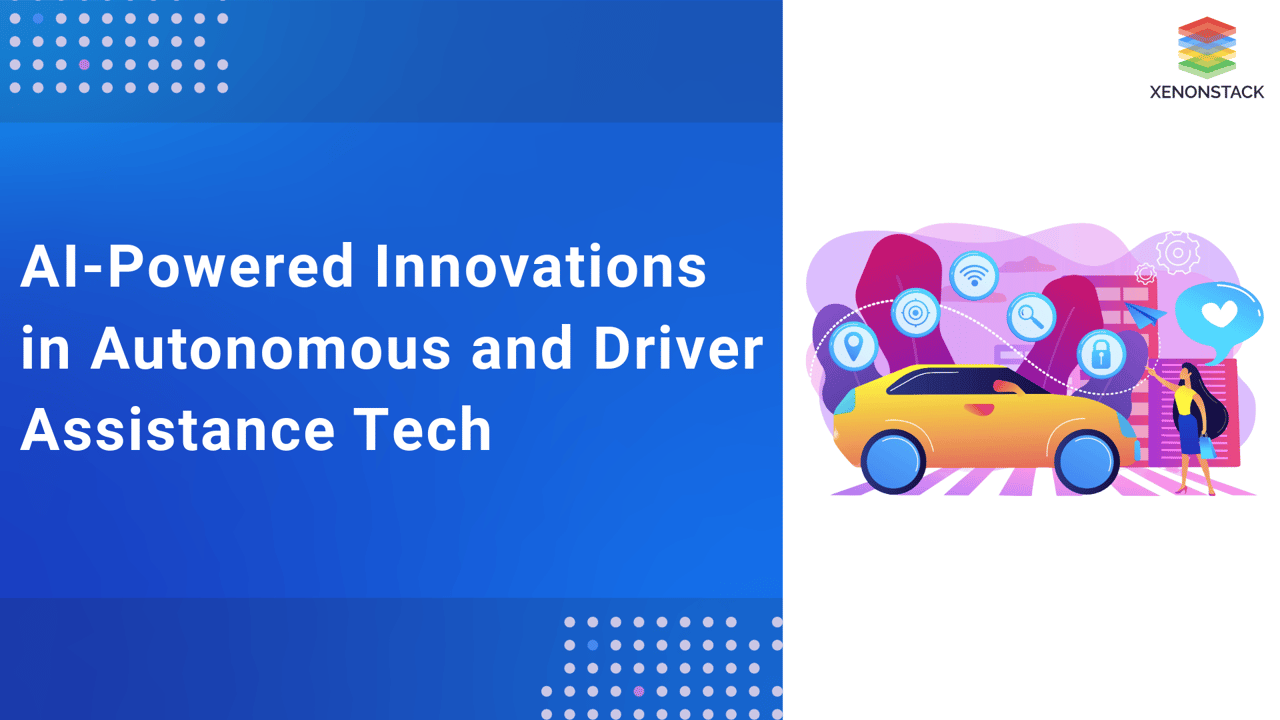
Artificial Intelligence (AI) is firmly placed at the cutting edge of the automotive industry’s development. It holds the key to a future with intelligent cars — both fully autonomous and smart assist systems. Here, we present the AI developments that underpin the self-driving revolution and advance the technology, bringing better and safer mobility. In this blog, we will consider the role of AI in the development of self-driving cars, current trends in innovations in driver safety systems, and the effects created by these innovations.
Functioning of AI in Self-Drive Cars
Self-driving cars, also known as autonomous mobiles, are based on using different or a group of artificial technologies like machine learning, computer vision, and deep learning to identify objects and select the appropriate paths. These can run independently in most cases due to embedded AI structures that mimic human sight, judgement, and decision-making capability.
AI enables AVs to:
-
Control its environment with mounted cameras, LiDAR, radar and ultrasonic sensors.
-
Analyze in real-time to determine the object's location, predict its movement, and assess the road surface conditions.
