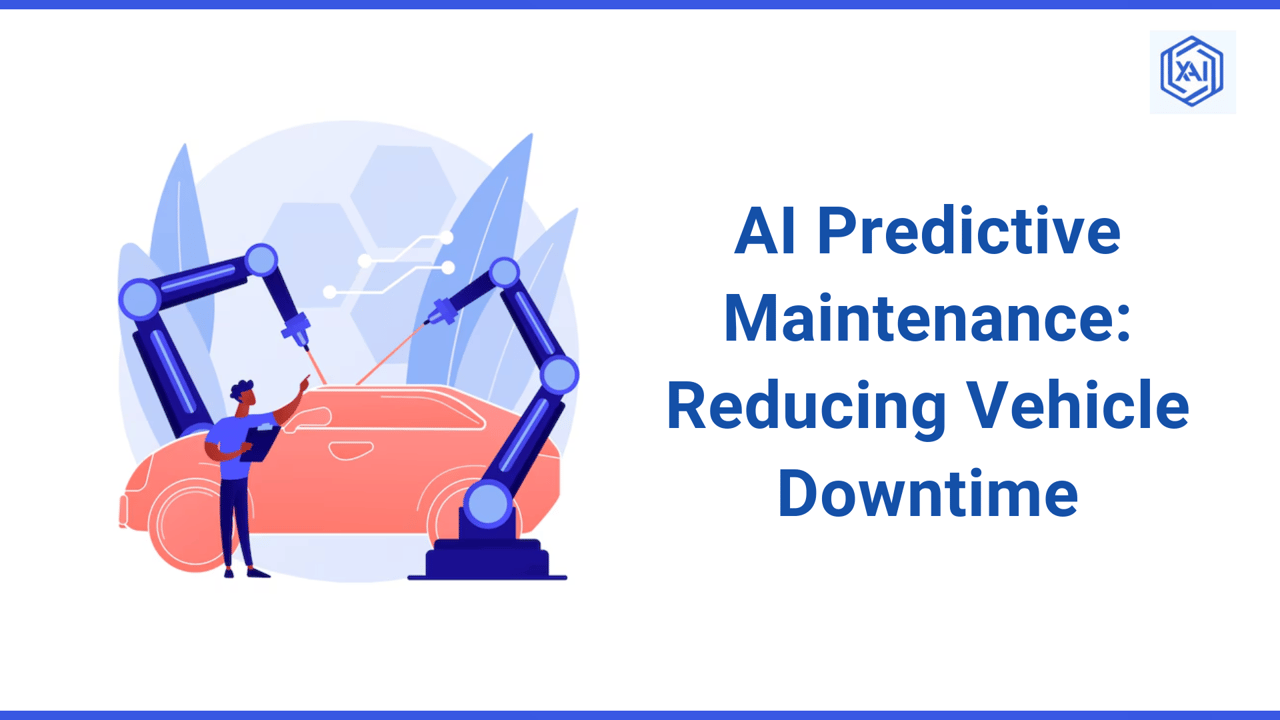
The automotive industry is experiencing rapid growth in the predictive maintenance market, which is expected to reach $16.75 billion by 2030, according to a recent study. This growth is fueled by the integration of artificial intelligence (AI) and IoT technologies that are revolutionizing vehicle maintenance. Leading cloud platforms like Azure AI services, Google AI Services, and AWS AI services are driving the adoption of AI-powered solutions, enabling vehicle owners and fleet managers to take a data-driven approach to maintenance.
AI-driven predictive maintenance is one of the most significant advancements, enabling the early detection of potential vehicle failures before they occur. Unlike traditional maintenance models, which rely on reactive or scheduled servicing, AI-powered solutions offer data-driven insights to predict component failures, leading to reduced downtime, improved safety, and enhanced operational efficiency.
Why Traditional Maintenance Methods Fall Short
Traditional vehicle maintenance models rely on either reactive maintenance (fixing problems after they happen) or scheduled maintenance (periodic servicing regardless of the vehicle’s actual condition). Both methods are inefficient in different ways, leading to increased costs, unexpected breakdowns, and reduced vehicle lifespan.
Predictive maintenance for vehicles powered by AI changes this paradigm by using data-driven insights to predict component failures, allowing vehicle owners, fleet managers, and manufacturers to address issues before they escalate. This shift from reactive to proactive maintenance results in lower costs, enhanced safety, and improved operational efficiency.
What is AI-powered Predictive Maintenance?
Predictive maintenance (PdM) is a technology-driven approach that uses artificial intelligence (AI), machine learning (ML), and Internet of Things (IoT) sensors to monitor a vehicle’s condition in real-time. Unlike traditional maintenance methods that rely on fixed service schedules or react to breakdowns after they occur, PdM continuously gathers data from key vehicle components like the engine, transmission, brakes, and battery.
AI-powered systems then analyze this data to detect early warning signs of potential failures. By identifying small irregularities, such as unusual vibrations, temperature fluctuations, or declining battery efficiency, predictive maintenance enables timely intervention before a problem escalates into a costly repair or a complete breakdown.
