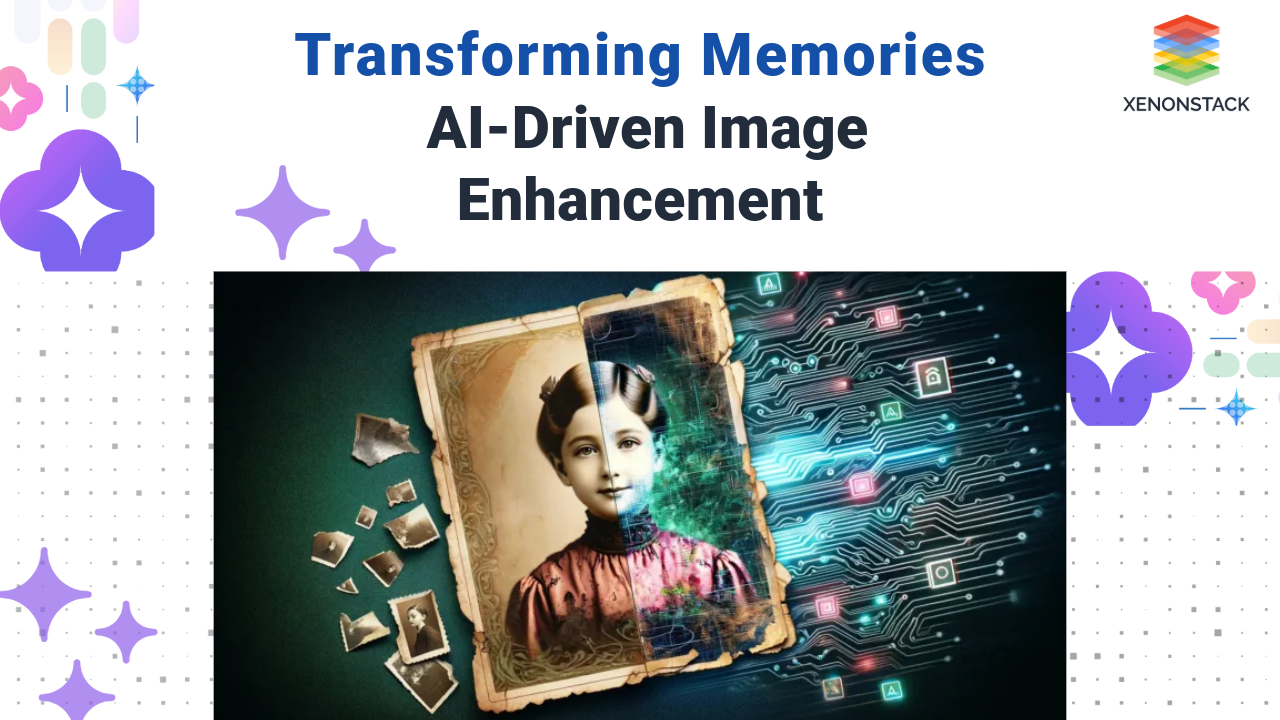
Understanding AI Image Restoration Techniques
Modern image restoration techniques differ rather drastically from traditional ones, with AI being incorporated into numerous solutions. AI also improves the value of restored images and transforms how we can view and collect the visual history of our archive.
Comparing Traditional and AI-Based Image Enhancement Methods
Traditional Image Restoration Methods
In the past, image restoration processes were more often performed manually, along with the help of quite simple algorithms. Common methods included:
-
Heuristic Filters: Filtering methods such as median and Gaussian filters were used to remove noise and convert the images to smooth ones. While helpful to some degree, these methods were not as efficient as previously thought since the image data were reduced to a minimally detailed outline.
-
Manual Retouching: Restoration specialists would spend hours, days and sometimes even years altering a single pixel needed in an image using tools such as Adobe Photoshop. Though this type of approach provides much control over the learning process, control was exerted at a high cost of time and effort, and the process remained rather subjective, leading to the unpredictability of results.
-
Frequency Domain Techniques: Specular reflectance scans were performed per image, while techniques like Fast Fourier Transforms were employed to isolate frequencies that could be boosted. Despite these attractive features, these techniques were computationally intensive and thus not easily portable for general use.
AI-Based Image Restoration Methods
On the contrary, the concept of AI-based restoration uses the features of increased machine learning and neural networks in the process of rendering restoration services. Key advantages include:
-
Deep Learning Models: Through conventional neural networks (CNNs), these kinds of AI models can identify distorted patterns and characteristics from image data sets with the help of large image data sets. This lets them restore deficiencies or boost enlarged photos to their correct quality and sharpness
-
Generative Adversarial Networks (GANs): These developments are arguably the most revolutionary in image restoration. Thus, when two computers, one creating an image and the other recognizing real and fake GANs, can create truly believable restorations that don’t lose the fundamental nature of the image.
