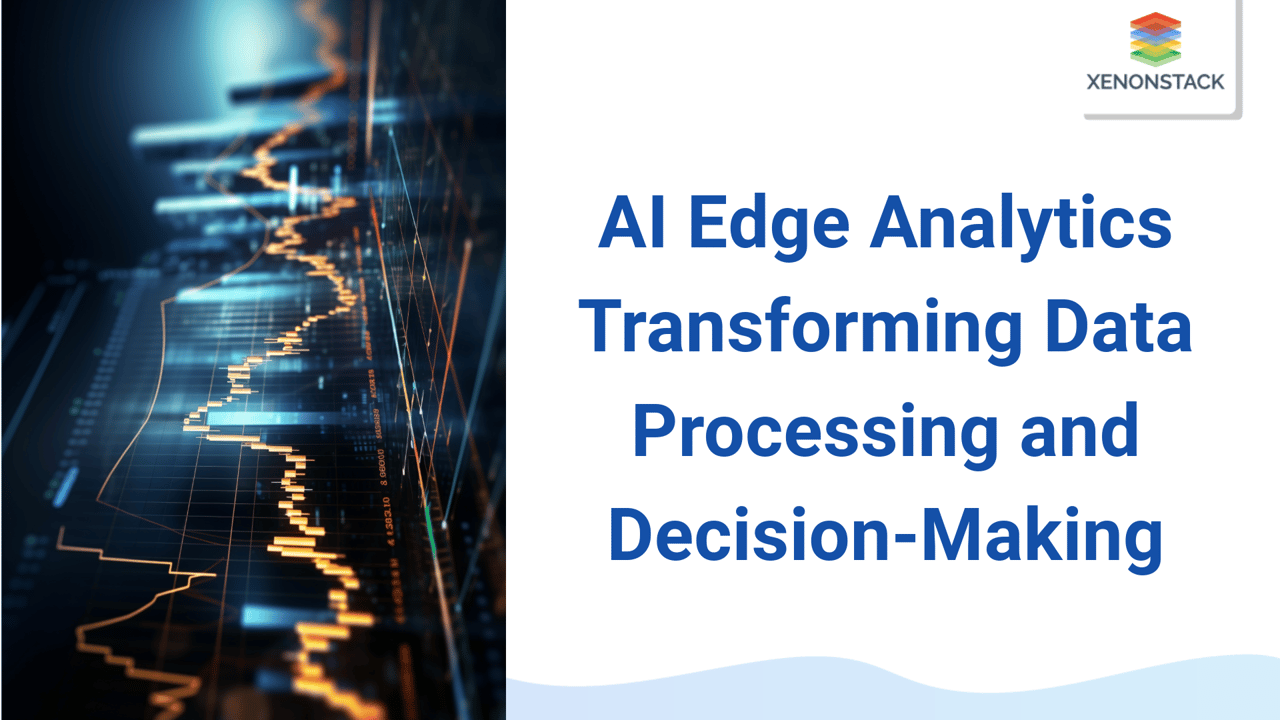
Today’s business world relies on instant data processing and analysis like never before due to increasing growth in digital technology. Companies and organizations receive huge volumes of data from many devices and sensors, including the IoT. Therefore, conventional approaches to data processing using the cloud are facing various drawbacks, such as delays, bandwidth constraints, and security vulnerabilities. Enter AI-powered edge analytics, where volumes are processed at the network's edge. By pushing data processing closer to the sources of this data, that is, closer to the ‘edge’ of the network, this novel approach improves the on-the-fly capability of decision-making and organizational functioning.
Edge analytics involves using artificial intelligence to analyze big data on the edge and helps organizations gather the information they need without having to send that information to centralized servers. In the subsequent sections, as we proceed further into this topic, we will also discuss what edge analytics has been used for, the present use of edge analytics, the issues that edge analytics is exposed to, and what edge analytics could be used for in the future.
Early Edge Analytics Approaches
The Foundation of Data Processing
This data processing process started with centralized operation, mainly using centralized data centres and mainframes. First-generation computing systems assumed that a single PU could collect data for processing, an approach that commonly demanded much physical infrastructure. Information was gathered from various sources, sent to data hubs, and analyzed before the information was obtained. This approach was quite suitable in the early years of computation, but as the amount of data increased and the requirement for more periodical results was set in the model, it started to show initial signs of weakness.
Fig 1: AI-Powered Edge Analytics
Emergence of Edge Computing
Originally, edge computing emerged during the early 2000s as a solution to the problems of centralized computation. It is the technique of computation and analysis of data on the network's boundary rather than centralized in a data centre. This shift allowed organizations to address several key challenges associated with traditional data processing:
-
Latency: With the advent of IoT, much information, especially data, was produced in real-time. When one had to analyze data and get insights about something, the delay due to data transmission to a central server became out of the question.
