenges, finally enhancing the quality and speed of diagnosis. Moving from identifying the first symptoms of cancer or other diseases to interpreting intricate brain scans, artificial intelligence-enhanced medical imaging is today’s miraculous tool in doctors’ hands.
The Role of AI in Medical Imaging
Medical imaging refers to techniques like X-rays, CT scans, MRIs, ultrasounds, and mammograms, which provide visual insights into the body for diagnostic and treatment purposes. Traditionally, radiologists analyze these images manually. However, due to the high volume of scans, the process can be time-consuming and prone to human error. AI, particularly machine learning (ML) and deep learning enhance medical imaging through automated analysis, detection, and diagnosis of abnormalities. AI models, trained on massive datasets, can identify patterns and anomalies with unmatched precision, assisting radiologists in making faster and more accurate diagnoses.
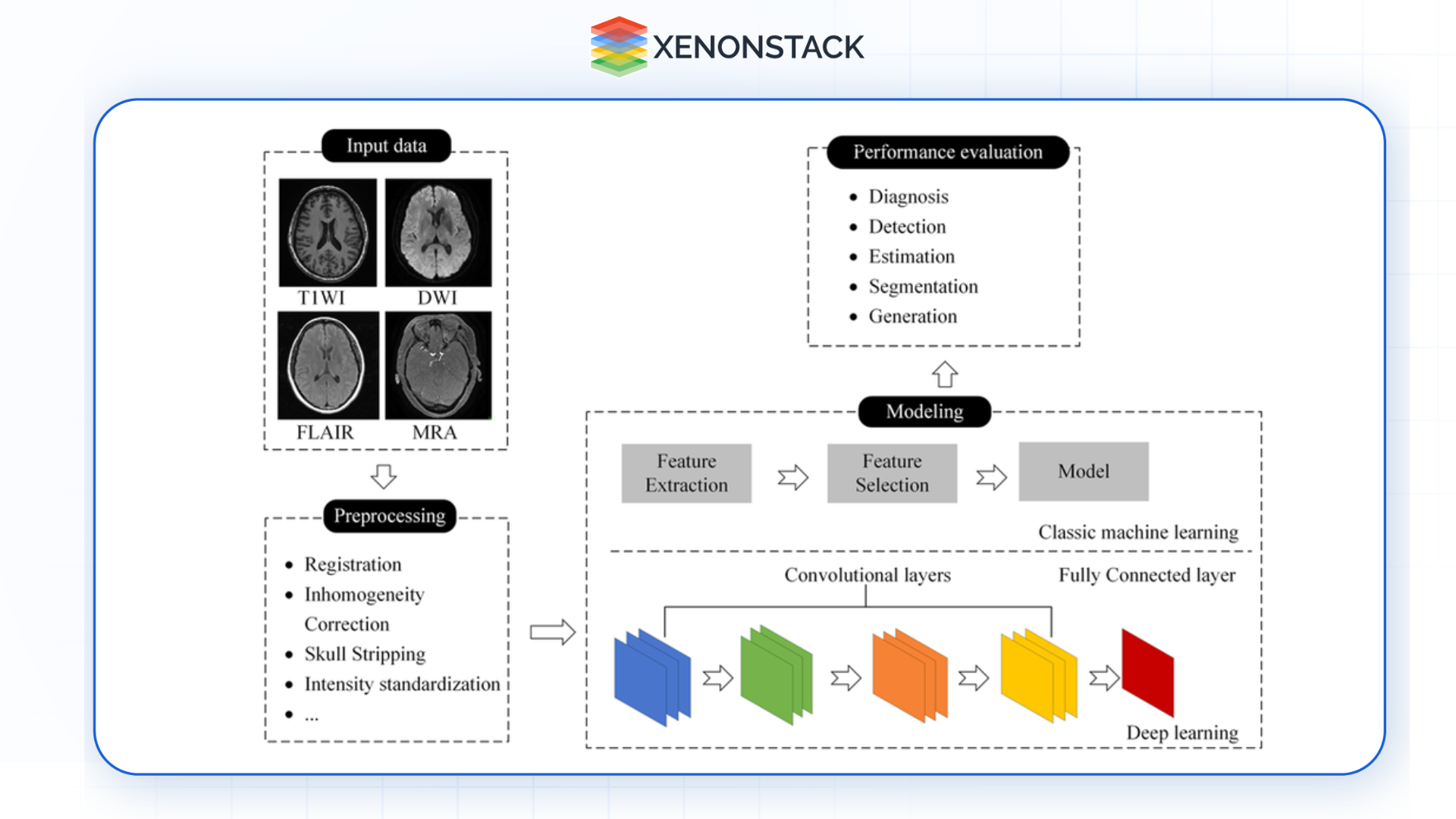
How AI Improves Diagnosis Accuracy
Early Detection of Diseases
AI systems can find infrequent and less noticeable anomalies in medical images that the human eye cannot easily pick up. This is crucial for diagnosing early diseases, including cancer, cardiovascular, and neurodegenerative diseases.