-
Real-Time Processing: Instagram algorithms mean that AI can analyze images in real-time and give feedback and corrections instantly. This interactivity improves the users’ experience and also enables fast prototyping in restoration processes.
Harness the power of Generative Adversarial Networks (GANs) to revolutionize your Digital Marketing Strategies with personalized content and stunning visuals. Explore how GANs can elevate your marketing game
Key Components of AI Image Restoration Systems
This Blog aims to analyse the effectiveness of AI image restoration systems and thus discusses the architecture of the systems. Here are the key components that contribute to their success:
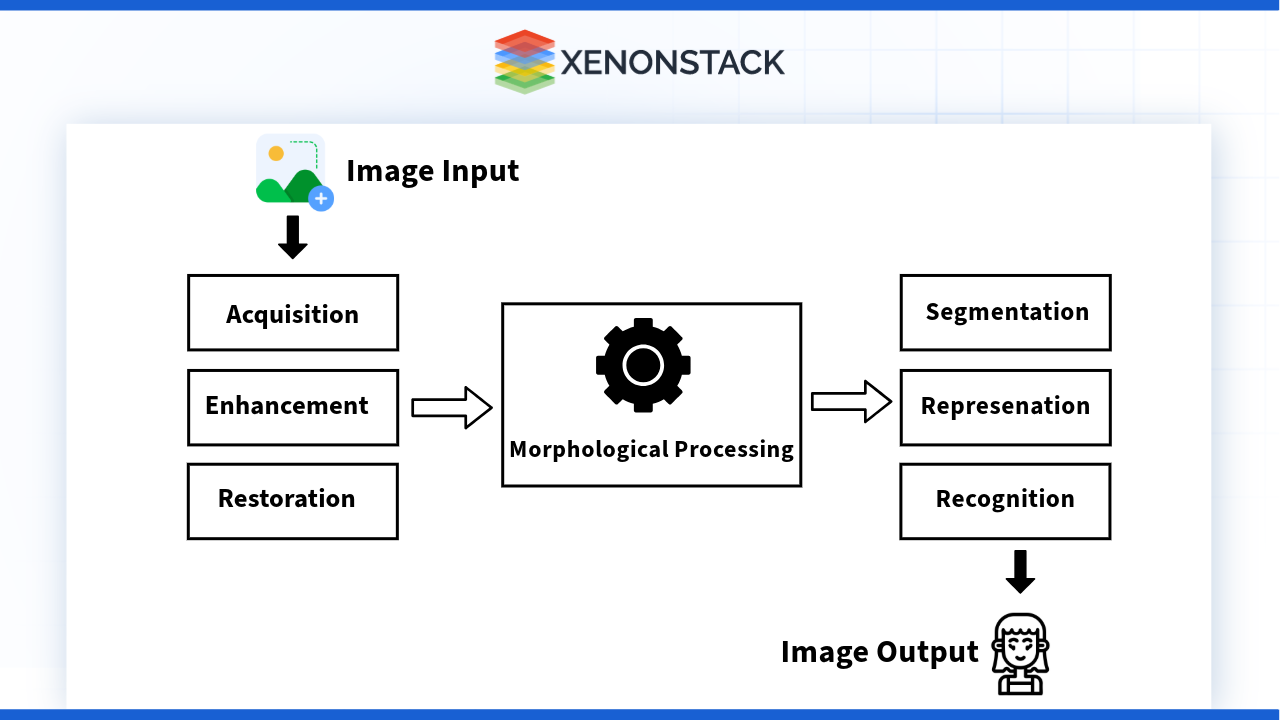
-
Data Acquisition and Preparation: AI is heavily dependent on data quality and data quantity in the formation of an operational base. Multiple types of images for various objects in low resolution and in the state where they were damaged or destroyed, together with undamaged versions of these objects, are essential for training dependable models. Features such as rotation, scaling and noise addition are some of the methods used to develop different sets of training data.
-
Deep Learning Architecture: When it comes to restoration, it has been seen that the architectural selection of neural networks can play a critically important role. Common architectures include:
-
Convolutional Neural Networks (CNNs): While two-dimensional images are excellent for spatial hierarchies, CNNs capture local features and pattern recognition.
-
U-Net: Particularly useful for image segmentation and restoration, U-net architecture enables it to locate specific details when working on more complex restorations.
Denoising and Deblurring Techniques for High-Quality Image Restoration
In image processing, two critical challenges persist: noise and blur. These artefacts cause image quality to sharp decline and blunt definition; important details are rendered as murkier images. With the development of artificial intelligence, more effective methods of noise and blur removal have been developed to help us improve the quality of our vision data. However, for a deeper description of the procedure and evaluation of the denoising techniques, the advanced deblurring algorithms, and the comparative study of these methods, which are beneficial in the current image restoration approaches, the next section is designed.
