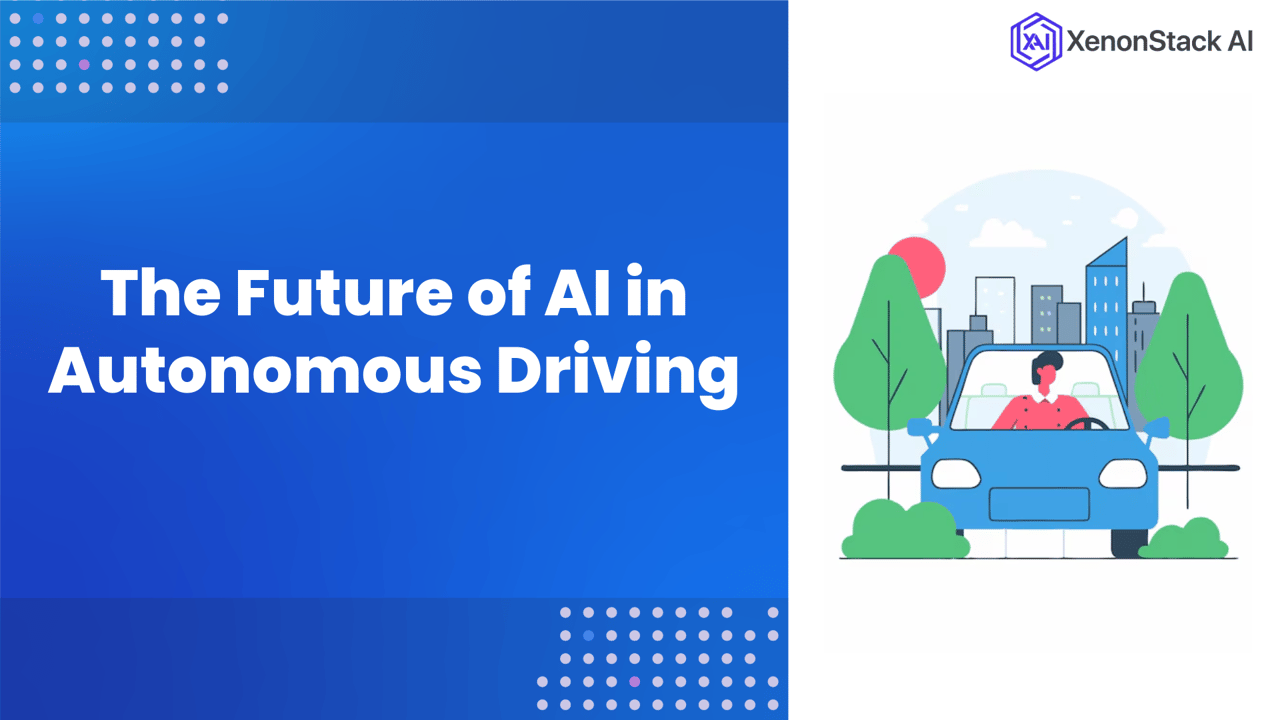
Autonomous vehicles (AVs) represent a revolutionary advancement in transportation, offering the potential for enhanced safety, efficiency, and convenience. Artificial intelligence (AI) is central to this transformation, which enables vehicles to perceive their surroundings, make decisions, and navigate complex traffic scenarios without human intervention. However, despite significant progress, AI-driven autonomous vehicles still face numerous safety and regulatory challenges that must be addressed to ensure public trust, safety, and large-scale adoption.
This blog delves into these challenges, explores the technologies behind autonomous vehicles, and highlights recent advancements in this rapidly evolving field.
The Role of AI in Autonomous Vehicles
Autonomous vehicles utilize artificial intelligence as their cognitive core to detect real-world settings by processing sensor, camera, radar, LiDAR, and machine learning algorithms data. These systems maintain ethical driving operations to achieve safety alongside control effectiveness.
Figure 1: Ethics of autonomous driving
Several safety issues continue to impede the widespread use of AI-driven autonomous vehicles until solutions can be found to resolve them.