How Predictive Maintenance Works
-
Data Collection: IoT sensors installed in vehicles capture real-time data on various performance metrics.
-
AI & Machine Learning Analysis: Advanced algorithms process the collected data to identify patterns and anomalies.
-
Failure Prediction: AI models assess the likelihood of component failures based on historical and real-time data.
-
Timely Alerts & Recommendations: The system notifies vehicle owners or fleet managers about necessary maintenance actions.
-
Continuous Optimization: AI improves over time by learning from past data, making predictions more accurate.
Traditional vs. Predictive Maintenance Approaches
Maintenance Type |
Description |
Challenges |
Reactive Maintenance |
Repairs occur only after a failure happens. |
High repair costs, unexpected downtime, and potential safety risks. |
Scheduled Maintenance |
Vehicles are serviced at fixed intervals (e.g., every 10,000 miles). |
This may lead to unnecessary maintenance or failures between scheduled services. |
Predictive Maintenance (AI-based) |
Uses real-time data and AI models to predict failures before they happen. |
Requires data collection, advanced analytics, and investment in AI technology. |
Cutting-Edge Technologies Driving AI in Predictive Maintenance
-
Machine Learning & AI Algorithms: These technologies analyze vehicle performance data to detect patterns and identify anomalies.
-
IoT Sensors: These sensors collect real-time data from critical components, including the engine, brakes, and transmission.
-
Cloud Computing: This infrastructure enables large-scale data storage and high-performance processing.
-
Edge Computing: This approach allows real-time decision-making directly within the vehicle, enhancing responsiveness and efficiency.
The Role of AI in Enhancing Vehicle Predictive Maintenance Accuracy
Machine Learning & Predictive Analytics
AI-powered predictive maintenance relies on machine learning (ML) algorithms that process vast amounts of historical and real-time vehicle data. These models identify patterns indicating potential failures, such as irregular engine vibrations, excessive heat generation, or fluctuating battery voltage.
For example, if an ML model detects an unusual increase in engine temperature and vibration, it can predict that a cooling system failure might occur within the next few weeks, prompting timely maintenance.
IoT & Sensors for Real-Time Data Collection
Modern vehicles are equipped with hundreds of IoT sensors that continuously monitor critical performance parameters. These sensors provide real-time data, helping AI systems assess a vehicle’s condition and detect potential issues before they escalate. Key parameters tracked include:
-
Engine Temperature: Identifies overheating issues and potential cooling system failures.
-
Tire Pressure: Monitors inflation levels to improve fuel efficiency and prevent blowouts.
-
Fuel Efficiency: Tracks consumption patterns to detect inefficiencies or fuel system problems.
-
Brake Wear: Measures brake pad degradation and responsiveness for optimal safety.
-
Battery Health: Analyzes voltage levels and charging patterns to predict potential failures.
Big Data & Cloud Computing
Predictive maintenance relies on large-scale data processing, requiring cloud computing to handle vast amounts of real-time diagnostic data from thousands of vehicles. Cloud platforms enable:
-
Centralized Data Storage: Securely stores diagnostic data for long-term trend analysis.
-
Advanced Analytics & AI Processing: Uses machine learning models to detect patterns in vehicle health.
-
Remote Monitoring & Updates: Allows manufacturers and fleet managers to monitor vehicles from anywhere.
-
Seamless Data Sharing: Enables collaboration between service providers, automakers, and fleet operators.
Edge AI for Onboard Diagnostics
Unlike cloud-based AI, Edge AI processes data directly on a vehicle’s onboard computer, enabling real-time diagnostics without requiring constant internet connectivity. This approach is crucial for immediate decision-making in critical situations. Key benefits include:
-
Instant Failure Detection: Alerts drivers about imminent issues, such as brake failures or engine malfunctions.
-
Reduced Latency: Processes data locally for real-time responsiveness.
-
Enhanced Security & Privacy: Keeps sensitive vehicle data within the onboard system.
-
Reliable Operation in Remote Areas: Functions even in locations with limited or no internet access.
Benefits of AI in Enhancing Vehicle Predictive Maintenance
-
Cost Savings: AI-driven predictive maintenance identifies issues early, reducing unexpected repair costs, preventing further damage, and minimizing emergency repairs and warranty claims.
-
Increased Vehicle Lifespan: Regular AI diagnostics proactively address issues, minimizing wear on key components, ensuring optimal performance, and extending vehicle lifespan.
-
Enhanced Safety: AI detects potential failures in critical safety systems like brakes and steering, preventing accidents and ensuring vehicle reliability.
-
Operational Efficiency for Fleets: AI predicts vehicle servicing needs and forecasts fuel efficiency trends, optimizing maintenance schedules and improving route planning to reduce downtime and costs.
-
Sustainability & Environmental Impact: By preventing breakdowns and detecting engine inefficiencies, AI reduces fuel wastage, emissions, and the environmental impact of vehicle fleets.
-
Improved Decision-Making and Planning: AI analyzes data in real-time, enabling informed decision-making for maintenance, repairs, and vehicle management, resulting in improved efficiency.