-
Example: In diagnosing breast cancer, AI models help analyze mammograms, identifying microcalcifications and masses, thus supporting the early detection of malignancies.
Pattern Recognition
AI algorithms are very good at distinguishing multifaceted patterns and relationships in images. For instance, they can identify lung nodules, fractures, or brain lesions faster and more accurately.
-
Example: CNNs, such as radiologists, are powerful in handling imaging data and replicating the diagnosis mechanism.
Reduction in False Positives and False Negatives
Failure to diagnose, worse in cancer, is a serious issue. AI minimizes the likelihood of misdiagnosing a disease (overdiagnosis) or completely missing it (underdiagnosis), enhancing the diagnosis's reliability.
-
Example: Computer-aided diagnosis in lung CT scans is highly accurate in differentiating between benign and malignant nodules, Automating Tax Preparation.
Automated Segmentation and Quantification
In medical images, AI quickly and easily divides the organs, tumours, or any other structure and their extent, size, volume, and position. This helps in following disease conditions over time.
-
In neurological imaging, AI systems learn to analyze MRI scan data to separate the brain into areas and perform a diagnosis of conditions such as Alzheimer’s disease or multiple sclerosis Code Assistants.
Faster Diagnosis with Real-Time Analysis
AI shortens the diagnostic time to seconds compared to manual diagnosis, where a doctor can examine several thousands of images. This is especially useful when quick decisions have to be made, such as during a stroke or trauma.
Recent Advancements in AI-Assisted Medical Imaging
Deep Learning Models
CNNs and transformers are some deep learning models that have made it easy for AI systems to process imaging data with high performance.
-
Vision Transformers (ViTs): The transformers are now integrated into the AI models to enhance medical image analysis and are intended to recognize minor variations.
Multi-Modal Imaging
AI merges patient data from various imaging modalities, such as CT scans and MRIs, to obtain holistic information about a patient’s status.
-
Example: PET-CT data in AI systems is a hypothesis that enhances cancer staging and treatment plans.
AI-Driven 3D Imaging
One of the most remarkable advancements of 3D medical imaging with the help of AI. New machine algorithms can improve the detection of tumours, fractures or internal injuries using 3D MRI and CT scans.