Denoising Techniques
From this definition, denoising refers to the process of removing noise from images, which may be a result of shot noise, quantum noise, low light conditions, degradations by the imaging sensors or errors during image transmission. The following are some prominent denoising techniques currently in use:
Spatial Domain Techniques:
-
Median Filtering: This non-linear technique replaces each pixel intensity with the median of its neighbours, which helps remove salt-and-pepper noise while preserving edge information.
-
Gaussian Filtering: This method applies a Gaussian kernel for smoothing, making it appropriate for images with loss of detail, particularly edges.
-
Wavelet Transform: This method breaks an image into many frequencies so that one can selectively remove the noises. It was found that by thresholding these wavelet coefficients, the noise can be removed while the useful details can still be preserved.
-
Fourier Transform: Like wavelet techniques, Fourier Transform analyses the frequency components. This method may also improve the signal-to-noise ratio since it reduces high-frequency noise, while mathematical morphology may not preserve spatial locality and wavelet methods.
-
Non-Local Means (NLM): To achieve a higher-quality image, this algorithm compares patches of pixels in the image, where similar patches are averaged to later enhance texture reduction of noise. Its strength is the use of non-local information, which makes the present system more efficient than the local filters.
-
Deep Learning Models: Currently, CNNs trained on large data sets have produced a great pronounced performance in denoising. Here, DnCNN and U-Net use learned features to de-noise.
Deblurring Algorithms
Deblurring is meant specifically to undo the impact of blur, which occurs due to the motion of the camera, the object, or due to the focal point. Here are some advanced algorithms designed for effective deblurring:
-
Blind Deconvolution: The procedural model presented in this technique provides the blur kernel and sharp image estimates in parallel, which is particularly important when the nature of the blur is unknown. However, blurred details can be recovered by using iterative optimisation techniques used in the blind deconvolution process.
-
Wiener Filtering: Considering prior knowledge of the signal and noise power spectra, Wiener filtering is a frequency-domain technique attempting to minimize the mean square error. Still, it is often effective and yields good results only if noise and blur characteristics are accurately estimated, which is not always possible.
-
Total Variation (TV) Regularization: This optimization method aims to minimize the total variation of an image, resulting in piece-wise constant areas while controlling noise. In deblurring, they pointed out that TV regularization effectively retains edge details, making it the most preferred method for high-quality image restoration.
-
Deep Learning-Based Deblurring: The new evolution of deep learning has led to an incredible state-of-the-art for deblurring models. For example, GANs or RNNs can learn complex blur patterns and give sharp outputs; often, these results are superior to the more traditional methods.
Comparative Analysis of Denoising and Deblurring Methods
It is important to gain a perspective on the techniques of denoising and deblurring and the various limitations that are inherent to them. Here’s a comparative analysis
Technique |
Advantages |
Limitations |
Median Filtering |
It is easy and efficient for S&P noise |
Blurs edges and fine details |
Gaussian Filtering |
Relatively simple to perform and practical to use. |
A lot of image blur and detail along the boundaries is lost |
Wavelet Transform |
Really good at being dense with information and sparse with noise |
Creates a lot of computational load; detailed tuning is needed |
Non-Local Means (NLM) |
Maintains texture and detail |
It takes a long time to execute, especially if you are working with large images. |
Blind Deconvolution |
Useful with unseen blur kernels |
May share slant slowly and create artefacts |
Total Variation Regularization |
It is good at preserving edges and slow at reducing noise. |
Can produce abrupt, flattened textures in frequently varying density regions |
Deep Learning Models |
Flexible and very efficient coined state-of-the-art result |
A complex design that needs large training sets and a great amount of computational power |
Real-World Applications of AI in Image Restoration
Reviving Historical Photos
Historically, we used historical photographs as sources, but most of them are in poor condition due to ageing. AI image restoration breathes new life into these treasures:
-
Repairing Damage: Another case is that AI algorithms are more effective than removing scratches, stains, and blotches and restoring the brightness and contrast of historical images. Advanced methods, such as inpainting, operate smartly to restore the absent portions of the artwork in a way that does not corrupt them.
-
Enhancing Details: AI tools increase the contrast and make the hitherto invisible parts of low-colored images apparent. This not only enhances the values of programmes but also helps the historian or researcher gain further insights about them.
-
Cultural Preservation: Using AI to digitize and restore these photos is an important way of helping to preserve history so that even future generations can see how special they are.
Enhancements in Photography
Today, owning smartphones or personal cameras has become easy for consumers to access. AI image restoration is transforming this landscape:
-
Automated Corrections: Advanced AI technologies in applications allow for correcting numerous problems without difficulty, improving picture quality regularly. Therefore, it is possible to achieve professional standards right from the comfort of one's own home.