Safety and Regulatory Challenges
The deployment of AI in autonomous vehicles introduces several safety and regulatory hurdles:
-
Perception Errors: AI systems have two fundamental requirements for sensing operations: sensors and machine learning algorithms for interpreting data. Data interpretation errors result in the wrong decisions made by these systems. These systems have demonstrated the ability to mistake a stop sign for a 45-mph speed limit sign under certain specific environmental conditions—IT Infrastructure Management with Autonomous Operations.
-
Ethical Decision-Making: Autonomous vehicles must make moral decisions regarding unavoidable accidents because they face dilemmas between adverse consequences when a collision becomes imminent. Embedding ethical decision capabilities inside AI systems is a highly intricate matter.
-
Regulatory Inconsistencies: Autonomous vehicles face regulatory challenges since different jurisdictions create inconsistent safety standards that oppose each other during approval procedures. More than 80 separate legislations about autonomous cars were passed by U.S. state governments, which established their unique regulations.
-
Public Trust: Public Trust refers to establishing trust with the general population about autonomous vehicles. Public concerns about self-driving vehicles increased after prominent accidents made it clear that safety assessments must align with clear industry communications. Autonomous Operations for Retail Industry.
Recent Advancements in the AV Industry
Despite these challenges, significant progress has been made:
-
Tesla's Unsupervised Driving Initiative: Tesla's Unsupervised Driving Initiative aims to deploy robotaxis through its service in Austin, Texas, starting June 2025. The service will arrive with a fleet of Tesla-owned vehicles that deliver driverless rides to customers through automated systems. Despite technical limitations and safety worries, Tesla demonstrated its sustained dedication to AI research in autonomous driving. Autonomous Operations in transforming Banking.
-
Waymo's Expansion: Waymo plans to conduct autonomous driving technology tests in ten new cities across 2025, including San Diego and Las Vegas. The expansion enables AI systems to become operational in various urban environments, thus improving the safety capabilities of their autonomous driving solution.
Core Technologies using AI in Autonomous vehicles
Several state-of-the-art technologies have merged into autonomous vehicles, delivering efficient performance and safety operations. The following part examines the fundamental technological components that support autonomous driving platforms.
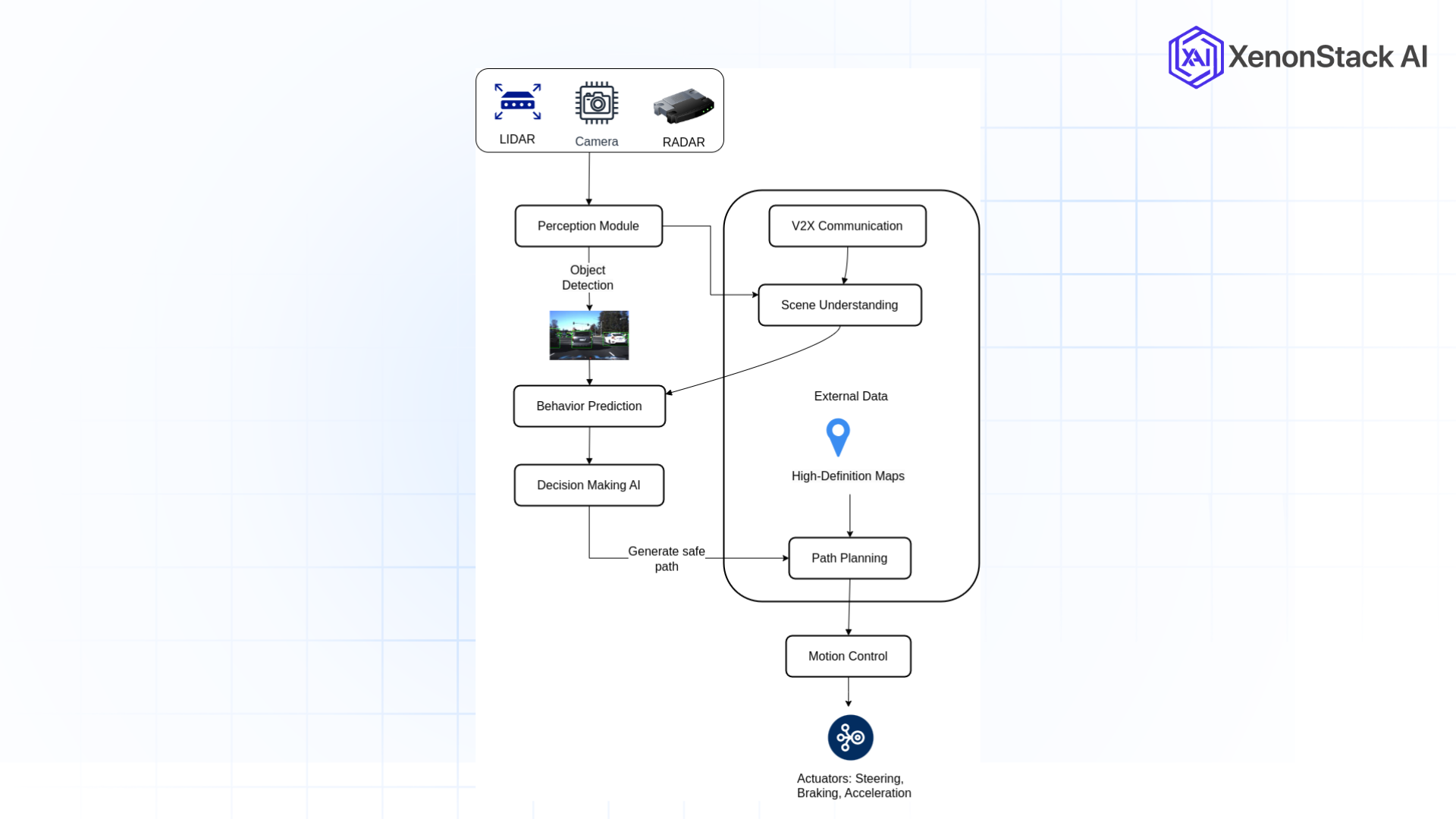
-
Sensor Systems and AI Integration
AI algorithms analyze the vehicle's surroundings, processing sensor data from various components to generate an entire view of the environment.