-
Bandwidth Constraints: It pointed to tremendous inherent pressure on the network's bandwidth due to the exponential increase in data generated by connected devices. All data is sent to the cloud for processing, and the approach didn’t make much sense regarding cost optimization or efficiency.
-
Security and Privacy Concerns: Sending confidential information to the cloud can cause data leakage and require specific legislation, health care, or finance.
Early solutions that involved a simple data filter and pre-processing approaching the edge answered straightforward questions in the first case. Companies have started adopting local computing capabilities for initial processing to filter the data that should be sent to the cloud. This was a starting point for shifting from centralized processing to edge computing and additional analytics.
Progress and Innovations Over Time
Over the years, the capabilities, ranging from the prospects of edge analytics, have continued to expand with advanced technology. Several factors have contributed to the advancement of AI-powered edge analytics:
Increased Computational Power
In today's world, high-performance edge devices that process data locally have marvellous processors, GPUs, and special hardware. These devices are now powerful enough to execute many complex mathematical calculations and very advanced AI algorithms in real-time. Therefore, edge analytics can handle billions of bits of data within a short period, which makes decision-making effective.
AI and ML as a Service
Another major development is the connection of artificial intelligence and machine learning algorithms to the edge analyzer on the edge gadgets. These algorithms allow devices to analyse data, learn from past data records and make predictions or decisions in real-time. By utilizing AI at the edge, organizations can:
-
Detect Anomalies: It’s much easier for an AI to look for irregularities and outliers in the trend that can be redressed before they become problematic.
Fig 2: Optimize Performance
-
Optimize Performance: The artificial neural network models prove to be capable of learning right from the new data it receives over time.
-
Personalize Experiences: Businesses can personalize services and products offered in a certain locality by analyzing customer data in real time.
Improved Connectivity
Edge analytics has been significantly enhanced with the help of high-speed connectivity technologies, including 5G, to mention but a few. This connection enhancement assists in transmitting, monitoring, and responding to data in real-time, which are all vital for edge analysis.
Fig 3: Improved Connectivity
Edge Computing Frameworks
Lately, some solid frameworks and platforms have begun to be developed for edge computing to deploy AI models at the edge. These frameworks empower developers with the right solutions, properties, and strategies to develop, maintain and deploy edge analytics solutions effectively. IoT cloud platforms with the scale of edge computing, including AWS IoT Greengrass, Microsoft Azure IoT Edge, Google Cloud IoT Edge, etc., enable organizations to extend AI and analytics capability to edge devices.
Expansion of Use Cases
When the idea of edge analytics started, the solutions were cruder and had fewer use cases, but with advancements in technology, many applications can now be found around it. Examples of areas using edge analytics are manufacturing, healthcare, transportation, and smart cities to foster breakthroughs and optimize their functioning.
Impact of AI-Driven Edge Analytics
Edge analytics using artificial intelligence is revolutionizing industries by allowing companies to benefit fully from the data. The impacts are multifaceted:
-
Real-time Decision Making
Real-time decision-making is one of the important benefits of edge analytics enabled by artificial intelligence. This means that organizations can address changes and irregularities as they occur to enhance the effectiveness of operations and customer experience. For example, in the manufacturing industry, edge analytics in predictive maintenance will help in the early detection of equipment likely to develop a fault in future, thus minimizing time wastage and monetary loss. -
Cost Savings
That means that bandwidth costs, as well as the costs of cloud storage, can be reduced if organizations reduce the amount of data transmitted to the cloud. By processing data locally, small data transfers are required, and they can be done effectively, which, when compounded over a period, saves a great deal of money. However, edge analytics can improve the utilization of resources for better efficiency in operations. -
Enhanced Security
Storing such data at the edge reduces the risk of a breach during data transfer between the various layers. This is because organizations can tackle security issues at the edge, thus minimizing exposures from transferring data to central servers. However, the data to be transmitted can be anonymous or grouped together, meaning that privacy and data security are further improved. -
Improved Reliability
Because edge analysis is a standalone process, it stays active even when the internet is unavailable or intentionally disconnected in parts of the world. This reliability is more important, especially for applications in agriculture and health, where real-time surveillance and control are inevitable. -
Increased Customer Satisfaction
AI-based edge analytics makes real-time data analysis possible, enabling organizations to create positive customer experiences. By embracing big data analytics techniques, companies can predict changes in customer requirements and purchasing habits and, in turn, provide an individualistic solution, improving customer satisfaction and loyalty.