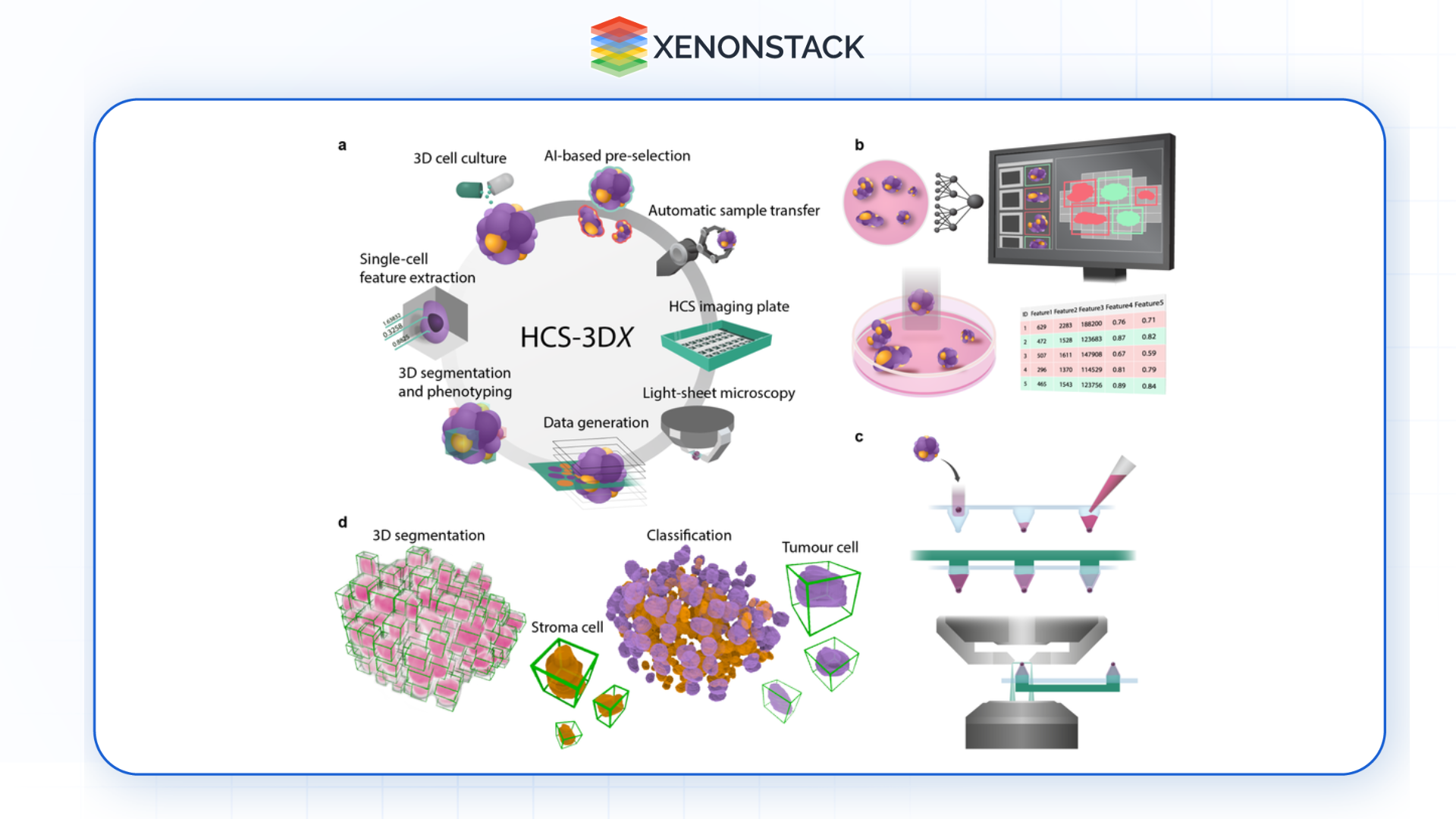
Edge AI for Point-of-Care Imaging
Edge AI enables medical imaging devices such as portable ultrasound machines to perform analyses locally using AI models, providing instantaneous results in areas lacking proper infrastructure.
-
Example: Real-time maternal care in rural clinics uses AI-assisted portable ultrasounds.