-
LiDAR (Light Detection and Ranging): A LiDAR system uses its generated 3D point clouds to model objects, which helps find their shapes, determine sizes and evaluate potential safety risks. The data obtained from sensors undergoes machine learning processing, which develops capabilities to identify pedestrians, cyclists, and other vehicles.
-
Cameras: Image-processing software operates through cameras to perform object recognition and sign reading operations with lane marker surveillance capabilities. Through deep learning, the vehicle system obtains enhanced capabilities to understand elaborate visual inputs, including predicting pedestrian intentions through body signal reading.
-
Radar: Computing systems analyze radar data through algorithms that determine nearby objects' movements and speeds. By combining radar data with additional sensor information, the vehicle achieves better decision-making in changing environments.
-
Perception and Localization through AI
The core function of AI is to help AVs understand their surroundings while establishing their precise position.
-
Simultaneous Localization and Mapping (SLAM): Through AI algorithms, SLAM technology enables the processing of sensor information to create real-time maps for unknown surroundings, during which it also maintains vehicle position logs. Secure navigation depends heavily on this capability as it helps robots manage unreliable GPS signals.
- Decision-Making and Control
Machine learning algorithms are central to the decision-making processes that govern vehicle behaviour.
-
Path Planning: Through path planning, algorithms analyze multiple alternative routes to establish the safest and practice-oriented routes by factoring in traffic situations and lane topology and following technical rules. Vehicles' path-planning strategies become more effective as reinforcement learning systems allow them to learn from their experiences.
-
Control Systems: Automated systems implement the smooth and safe operation of control systems to execute driving manoeuvres through the vehicle. The combination of real-time sensor information enables AI algorithms to control steering, acceleration, and braking, thus achieving safe driving distances while maintaining the correct lane position.
- Connectivity and AI
AI provides vehicles with advanced interconnectivity options, resulting in better decision quality.
-
Vehicle-to-Everything (V2X) Communication: V2X Communication acts through AI by processing information from other vehicles and infrastructure to predict moving traffic conditions. Through V2X communication, the system obtains traffic signal information, enabling it to modify its vehicle speed accordingly.
Emerging Technologies in Autonomous Vehicles
Recent advancements in AI are further propelling the capabilities of AVs.