Real-World Applications
-
Manufacturing: AI is changing the production environment in manufacturing through edge analytics. Predictive maintenance systems supervise machinery conditions, analyze performance parameters, and predict failure. This action reduces maintenance time and the overall cost of maintenance and makes the machines work efficiently.
-
Healthcare: This is the case despite the revolutionary advancement of innovative healthcare technologies that embrace edge analytics. Smart garments and telemonitoring platforms quantitatively measure physiological quantities and changes in clients’ status and signal for urgent response in case of necessity. This can allow emergency cases and specific treatments to occur on time.
-
Smart Cities: AI edge analytics is a top emerging category in smart city projects. Transportation management systems use various edge devices to analyze data from various sensors in real-time to improve traffic movement, minimize traffic jams, and increase the overall safety of the individuals using the transport facilities. Likewise, environmental monitoring systems can process data concerning air quality to provide city planners with useful recommendations.
-
Retail: To weaken the pressure on traditional centralized power infrastructures and the ecosystem glut, retailers use edge analytics to transform shopping experiences and stock prized possessions. Real-time analysis of customers, their buying patterns, and their choices will help the business produce more effective promotional campaigns and recommendations that are more likely to delight customers and increase business activity.
-
Agriculture: In precision agriculture, predictive models are making a difference at the edge. Devices in the fields can capture information regarding soil status, climate, and plant conditions. When such data are analyzed at the local level, farmers can determine how best to irrigate their crops, apply fertilizers, or use pesticides to increase yields without using many resources.
Key Challenges and Solutions
As is true with most innovative technologies, edge analytics based on artificial intelligence also has issues that must be solved to reach its full potential.
-
Scalability
At the centre of this challenge is that as the number of connected devices rises, scaling edge analytics solutions can become quite difficult. The enterprise needs to deal with the rising number of devices with which it is integrated and with the flow of data that needs to be processed by those devices.
Solution: Modular Architectures
Scalability problems can be prevented by establishing flexible module infrastructures that support adding new devices. An organization can incorporate the features of flexibility and scalability that allow for integrating new devices into the whole network without major readjustments. This can allow businesses to be more like current needs and firsts in technology that can be helpful. -
Data Management
Collecting, processing, and storing data requires proper handling of the lifecycle process, and it becomes even more difficult to focus on edge devices. Data quality, consistency, and governance are some of the factors most needed to be right regarding edge analytics.
Solution: Automated Data Management Tools
This is when automated data governance and management tools can effectively ease this process. These tools can set up standards for data quality, compliance checking, and automation of various connected tasks. Thus, organizations can achieve more reliable and accurate edge analytics solutions by following strict data management practices. -
Integration with Legacy Systems
As we will discuss, some current edge analytics solutions have issues that may not support many organizations’ existing legacy systems. Integration can, however, be very challenging when new edge technologies are introduced into these systems.
Solution: APIs and Middleware
Unfortunately, there is a significant gap between legacy systems and new solutions based on edge analytics here. Again, developing APIs and middleware can help link these two. In this process, organizations may reuse their existing structural investments, help introduce new technologies, and integrate systems with different formats and data structures. -
Security Risks
Edge computing can improve security, but the concept also brings new security risks, specifically to the edge. Gadgets on the edge can be targets of cyberattacks, which can harm the data accumulated.
Solution: Robust Security Protocols
It is critical to implement strong security measures to enhance security on edge devices, including, but not limited to, encryption, access controls, and device authentication. Security professionals must focus on adopting a defence-in-depth security model to secure an organization’s edge analytics infrastructure. -
Skill Gaps
A fast-growing interest in edge analytics technologies has led to the need to find qualified specialists to develop and manage such solutions. However, talent scarcity in this area makes deployment a challenge for industries.
Solution: Training and Development Programs
Top management can, therefore, take advantage of training and development activities to train their employees in the new technologies and also attract new talents. Negotiations with educational institutions where new programs are to be developed to address the market's needs in the sphere of edge analytics will also contribute to the company’s staffing in the future.