-
Make decisions using normative predictive algorithms to manage speed, steering, and braking.
It helps AI, the AV for autonomous driving, solve problems by copying the human mind's processes, enabling the vehicle to deal with dynamic and unpredictable circumstances.
The main AI technologies behind Self-Driving cars
Computer Vision
Computer vision enables the AV to understand signals it receives from the cameras and other sensors installed in the car. Because the objects and the model include pedestrians, vehicles, traffic signs, and lane markings, the AV can perceive instances and respond to them. YOLO, SSD, and Mask R-CNN, to name but a few, are used for precise real-time image segmentation and object detection.
Sensor Fusion
The contemplated self-driving cars use cameras, LiDAR (Light Detection and Ranging), radar, and ultrasonic sensor data to fuse them. This integrated data is then fed through the AI algorithms to generate a 3D map of the local environment, and the algorithm can still accurately map out the environment even in the presence of rain or fog AI-driven quality control.
Machine Learning and Deep Learning
These AI models are built from large data sets with which they learn to identify patterns and patterns, generate predictions and take actions.
For example:
-
In supervised learning, the vehicles can also detect objects, including but not limited to Pedestrians and road signs.
-
The training by reward offers the vehicle the ability to practice on simulations and enhance the capability to encounter other cars.
-
Neural networks work directly with big amounts of data from the vehicle’s sensors for immediate decision-making.
Path Planning and Control
Superimposing algorithms on the architectural designs of AVs allows them to determine the most efficient paths to drive on. Path planning systems determine courses, paths, and lanes, avoid collisions, and make acceptable lane changes. Unlike vehicle control systems, Artificial Intelligence makes driving as smooth as possible.
Efficient Natural Language Processing (NLP)
Natural language processing (NLP) is used in vehicles as voice recognition and as a virtual assistant. It has embedded applications in navigation, car settings modification, and even voice commands since it enhances user interfaces.
Levels of Autonomy
The Society of Automotive Engineers (SAE) categorizes autonomous vehicles into six levels (0-5) of autonomy:
-
Level 0: No use of automation; all worked by the driver.
-
Level 1: Primary help systems that are not dependent on sensors in the vehicle or environment (for example, ACC – adaptive cruise control).
-
Level 2: Partial automation steering with lane centring and parallel parking (driver needs to look at the road).
-
Level 3: Partial autonomy; the system performs some driving tasks while the others, requiring a human decision, are left to the driver.
-
Level 4: High automation; the vehicle's capability for manoeuvring through its environment without direct human control is applied in specific areas like urban areas.
-
Level 5: MPC and completely self-governing operation in any environment without a driver.
AI is now helping to move vehicles from Levels 1-2 (current commercial systems) to Levels 4-5.
AI in Driver Assistance Systems (ADAS)
ADAS is another term for a class of AI partial automation technologies that aim to augment the driver's safety and the ride's comfort. These systems are between conventional, manually operated cars and fully autonomous cars. AI powers ADAS features such as:
-
Adaptive Cruise Control (ACC)
Thus, AI optimizes the car's speed and distance from neighbouring vehicles to prevent drivers from getting tired during long drives.
-
Low-Speed Autonomous Emergency Braking (LS AEB)
It applies brake technology; by analyzing sensor data and utilizing artificial intelligence algorithms, it detects that a possible collision is impending, and the resultant action is to stop the vehicle.
-
Lane Departure Warning (LDW) and Lane Keeping Assist (LKA).
Other applications of AI technology include guiding drivers’ attention to road markings, alerting them, and correcting the car’s position if it moves out of the lane.
-
Blind Spot Detection
AID recognizes vehicles within blind zones and alerts drivers, reducing potential side impacts.
-
Traffic Sign Recognition
Some cameras include the AI camera, which can read traffic signs, and the information can be fed to the dashboard where the driver can see and adhere to the information given.
-
Driver Monitoring Systems
AI analyzes facial and eye movements to recognize the driver’s fatigue or distraction and sends notifications to reduce the risk of an accident.