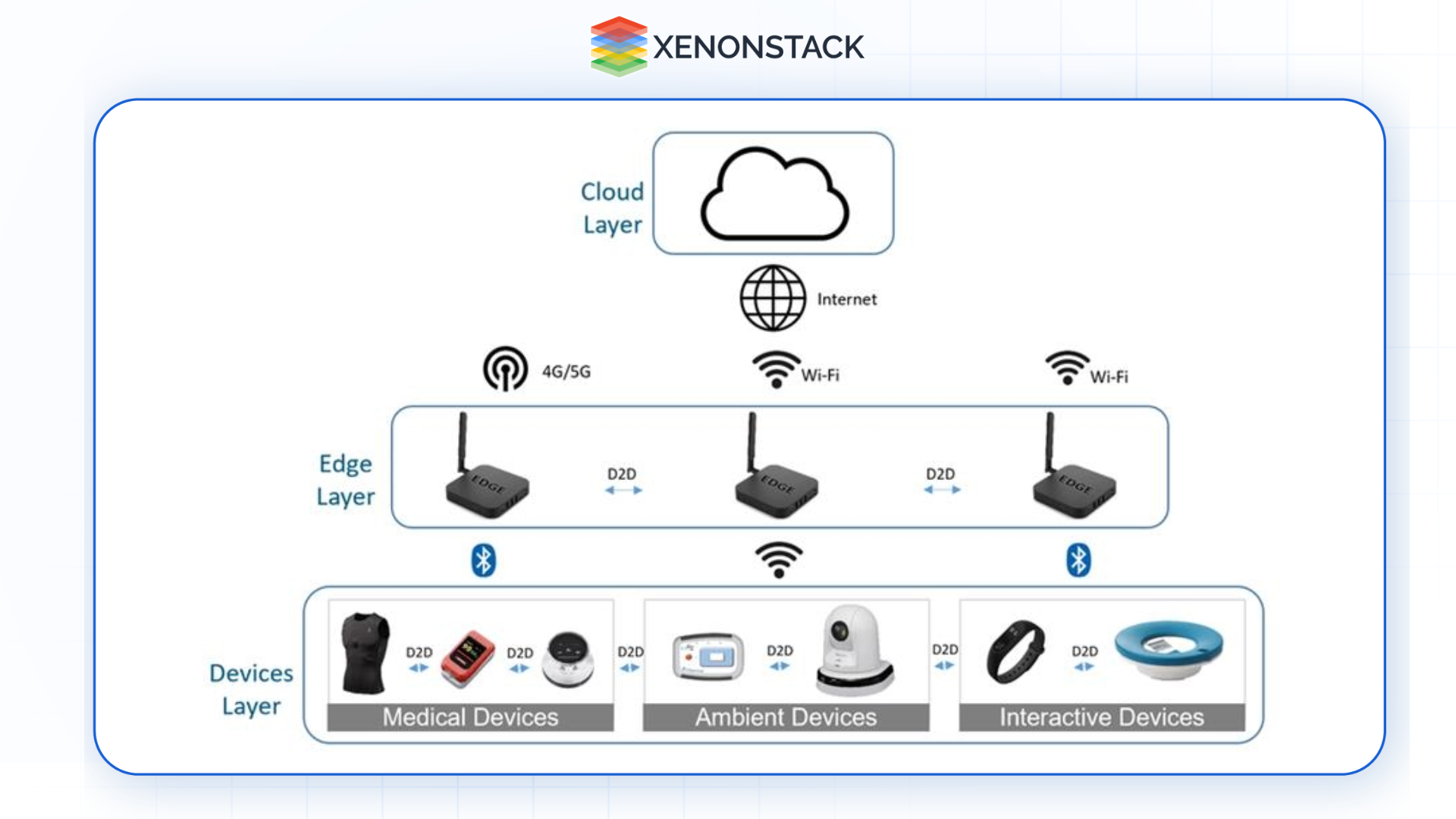
Real-Time Image Enhancement
Applications of deep learning systems enhance medical imaging from low-dose Computed Tomography scans. This makes it possible to minimize the radiation dose to patients who undergo CT or X-ray diagnosis and yet obtain accurate results.
Application of AI in Medical Imaging
-
Oncology: Cancer Detection and Monitoring
AI has advanced greatly in powering accurate cancer diagnosis by detecting tumours in mammograms, CT scans, and MRI scans.
-
Breast Cancer: Artificial intelligence patterns blaze through mammograms to identify breast lesions far earlier than other approaches. Computer programs like Google's DeepMind have proven more accurate than humans in breast cancer screening.
-
Lung Cancer: AI structures identify lung nodules in CT scans and differentiate between the benign and malignant forms with significant accuracy.
-
Neurology: Brain Imaging
AI-based imaging is revolutionizing how stroke, brain tumours, and Alzheimer’s disease are diagnosed.
-
Stroke Detection: Using AI to scan brain CT scans allows for determining ischemic strokes requiring faster treatment.
-
Neurodegenerative Disorders: AI models scan MRIs of brains to identify features for diagnosis of early Alzheimer’s, such as brain atrophy and volume loss.