Emerging Trends in AI Edge Analytics
As technology evolves, several trends are emerging in the realm of AI-powered edge analytics:
-
Federated Learning
Federated learning is the process of performing model updates at the edges where data are collected without transferring actual data to other devices. Instead of uploading the data to a central server for training, the devices engage in collaborative learning with data remaining on the device. This improves privacy and does not require data movement, making it most suitable for medical or banking sectors. -
Edge AI for Autonomous Systems
Current applications of edge AI include autonomous transport through self-driving cars, drones, and robots. These systems call for real-time as they are expected to make decisions on behalf of a user based on signals they receive from the cloud but without needing further cloud support. For instance, self-driving cars employ data coming from the onboard sensors and cameras to operate in real mode. -
Integration with Blockchain
They pointed out that the integration of edge analytics and blockchain can improve data protection and data accuracy. As such, the concept of blockchain can be used by organizations to develop and implement systems that will receive data from edge devices in a decentralized manner, making sure that it is secure and unaltered. This is especially true in industries like finance, supply chain and health, where accuracy when dealing with data is highly important. -
Self-Healing Networks
Current work also needs to be done to develop AI algorithms that allow edge networks to self-recognize and self-heal when failures occur. It has the ability to locate a problem, route traffic, and work around it so that services continue without interruption. This capability is particularly important for applications that can have a material impact on business outcomes, particularly in industries that operate in the telecommunications and energy sectors.
-
Enhanced Privacy Regulations
Increasingly, organizations will need to follow the rules of data privacy legislation as edge analytics becomes more popular. In this context, organizations must address large numbers of legal peculiarities to process the data, adhering to the requirements of both the local legislation and the legislation of other countries. Relatively, future directions show that there will be an increased focus on privacy considerations by design principle for edge analytical solutions with compliance mechanisms included in the tools for handling the data. -
Low Code and No Code Development
New platforms allow the introduction of edge analytics by utilizing low-code and no-code solutions and approaches. These platforms allow ordinary users with little programming experience to design and implement edge analytics applications. Consequently, it is easier for enterprises to adopt edge analytics features as they help cut the time companies need to develop them.
Features
|
Low Code
|
No Code
|
Primary Server |
Developers |
Business Users |
Primarily Objective |
Speed of Development |
Ease of Use |
Coding Need |
Low but Present |
No coding Required |
Customization |
Total Customization Available |
Pre-built Templates can be Customized |
End-to-End Development |
All Platforms Provide End-to-End Development |
Some platforms provide only limited Capabilities |
APP Complexity |
Can create complex apps |
Can create simple apps |
Purpose |
Next-Gen Rapid application development tools for professional developers |
Self-service application for business users |
What’s Next for AI in Edge Analytics
The future of AI-powered edge analytics is promising, with numerous developments on the horizon that will shape its evolution:
-
Widespread Adoption Across Industries
Additional industries, including agriculture, retail, logistics, and telecoms, shall adopt edge analytics to enhance operational productivity and customer experience. As more companies realize the value of near real-time message processing, edge analytics will only increase and become the norm in their organizations. -
Enhanced Interoperability
New developments in edge analytics will probably include integration with the coordinated and in conjunction approach in which multiple devices and systems interact. Standardized protocols and frameworks will help other vendors and technology stack up to create orderly edge ecosystems. -
Greater Emphasis on Sustainability
As more organizations become environmentally conscious, they will look for edges that can operate with low energy consumption and, thereby, low emissions. Systems on the edge will engage in sustainability where feasible, as we shall see them using renewable energy to power the devices and having mechanisms drawn from the earlier formats that govern resource usage that align with CSR. -
Evolution of Regulatory Frameworks
Over time, regulatory agencies will emerge to provide policy on ownership, privacy, and data security at the edge from the increasing adoption of edge analytics. Another risk is that organizations will need to continue their update of changes in regulations and incorporate them into edge analytics solutions. -
AI Democratization
Thus, they conclude that the democratization of AI is likely to remain the key determinant of edge analytics’ evolution. With this advancement in artificial intelligence and the acquisition of mass, people will normally be able to use edge analytics in AI at the business level. This trend befitting the small organization will put it on a level ground with other large enterprises. -
Quantum Computing Integration
Still in its early stages, quantum computing is set to massively disrupt the edge analytics market. The possibility of working with a huge amount of information at a speed that has not been possible before can open new opportunities for analytics and various decision-making processes at the edge. While discussing the trends, one can imagine that the advancement in quantum technology might bring the technological shift to edge analytics solutions in industries and applications.
Final Insights
AI at the edges represents a shift from current data analysis models for organizations. By deploying analytics closer to where data is being created, organizations effectively cut through time and bureaucracy, pave the way to informed decisions, and save costs while bolstering security. These positive developments regarding computation, AI, connectivity, and frameworks have ensured that edge analytics now provides the desired benefits that were originally not available while handling large amounts of data.
Nevertheless, this field will soon encounter some obstacles; however, recent trends in the technology world and the opportunities provided by the development of AI confirm the perspectives of success for edge analytics. While industries try to come up with more uses for AI at the edge, one surely must admit that this technology, which pushes computing and intelligence to the physical edge where data is generated, will change the world as it optimizes it and opens more opportunities.
Read More Edge AI vs Federated Learning