-
Real-Time Enhancements: The AI algorithms, therefore, provide photographers with live image processing, which many photographers can edit as they take pictures, intending to get the perfect shot from the start.
-
Personalized Editing: AI learns user preferences and looks for manners in which to contribute to its user’s style, applying improvements to enhance the style but maintaining the ethos of the initial image.
Medical Imaging and Satellite Imagery
AI image restoration is critical in sectors where clarity is paramount:
-
Medical Imaging: analyzed, AI helps to improve signals in the images of a patient, for example, X-rays, MRIs, and others, through denoising and improving the contrast for radiologists to detect anomalies so that patient results are improved.
-
Satellite Imagery: In environmental monitoring and disaster response, AI restoration increases the clarity of satellite images. Pay attention to certain important parameters, such as resolution and atmospheric effects, which benefit policy-making.
-
Geospatial Analysis: AI helps to enhance the quality of geospatial images obtained in different conditions, which, in turn, improves operating decisions within the agricultural, forestry, and city-planning fields.
AI-based Video Analytics's primary goal is to detect temporal and spatial events in videos automatically. Click to explore our, AI-based Video Analytics
Ethical Considerations and Privacy in AI Image Restoration
AI image restoration features are also improving today in Photoshopping, which is amazing with positive and negative effects, such as the following. As soon as technologies are connected to personal stories, problems arise regarding the privacy of specific persons and legal issues of the techniques’ application. In this section, we’ll explore two key dimensions: The problems of data security, especially in image restoration companies and the ethical question of pixels and changes.
Data Privacy in Image Restoration Services
Privacy of these images is paramount as they tend to involve individuals’ parameters. Users often share cherished memories and sensitive information, so it's essential for service providers to prioritize privacy through the following measures:
-
User Consent: Organizations providing such services should clearly explain how the images will be used, stored, and erased, thus allowing users to decline being captured unnecessarily.
-
Data Security Measures: Ensure user-uploaded images do not fall prey to hackers. To this end, encryption and security audits shall be undertaken to protect the data both ‘in transit’ and at rest.
-
Image Retention Policies: Policies should be set that dictate how long the images are to be retained. Optimally, images should be erased soon after being processed; this makes the process more transparent and trustworthy.
-
Anonymization of Data: When using image data for training AI models, anonymize the data to protect individuals' identities and ensure sensitive information remains confidential.
-
Compliance with Regulations: Adhere to privacy regulations like GDPR and CCPA to safeguard user rights and bolster your service's credibility.
Ethical Implications of Image Manipulation
While AI image restoration enhances visual content, it also raises pressing ethical questions:
-
Authenticity and Trust: Large changes can obliterate the border between the real world and illusion. This is an important requirement that needs to be given to society because people have to believe that the pictures they see are genuine memory sequences, particularly in journalist or historical journalism.
-
Misleading Representations: Restored images can be bad representations and give viewers a wrong perception of the original images. To avoid ambiguity, clear labelling is required in this research.
-
Cultural Sensitivity: Restoration practices should be exercised cautiously within cultures, underestimating the culture’s stories and thereby disregarding their own meanings.
Future Trends Shaping AI-Powered Image Enhancement Technologies
AI image restoration opens a broad list and field of possibilities that can be reached in the future. Here are some key trends to watch:
-
Augmented Reality Integration: AI image restoration will integrate with augmented reality (AR), boosting live images and videos in real-time. This advancement will revolutionise everything from the game perspective for users to the perspective of learning and the like.
-
Advanced Algorithms: The future development of deep learning will create more advanced algorithms to solve various restoration problems and provide greater image quality even under adverse environmental conditions.
-
Personalization: Future tools will be better at customization since they will permit users to select the type of restoration they want to receive. There will be behaviour patterns to which machine learning shall suggest tailor-made improvements.
-
Cross-Domain Applications: Approaches from AI image restoration are proposed to be applied to video restoration, virtual reality, and audiovisual media.
-
Ethical Standards: With capabilities, there will be an increasing concern with ethical norms and reporting. From the enhancement process, the providers will still be at risk of violating user rights as they carry out image processing, which will require some measures to balance the two.
Key Takeaways on AI Image Restoration Advancements
AI image restoration is a fascinating intersection of engineering and art, which puts effective instruments for editing and archiving images in one's hands. Therefore, as we advance, industry players must consider the ethical aspects that accompany the prospects of using AI technology in image restoration while considering user privacy.
The future is ahead, and there are great expectations as far as the development of the next imaging devices and their features are concerned: both issues that can enhance the quality of the obtained images and can extend the possibilities of interpreting the overall image of the world we see. It identified that, through responsible innovation, AI image restoration can extend the ability to make a positive social impact and do more than fill vital secular roles in safeguarding and deepening our cultural connections.