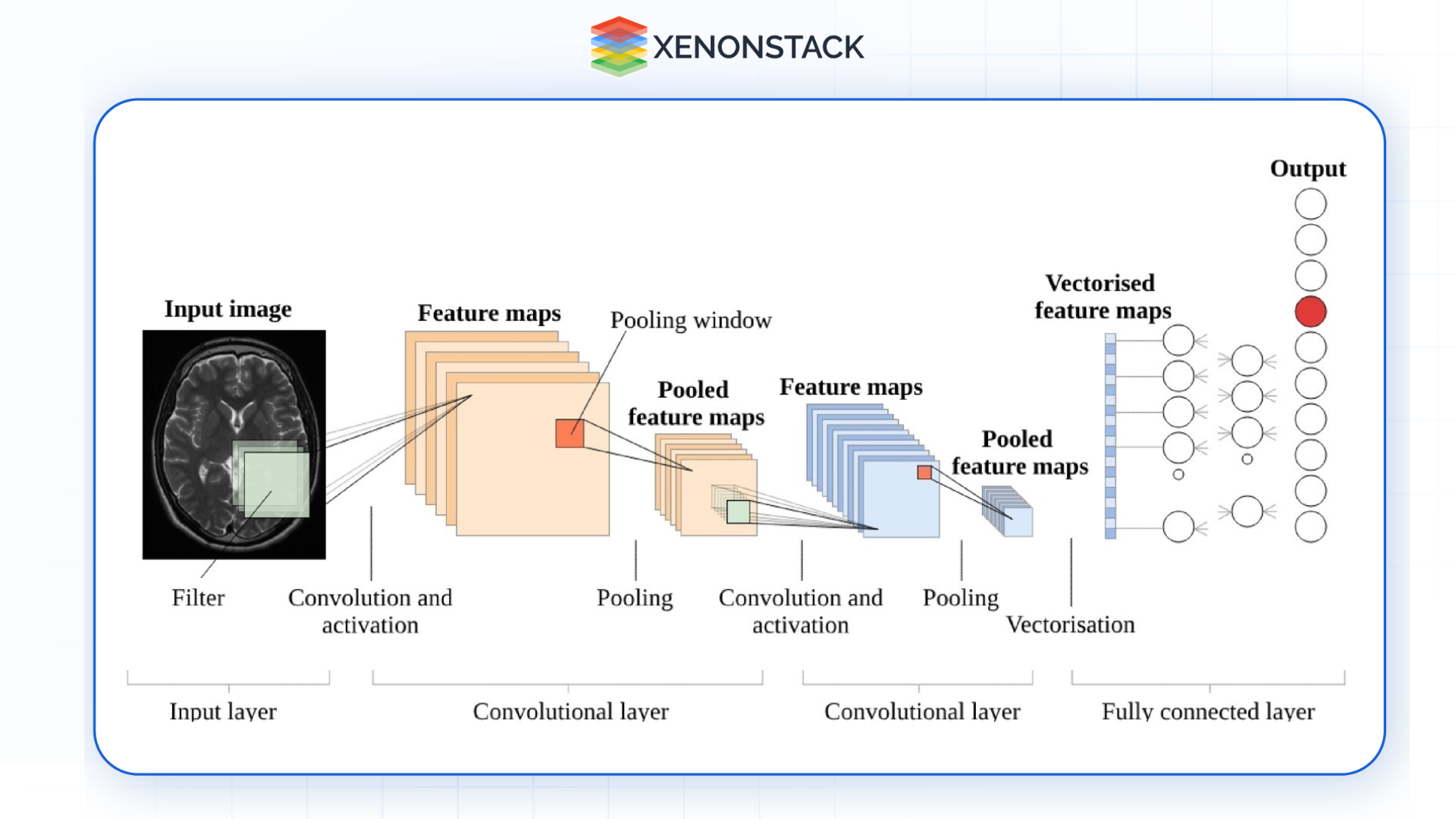
-
Cardiology: Heart Disease Diagnosis
AI assists cardiologists in evaluating echocardiographic images, cardiac MR images, and CT angiograms to identify heart diseases.