Traditional Maintenance vs. AI-Driven Predictive Maintenance
Factor |
Traditional Maintenance |
AI-Powered Predictive Maintenance |
Efficiency |
Periodic, can be unnecessary or late. |
Data-driven, optimizes maintenance cycles. |
Cost |
Higher due to unexpected repairs. |
Lower due to proactive intervention. |
Downtime |
Unplanned breakdowns disrupt operations. |
Minimizes vehicle downtime. |
How Leading Automotive Companies Use AI for Predictive Maintenance
Tesla: AI-Driven Predictive Maintenance & OTA Updates
Tesla employs AI to monitor critical vehicle components such as battery health, motor performance, and braking systems in real-time. This proactive approach enables early detection of potential issues, allowing for timely interventions that enhance vehicle reliability and customer satisfaction. Additionally, Tesla's over-the-air (OTA) updates facilitate remote software fixes, reducing the need for physical service center visits and improving operational efficiency.
General Motors (OnStar Diagnostics)
General Motors' OnStar system offers real-time vehicle diagnostics and predictive maintenance alerts, assisting owners in preventing costly breakdowns. By providing easy-to-read monthly diagnostics reports and proactive alerts, OnStar helps maintain vehicle health and ensures a safer driving experience.
BMW & Daimler (Mercedes-Benz Uptime)
Mercedes-Benz Uptime utilizes AI to monitor truck components, delivering early warnings about potential failures and reducing downtime for logistics companies. This system enhances fleet management by ensuring vehicles are serviced only when necessary, optimizing maintenance schedules, and improving overall operational efficiency.
Uber & Lyft: AI for Ride-Sharing Fleet Optimization
Ride-sharing companies like Uber and Lyft employ AI-based maintenance prediction to keep their vehicles in optimal condition, minimizing service disruptions. By analyzing real-time data, these platforms can predict maintenance needs, schedule timely interventions, and ensure a reliable service for users.
AI in Trucking & Logistics: Preventing Costly Downtime
Companies such as Daimler Trucks, Volvo, and FedEx use AI-driven maintenance analytics to reduce fleet downtime and enhance delivery efficiency. By predicting component failures and scheduling maintenance proactively, these companies ensure their fleets operate smoothly, leading to improved service reliability and customer satisfaction.
Industry Statistics on AI in Predictive Maintenance
-
McKinsey estimates that AI-driven predictive maintenance could reduce maintenance costs by 10-40% and cut downtime by up to 50%.
-
IBM predicts that AI-driven fleet management can improve fuel efficiency by 15-20%.
-
Deloitte reports that predictive maintenance in the trucking industry can increase fleet availability by 20-25%.
Future Trends in AI-Powered Predictive Maintenance
Self-Learning AI Models: AI systems will evolve into self-learning models that continuously improve their predictive abilities, analyzing more data to identify potential issues earlier and optimize maintenance schedules. AI in Autonomous Vehicles: As autonomous vehicles become more common, AI will enable self-maintenance by performing diagnostics, predicting failures, and autonomously initiating repairs, reducing human intervention and improving fleet management. Integration with Electric Vehicles (EVs): AI will monitor EV battery health and optimize charging cycles, extending battery life and predicting potential issues before they cause downtime. AI-Driven Smart Fleet Management: AI will integrate predictive maintenance with smart fleet management systems, optimizing routes, forecasting fuel consumption, and providing real-time maintenance alerts to improve operational efficiency for vehicle owners and fleet operators.
Challenges in Full Adoption of AI Predictive Maintenance in Vehicles
-
Data Privacy & Security: With vehicles collecting vast amounts of data, ensuring the security of vehicle diagnostics is crucial. Protecting sensitive information from cyber threats and unauthorized access is essential to maintain user trust and privacy.
-
Compliance with Regulations: Companies must adhere to data regulations like GDPR and CCPA, requiring secure data storage and transparent data-sharing practices to meet compliance and mitigate legal risks.
-
Data Quality & Integration: For AI to effectively predict maintenance needs, consistent, high-quality data from various vehicle components is required. Ensuring data accuracy and smooth integration from diverse sensors poses a technical challenge.
-
Cost of Implementation: The initial cost of implementing AI-based predictive maintenance, including hardware, software, and training, can be a barrier for smaller businesses. Addressing these financial challenges will be key to broader adoption.
Why AI-powered Predictive Maintenance is the Future of Vehicle Care
AI-powered predictive maintenance is transforming the automotive industry, making vehicle management more cost-effective, efficient, and safe. By using machine learning, IoT sensors, and big data, companies can predict failures before they happen, ultimately improving vehicle performance and reducing operational costs.
As more automakers and fleet operators adopt AI-driven maintenance solutions, the future of vehicle management will shift towards a data-driven, proactive model, reducing breakdowns, enhancing safety, and ensuring long-term sustainability.
The road ahead is clear—AI is the future of vehicle maintenance.
Next Steps in Adopting AI-Powered Predictive Maintenance
Talk to our experts about implementing AI-powered predictive maintenance systems, and how industries and different departments use machine learning and IoT sensors to predict vehicle failures. Leverage AI to optimize maintenance processes and improve operational efficiency, ensuring proactive decision-making and minimizing unexpected downtime.