-
Parking Assistance
Cameras and ultrasonic sensors help AI systems support drivers in parking tasks, particularly parallel and reverse parking.
Real-World Use Cases
AI in autonomous vehicles and ADAS is versatile across different industries, providing safety, efficiency, and increased convenience.
-
Ride-Hailing and Robo-Taxis
Waymo, Tesla, and Cruise are among some key players that are integrating AI into self-driving ride-hailing. Self-driving cars eliminate traffic jams and manage well in cities.
-
Logistics and Freight Transport
Trucks controlled by artificial intelligence are recasting the supply chain industry. However, the major use of AI in the field is seen in companies such as TuSimple and Embark for self-driving trucks, resulting in faster delivery, fewer expenses, and the problem of scarcity of drivers.
-
Public Transportation
Self-driving cars for public transit are becoming popular, and artificial intelligence is being piloted in many cities across the globe. They provide evidence of safer, environmentally friendly, and improved efficient public transportation in urban areas.
-
Improved Road Safety
ADAS, backed by AI, greatly reduces road accidents since it helps drivers be attentive and promptly respond to any danger on the road. For instance, Volvo’s driver assistance systems are recognized as safe.
-
Smart Cities
Self-driving cars are critical to smart cities because they depend on artificial intelligence and intelligent systems—traffic signals and sensors—to optimize vehicle flow.
-
Emergency Services
For instance, self-driving cars can be deployed in ambulances to navigate traffic-congested roads and reach their destinations independently.
-
Conventional Construction and Mining Vehicles
AI makes self-propelled equipment work independently in dangerous areas like construction zones and mines, which helps reduce the rate of accidents.
New Trends of AI Applied to Autonomous Systems
5G Connectivity
Integration with 5G networks improves the ability of AI cars to process Data in real-time. 5G technology provides reliable communication with low latency between vehicles and infrastructure to support proper decision-making and safety.
Edge Computing
AI models are now implemented inside the vehicle through Edge Computing that avoids using cloud resources. Edge computing makes a response faster, and that response is crucial in the case of self-driving vehicles.
AI-Powered Simulations
Managers apply AI simulations to self-governing systems in experimental environments for developmental and educational purposes. This helps to progress the state of the art in AI quickly and safely, in that the models are reproduced more rapidly and to a higher standard than was possible before they were established.
New generation LiDAR and Camera
LiDAR has progressed in recent years, and the high-definition cameras have enhanced object recognition in perception and helped construct a better environment map.
AI-Powered HD Maps
HD maps offer resolution details of the road network combined with AI to provide geometries, traffic regulations and infrastructures for effective navigation for self-driving cars.
AI Barriers to Self-Driving Cars
While AI is transformative, there are still challenges to address:
-
Regulatory Hurdles: The self-driving cars will need to meet different regional standards.
-
Ethical Dilemmas: Evaluating a risk to take or avoid in this and other similar circumstances always poses an ethical dilemma.
-
Safety and Security: The main issues are cybersecurity and AI model quality.
-
Public Acceptance: Consumer trust is the key to user adoption of a social commerce platform.
Robotics undeniably disrupt the on-road automotive industry; self-driven cars and smart or assisted driving are already making driving safer, faster and more comfortable. From autonomous vehicles to intelligent safety assist systems, AI is revolutionizing the future of mobility. 5G is also still emerging and improving, as are edge computing and sensor techs, and as all these technologies proceed forward, fully autonomous driving is advancing with it. Fraud Prediction Using AutoAI.
AI has a bright future for all transport solutions by enhancing smarter, safer, and more connected vehicles. It will also ensure that AI continues to evolve continuously, which will help set the stage where robot-based systems always change the course of various industries and people’s lives.
Next Steps with Autonomous Vehicles
Consult our experts about implementing advanced AI systems and how industries leverage Decision Intelligence to enhance autonomous vehicles and driver assistance systems. Utilize AI to automate and optimize vehicle operations, improving efficiency, responsiveness, and safety.