-
Example: From imaging data, AI tools diagnose heart valve defects, blockages and coronary artery disease for preventive health.
-
Orthopedics: Fracture and Joint Analysis
An example is the identification of fractured bones, dislocations and arthritic conditions such as arthritis from X-rays and CT scans.
-
Spinal Injuries: AI systems scan spinal MRI to determine if there is a herniated disc or a fracture in the vertebrae.
-
Ophthalmology: Eye Disease Screening
AI tools use retinal images to diagnose diseases, including diabetic retinopathy, glaucoma and age-related macular degeneration.
- Example: The top technologies on IDx-DR are imaging systems aided by artificial intelligence cleared by the FDA to diagnose diabetic retinopathy.
-
COVID-19 Detection
Machine learning techniques have been used to analyze chest X-rays and CT scans during the COVID-19 pandemic to identify infection and disease progression.
-
AI systems correctly detected ground-glass opacities of lungs and gave fast diagnoses in cases when it is vital.
Advantages of Artificial Intelligence Integrated Medical Imaging
-
Improved Diagnostic Accuracy
Some already know that AI can find small variations in patient data to improve the accuracy of diagnosis, leading to improved patients’ lives.
-
Less Workload on Radiologists
Some radiological procedures are cumbersome and time-consuming, but AI systems can manage them well, freeing many radiologists to concentrate on difficult cases and decisions.
-
Faster Diagnosis
AI helps speed up the process of reviewing the images, making it easier for clients to be treated when their condition requires emergency attention.
-
Cost-Effective Healthcare
Adopting AI leads to fewer misdiagnoses, better ways to organize work and, thus, less expenditure.
-
Accessibility to Remote Areas
Due to AI technologies, portable imaging devices cause an accurate diagnosis in areas without specialists.
Challenges in AI-Assisted Medical Imaging
-
Data Privacy and Security
Medical imaging data is private, and privacy is always challenging when using artificial neural networks.
-
Data Quality and Availability
The training of AI models needs large-quality datasets, which are generally scarce or of bad uniformity.
-
Concordance with Clinical Processes
Artificial intelligence technologies must be incorporated successfully into the hospital's operational environment without much hindrance.
-
Interpretability of AI Models
Radiologists and clinicians need to believe AI results. An attribution theory is required to explain how AI systems make their decisions.
The Future of AI-Assisted Medical Imaging
-
Personalized Medicine: This imaging data, along with patient history, genetic profiles, and biomarkers, are all key to diagnosis, and treatment will be delivered through AI.
-
Real-Time Monitoring: AI systems will track assessment images in real-time, improving the decision-making process in the clinical healthcare setting.
-
AI-Enhanced Robotics: AI will operate robotic systems to execute imaging-guided surgeries with high levels of accuracy.
-
Advanced Multi-Modal Imaging: The integration of combined multimodal imaging, e.g., using MRI with PET imaging with AI help, will also improve the understanding of disease diagnosis and prognosis.
-
AI-Driven Drug Development: AI models will analyze imaging data to detect disease biomarkers and speed up drug development and clinical trials.
With AI's help, medical imaging is being introduced to healthcare organizations as it increases the accuracy of diagnostics, lays less burden on employees, and enhances the speed of delivering treatment. From cancer diagnosis to neurological imaging and cardiology, AI has become almost mandatory for today’s medicine. However, experts have said that these are some of the barriers that continue to slow down the future of diagnostics through AI technology; some of the barriers include data privacy and data integration, among others.
Notwithstanding, these are some of the ground-breaking discoveries that have made diagnosis faster, more accurate, and even more accessible in the future through the application of artificial intelligence. As these computing changes persist, the application of AI in medical imaging will increase, enhancing health care and saving lives. By adopting this technology, the healthcare industry can be in a position to approximate the best standards of care for patients.
Next Steps with AI-Assisted Medical Imaging
Talk to our experts about implementing advanced AI systems and how industries and departments utilize Decision Intelligence to enhance outcomes. Leverage AI to automate and optimize medical imaging processes, improving accuracy, efficiency, and diagnostic responsiveness.