-
End-to-end Learning: Tesla and other companies are researching end-to-end machine learning systems, which enable models to use raw sensor data to generate driving actions. Experts seek to reduce decision complexity through this method, even though it makes safety testing and understanding system decisions more difficult.
-
Multimodal AI Models: Waymo is investigating using multimodal AI models from Google's Gemini to explore advanced sensors and enhance vehicle command decisions in complex driving conditions. The models improve vehicles' ability to understand different environments around them.
-
Edge AI: Implementing AI models inside vehicles forms what we characterize as Edge AI for real-time data analysis while decreasing response time. This method remains essential for safety-critical applications since it enables fast responses to changes in driving situations.
Real-World Applications of Autonomous Vehicles
Autonomous vehicles are being utilized in various practical applications:
Ride-Hailing Services: Companies like Waymo and Tesla are establishing autonomous transportation solutions for ride-hailing operations that provide driverless passengers with an easy and efficient driving experience.
Logistics and Delivery: The supply chain benefits from autonomous trucks and delivery vehicles being tested for goods transport as they reduce operational expenses and enhance delivery efficiency.
Public Transportation: Some cities are exploring autonomous buses and shuttles to enhance public transit systems, offering flexible and on-demand services.
Personal Mobility Devices: The development of autonomous scooters and bikes are becoming available for personal use to enhance urban transit systems.
Mining and Agriculture: Self-driving vehicles are now active in mining and agricultural operations, helping organizations achieve operational efficiency and safety standards under challenging settings.
Advantages of AI-Driven Transportation
The adoption of autonomous vehicles offers several potential benefits:
-
Improved Safety: AVs can decrease road accidents by overcoming human mistakes that trigger most traffic accidents.
-
Increased Efficiency: The autonomous driving system enables vehicles to enhance driving patterns, reducing congestion and improving efficiency measures.
-
Enhanced Mobility: Autonomous vehicles ensure transportation access to people who cannot drive through their adult system, which provides mobility benefits to disabled individuals and elderly citizens.
-
Environmental Benefits: Autonomous vehicles achieve ecological benefits as they optimize routes. This reduces fuel consumption and helps the environment by lowering carbon emissions.
-
Cost Reduction for Businesses: AVs reduce operational expenses, particularly in the delivery and public transit sectors and logistics operations, which lowers costs for all parties.
Future Outlook for Autonomous Driving
The future of autonomous driving is poised to witness several trends:
-
Advancements in AI: Research in Artificial Intelligence and machine learning will allow scientists to develop more advanced algorithms to handle challenging driving situations and improve decision processes.
-
Integration of V2X Communication: Autonomous vehicles using V2X communication will establish real-time interaction with street infrastructure, vehicles, and pedestrians, thus improving overall system visibility for better safety.
-
Regulatory Developments: Regulatory bodies will create more defined rules and deployment standards once AV technology reaches stability.
-
Adoption in Smart Cities: Autonomous vehicles will substantially enhance innovative city development, as they can connect to intelligent infrastructure to create improved transportation systems and lower urban congestion.
-
Advanced Human-Machine Interaction: Better human-machine interaction through advanced interface design will create effortless communication between people and driverless vehicles, enhancing comfort for people lacking technical skills.
AI-powered autonomous vehicles have the potential to revolutionize transportation, reducing accidents, improving traffic efficiency, and transforming mobility. However, safety and regulatory challenges remain significant hurdles to their widespread adoption. Stakeholders can create a safer and more reliable AV ecosystem by addressing AI perception limitations, ethical dilemmas, cybersecurity threats, and regulatory inconsistencies. The future of autonomous driving depends on collaboration between AI researchers, policymakers, and industry leaders to develop solutions that prioritize safety, transparency, and public trust. Only through these collective efforts can we pave the way for a future where AI-driven autonomous vehicles become a mainstream reality.
What’s Next for Autonomous Vehicles?
Talk to our experts about implementing compound AI systems in autonomous driving and how industries and departments leverage Agentic workflow and Decision Intelligence to become decision-centric. Harness AI to automate and optimize vehicle operations, enhancing efficiency, safety, and responsiveness.